This course will help prepare students for developing code that can process large amounts of data in parallel on Graphics Processing Units (GPUs). It will learn on how to implement software that can solve complex problems with the leading consumer to enterprise-grade GPUs available using Nvidia CUDA. They will focus on the hardware and software capabilities, including the use of 100s to 1000s of threads and various forms of memory.

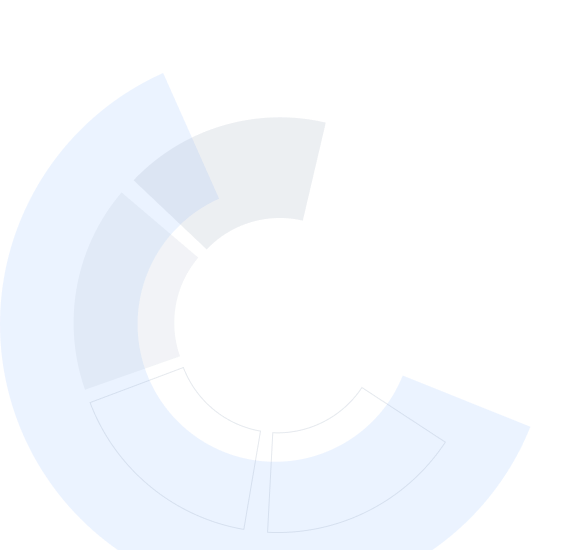
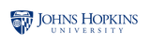
Introduction to Parallel Programming with CUDA
This course is part of GPU Programming Specialization

Instructor: Chancellor Thomas Pascale
Sponsored by IEM UEM Group
6,663 already enrolled
Recommended experience
Recommended experience
What you'll learn
Students will learn how to utilize the CUDA framework to write C/C++ software that runs on CPUs and Nvidia GPUs.
Students will transform sequential CPU algorithms and programs into CUDA kernels that execute 100s to 1000s of times simultaneously on GPU hardware.
Skills you'll gain
Details to know

