The "Data Analysis Project" course empowers students to apply their knowledge and skills gained in this specialization to conduct a real-life data analysis project of their interest. Participants will explore various directions in data analysis, including supervised and unsupervised learning, regression, clustering, dimension reduction, association rules, and outlier detection. Throughout the modules, students will learn essential data analysis techniques and methodologies and embark on a journey from raw data to knowledge and intelligence. By completing the course, students will be proficient in data analysis, capable of applying their expertise in diverse projects and making data-driven decisions.

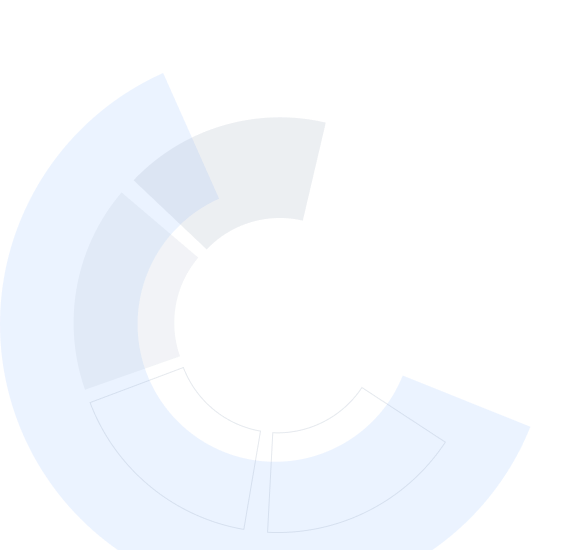
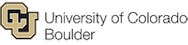
Data Analysis with Python Project
This course is part of Data Analysis with Python Specialization

Instructor: Di Wu
Sponsored by IEM UEM Group
Recommended experience
Recommended experience
What you'll learn
