This course introduces students to data and statistics. By the end of the course, students should be able to interpret descriptive statistics, causal analyses and visualizations to draw meaningful insights.

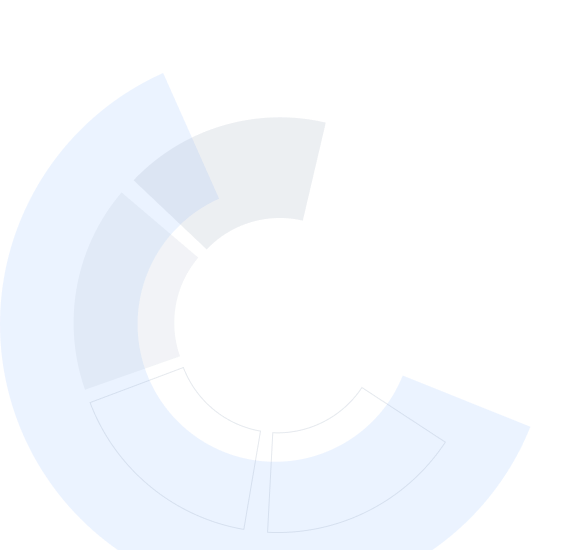
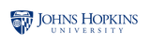
Data – What It Is, What We Can Do With It
This course is part of Data Literacy Specialization

Instructor: Jennifer Bachner, PhD
Sponsored by IEM UEM Group
9,306 already enrolled
(167 reviews)
Recommended experience
Skills you'll gain
- Mathematics and Mathematical Modeling
- Exploratory Data Analysis
- Probability & Statistics
- Research Methodologies
- Descriptive Statistics
- Probability
- Research
- Statistical Inference
- Statistical Methods
- Applied Mathematics
- Data Science
- Scientific Methods
- Analytics
- Statistics
- Experimentation
- Data Analysis
- General Science and Research
- Statistical Analysis
- Data Visualization
- Research Design
Details to know

Add to your LinkedIn profile
13 assignments
See how employees at top companies are mastering in-demand skills
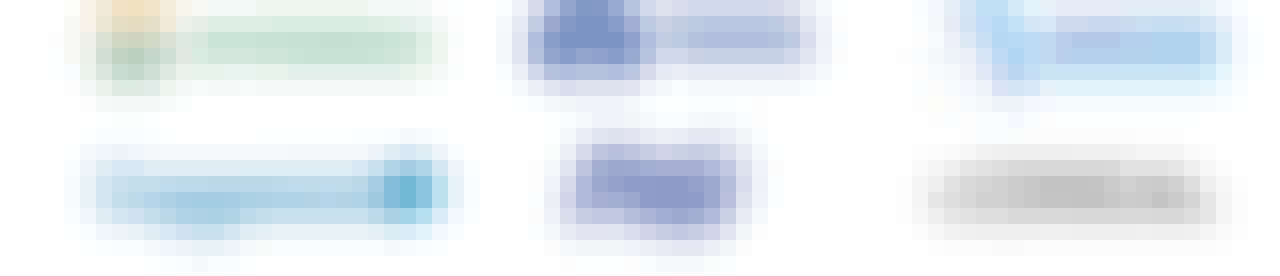
Build your subject-matter expertise
- Learn new concepts from industry experts
- Gain a foundational understanding of a subject or tool
- Develop job-relevant skills with hands-on projects
- Earn a shareable career certificate
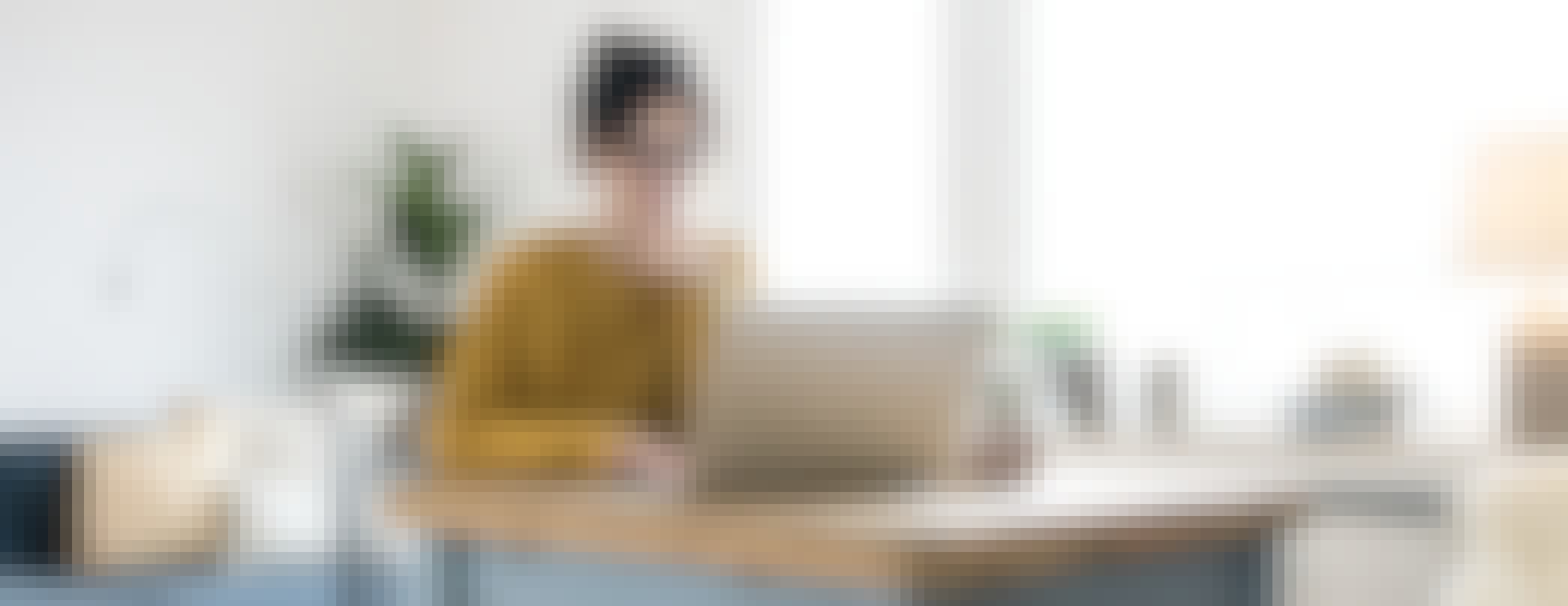
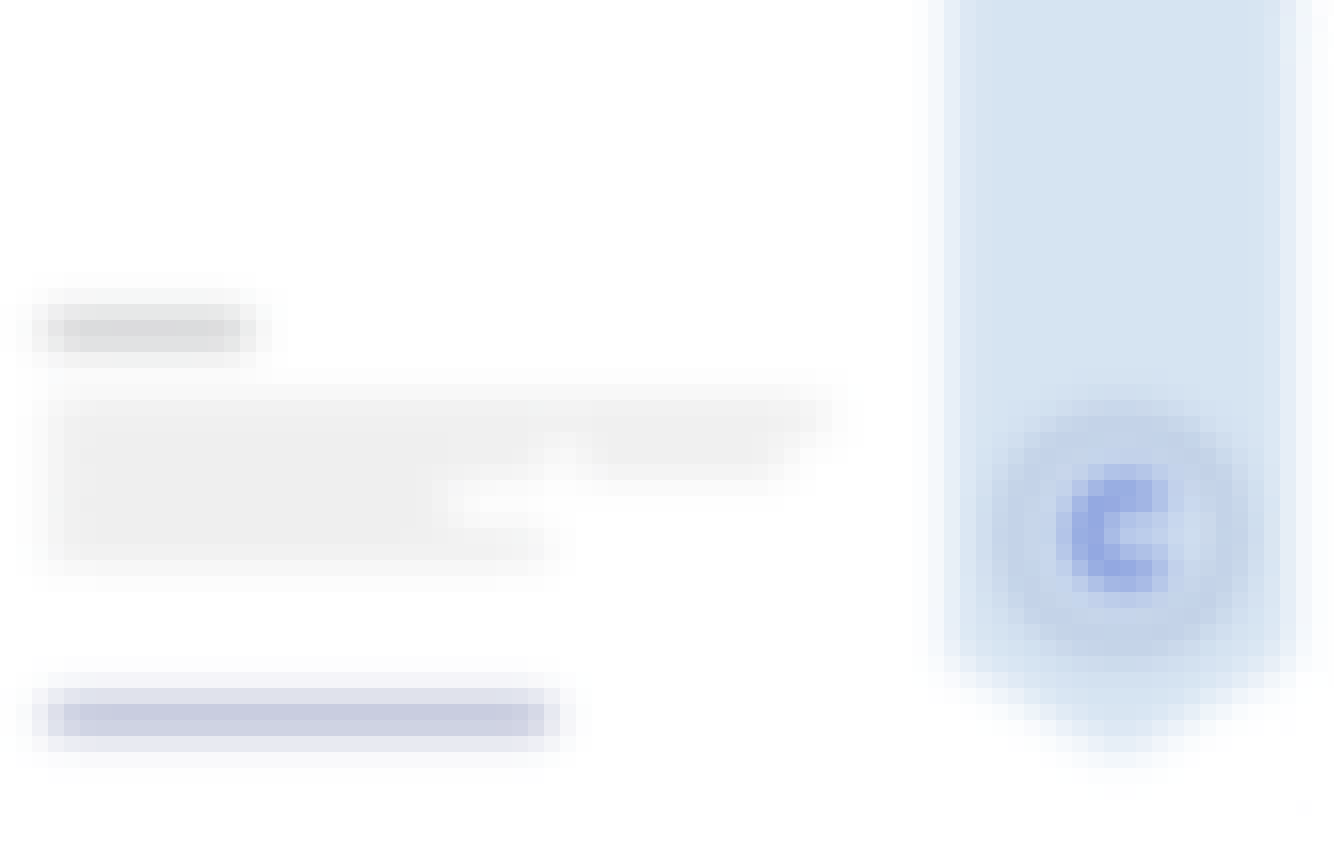
Earn a career certificate
Add this credential to your LinkedIn profile, resume, or CV
Share it on social media and in your performance review
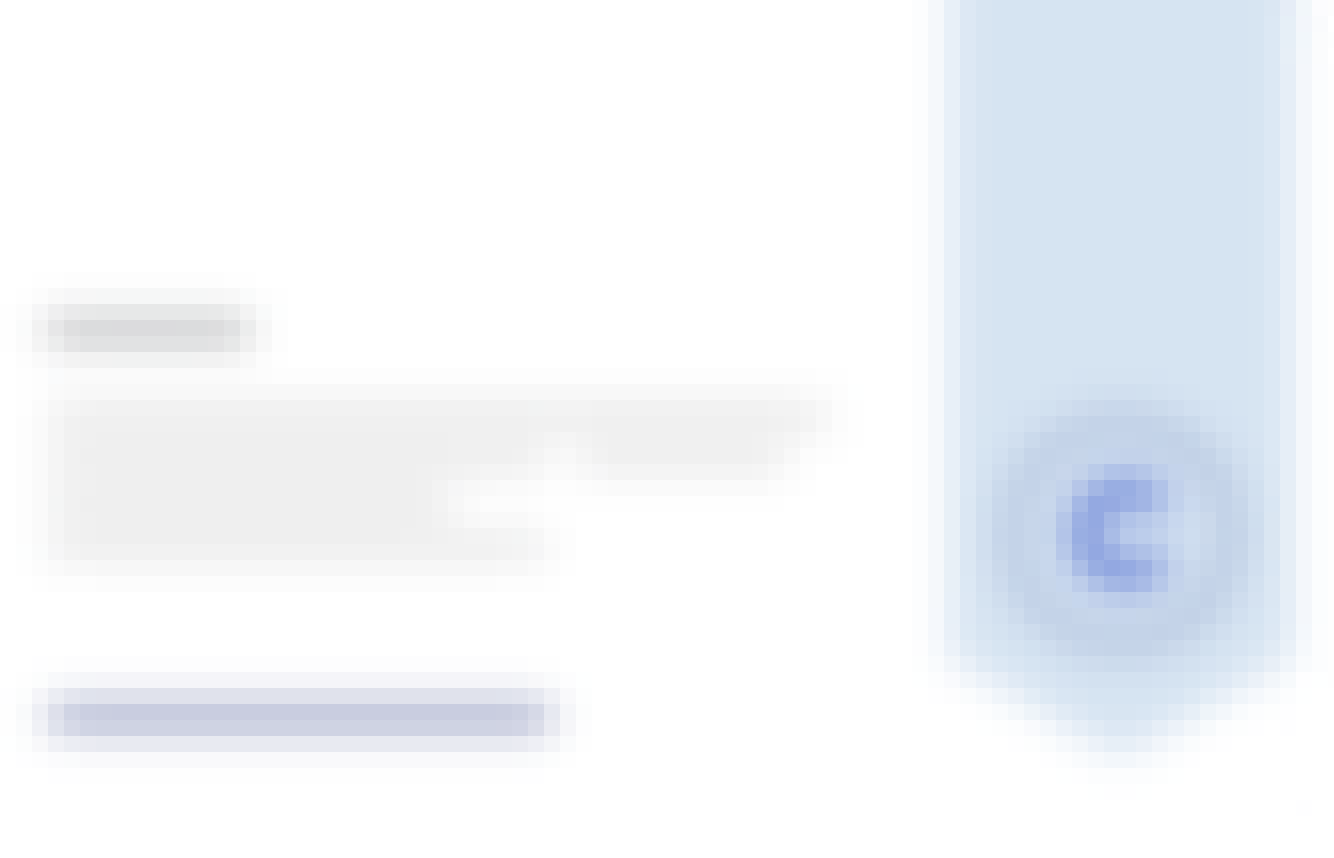
There are 4 modules in this course
When most people think about using data, they quickly jump to considering the best way to analyze it with statistical methods. A good analysis, however, begins with a strong theoretical framework. A good theory will guide the collection of data, selection of appropriate statistical methods and interpretation of the results. Further, the theory will determine what kind of research design is needed, such as an observational study or experiment. This module will focus on the development of high-quality theories that can be used to guide descriptive, causal and predictive inference.
What's included
4 videos3 readings1 assignment1 discussion prompt
Establishing causality is frequently the primary motivation for research. Policymakers often want to understand how the implementation of a new program or other policy tool will affect an outcome of interest. Will smaller class sizes increase student learning? Will the implementation of stricter background checks for gun buyers reduce gun violence? Biomedical researchers often want to understand whether a new medicine will improve a disease outcome. Will taking a drug improve life expectancy, or even cure the disease under study? To answer these and similar questions, analysts must develop research designs that are appropriate for causal inference. Estimating a causal effect is challenging, yet it is essential to understand the impacts of a policy, medicine or any other kind of intervention.