Define the scope and direction of a data analysis project, identifying appropriate techniques and methodologies for achieving project objectives.
Apply various classification and regression algorithms and implement cross-validation and ensemble techniques to enhance the performance of models.
Apply various clustering, dimension reduction association rule mining, and outlier detection algorithms for unsupervised learning models.
Details to know

Add to your LinkedIn profile
1 assignment
See how employees at top companies are mastering in-demand skills
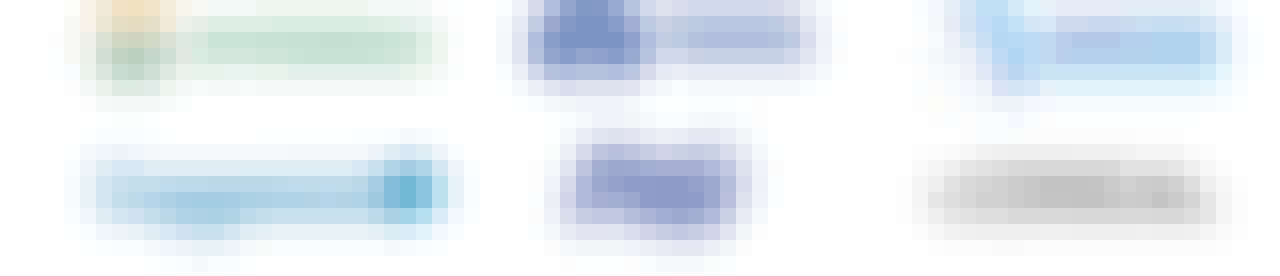
Build your subject-matter expertise
- Learn new concepts from industry experts
- Gain a foundational understanding of a subject or tool
- Develop job-relevant skills with hands-on projects
- Earn a shareable career certificate
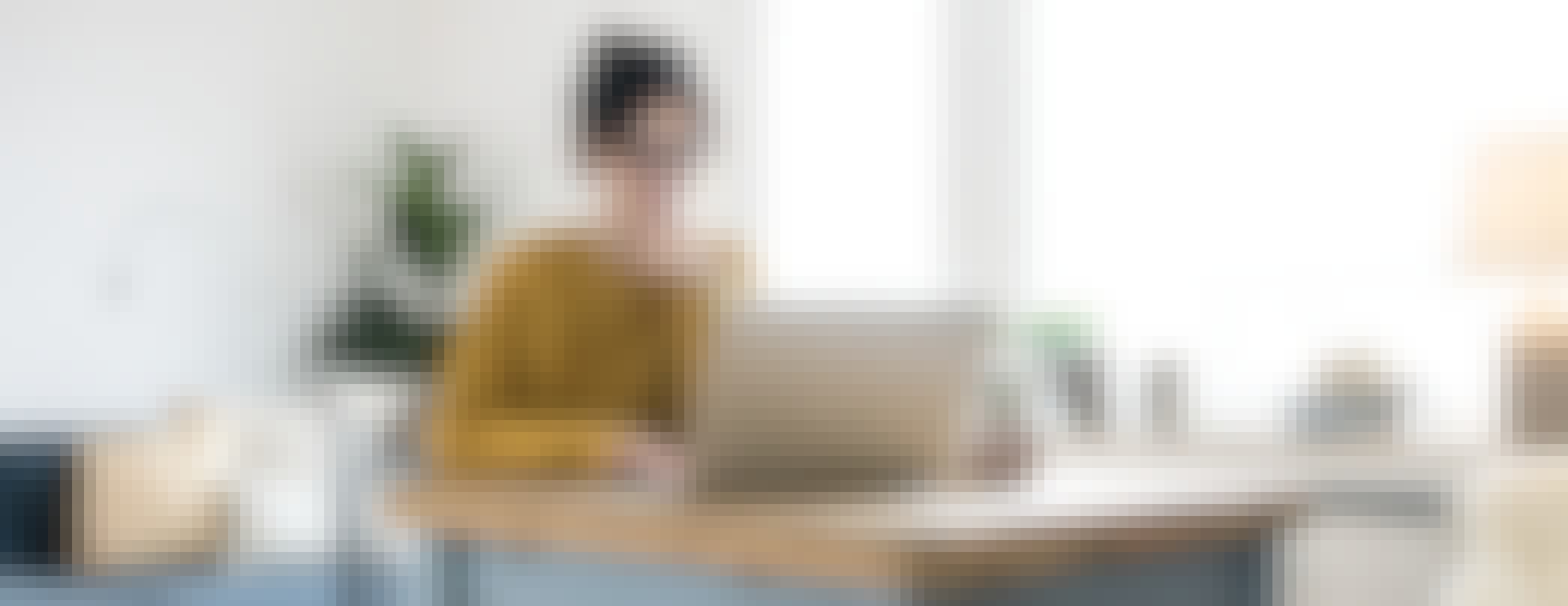
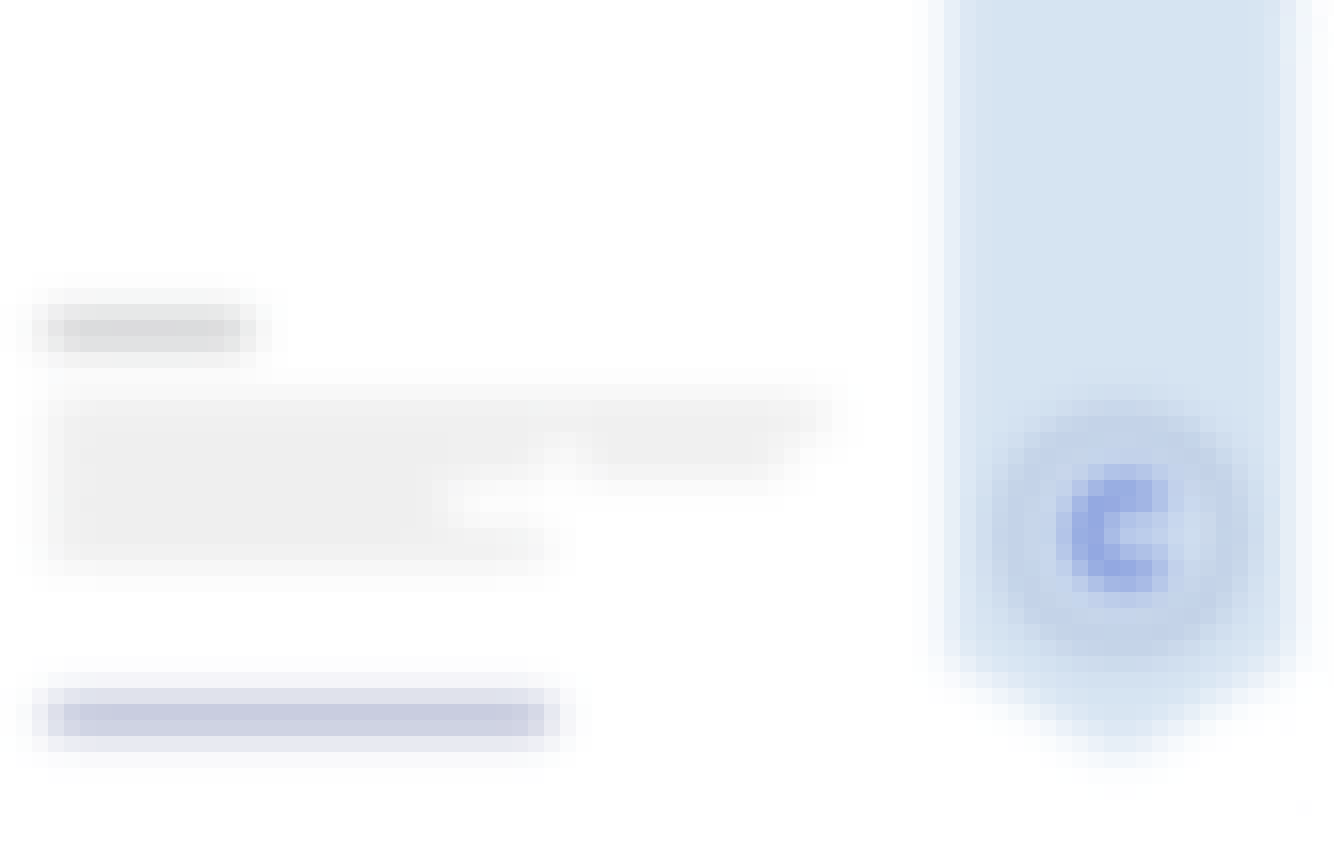
Earn a career certificate
Add this credential to your LinkedIn profile, resume, or CV
Share it on social media and in your performance review
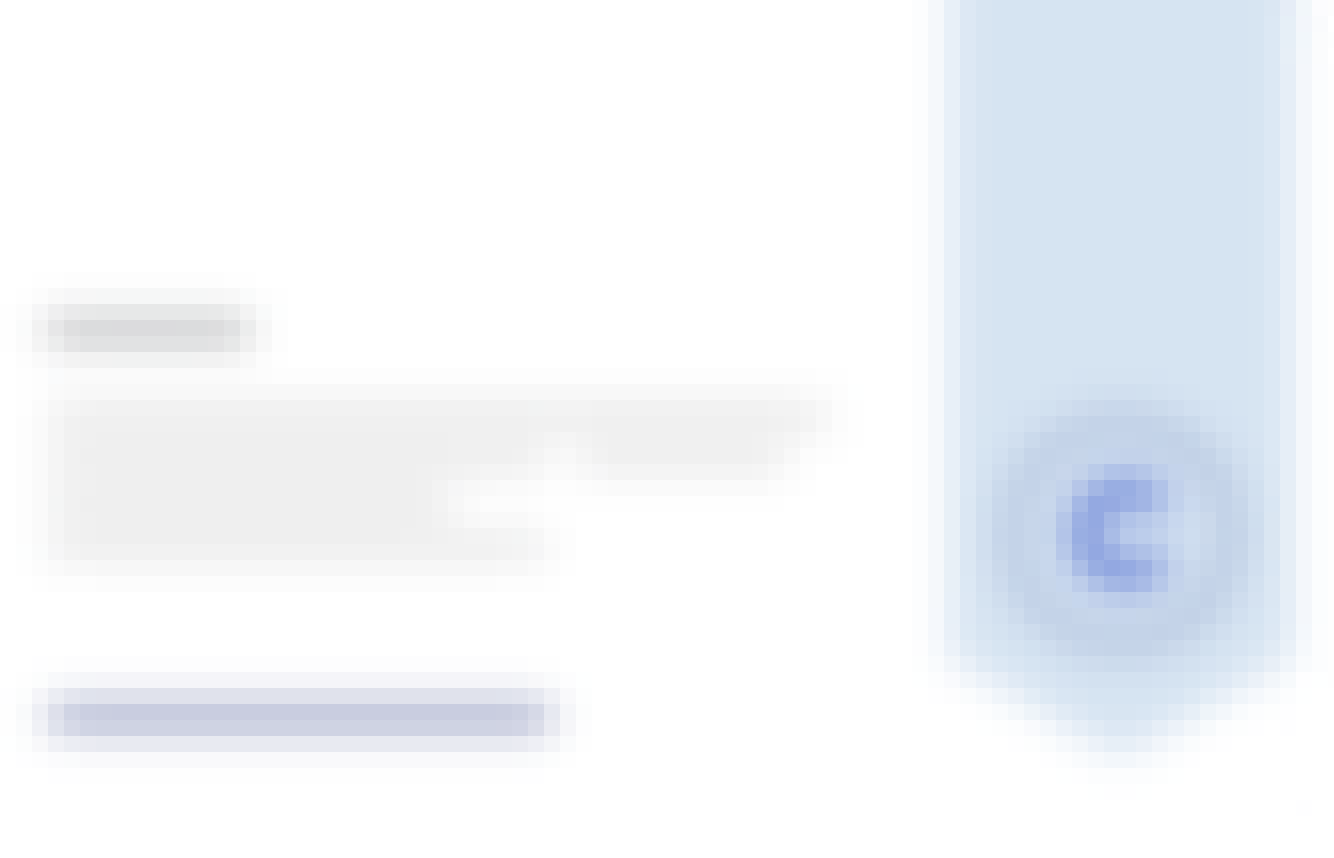