What's included
4 videos3 readings4 assignments
Over the next four lessons we'll begin to make sense of raw data. Staring at raw data, such as a spreadsheet, does not reveal much of anything about the key takeaway points. Consider a variable such as a survey question that asks about the level of discrimination in the U.S. (where the answer choices are "a lot," "some," "only a little," "none at all," and "don't know"). Reading the raw data does not tell you about the average respondent or the distribution of responses among the possible answer choices. To better understand the shape of the distribution, we can calculate measures of central tendency, measures of spread and characterize the data's dispersion. These summary statistics allow a researcher to draw some simple yet powerful initial conclusions about what the data tell us in a real-world sense.
What's included
4 videos5 readings4 assignments
Edward Tufte, a world-renowned expert of data visualization, once said, "There is no such thing as information overload. There is only bad design." When communicating the results of an analysis, and particularly when trying to persuade an audience, a picture is truly worth a thousand words. A well-designed graph can leverage either a small or large amount of data to make a convincing argument. Data visualizations highlight specific points about the underlying information and enable the viewer to draw insights that are nearly invisible when staring at the numbers alone. In short, to be a good at communicating with data, you must become skilled at visualizing data.
What's included
3 videos4 readings4 assignments
Instructor

Offered by
Why people choose Coursera for their career




Learner reviews
167 reviews
- 5 stars
70.65%
- 4 stars
22.75%
- 3 stars
4.19%
- 2 stars
1.19%
- 1 star
1.19%
Showing 3 of 167
Reviewed on Jan 18, 2022
Some of the quiz questions feel a bit unfair. Answers that are "Accurate, but..." do not feel appropriate for these kinds of lessons.
Reviewed on Jul 29, 2023
it was very helpful as it provided me with general idea on how to analyze and interpret data
Reviewed on Jul 9, 2023
Very nice and clearly explained the topics with practical examples.
Recommended if you're interested in Data Science
University of Colorado System
Google

Open new doors with Coursera Plus
Unlimited access to 10,000+ world-class courses, hands-on projects, and job-ready certificate programs - all included in your subscription
Advance your career with an online degree
Earn a degree from world-class universities - 100% online
Join over 3,400 global companies that choose Coursera for Business
Upskill your employees to excel in the digital economy