There are 7 modules in this course
In this first week, you will gain an overview of data analysis, understanding supervised and unsupervised learning directions. You will learn how to define the scope and direction of their data analysis project effectively.
What's included
1 reading
This week focuses on classification techniques, where you will explore Nearest Neighbors, Decision Trees, SVM, Naive Bayes, Logistic Regression, cross-validation, ensemble methods, and evaluation metrics.
What's included
1 reading
This week you will delve into regression techniques, including Simple Linear, Polynomial Linear, Linear with regularization, multivariate regression, cross-validation, ensemble methods, and evaluation metrics.
What's included
1 reading
This week introduces clustering techniques, including partitioning, hierarchical, density-based, and grid-based methods, for unsupervised pattern discovery.
What's included
1 reading
This week will focus on dimension reduction techniques, with a particular emphasis on Principal Component Analysis (PCA).
What's included
1 reading
This week focuses on a comprehensive case study where you will apply association rule mining and outlier detection techniques to solve a real-world problem.
What's included
1 reading
This final week focuses on outlier detection methods, including Zscore, IQR, OneClassSVM, Isolation Forest, DBSCAN, LOF, and contextual outliers.
What's included
2 readings1 assignment1 discussion prompt
Instructor

Offered by
Why people choose Coursera for their career




Recommended if you're interested in Data Science
University of Michigan
University of Maryland, College Park
University of Michigan
Coursera Instructor Network

Open new doors with Coursera Plus
Unlimited access to 10,000+ world-class courses, hands-on projects, and job-ready certificate programs - all included in your subscription
Advance your career with an online degree
Earn a degree from world-class universities - 100% online
Join over 3,400 global companies that choose Coursera for Business
Upskill your employees to excel in the digital economy