Add to your LinkedIn profile
5 assignments
See how employees at top companies are mastering in-demand skills
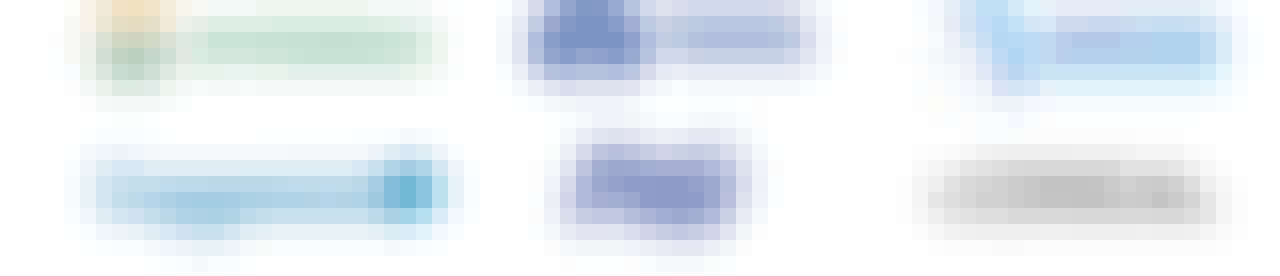
Build your subject-matter expertise
- Learn new concepts from industry experts
- Gain a foundational understanding of a subject or tool
- Develop job-relevant skills with hands-on projects
- Earn a shareable career certificate
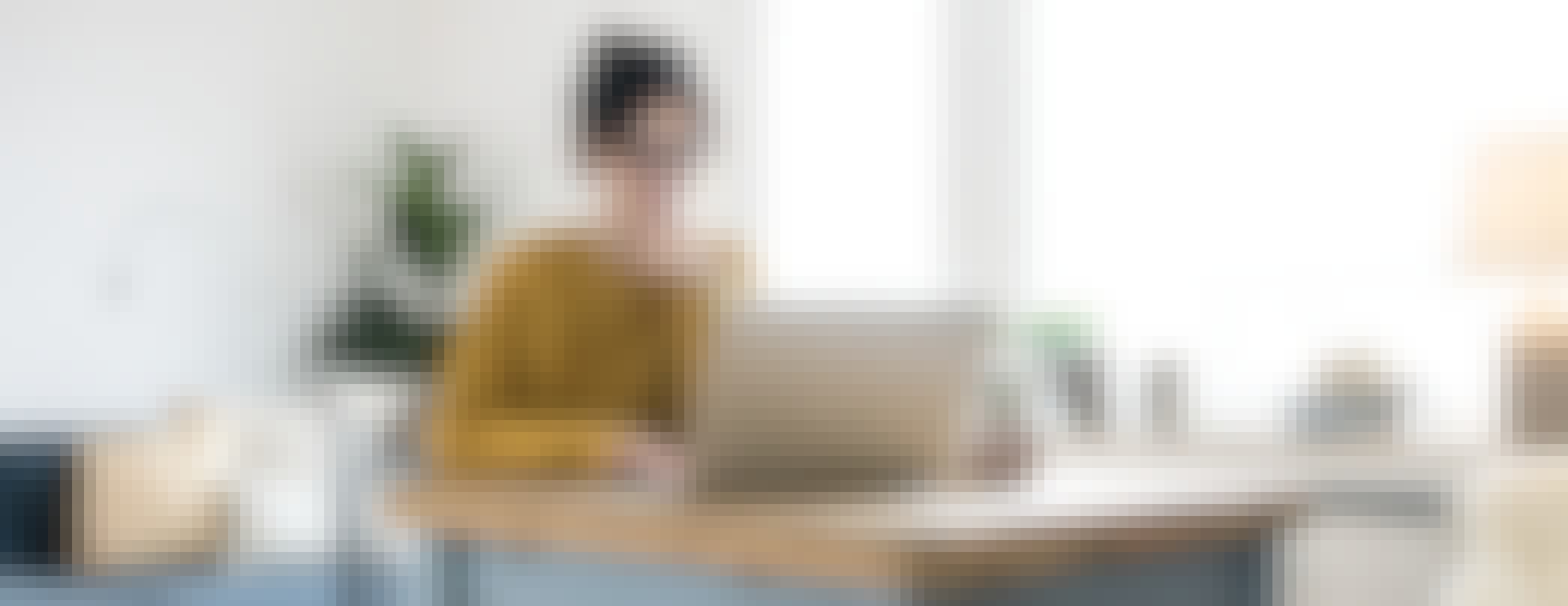
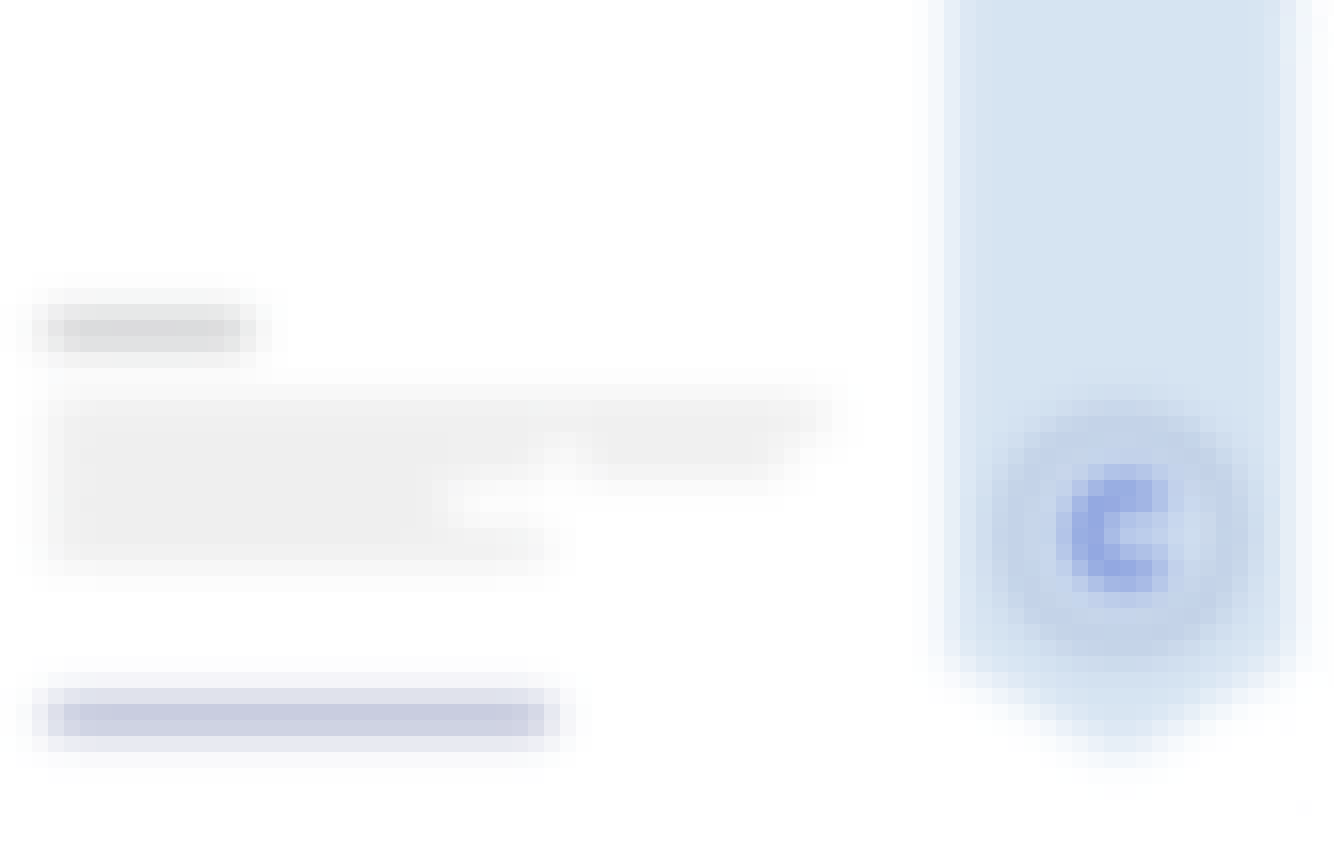
Earn a career certificate
Add this credential to your LinkedIn profile, resume, or CV
Share it on social media and in your performance review
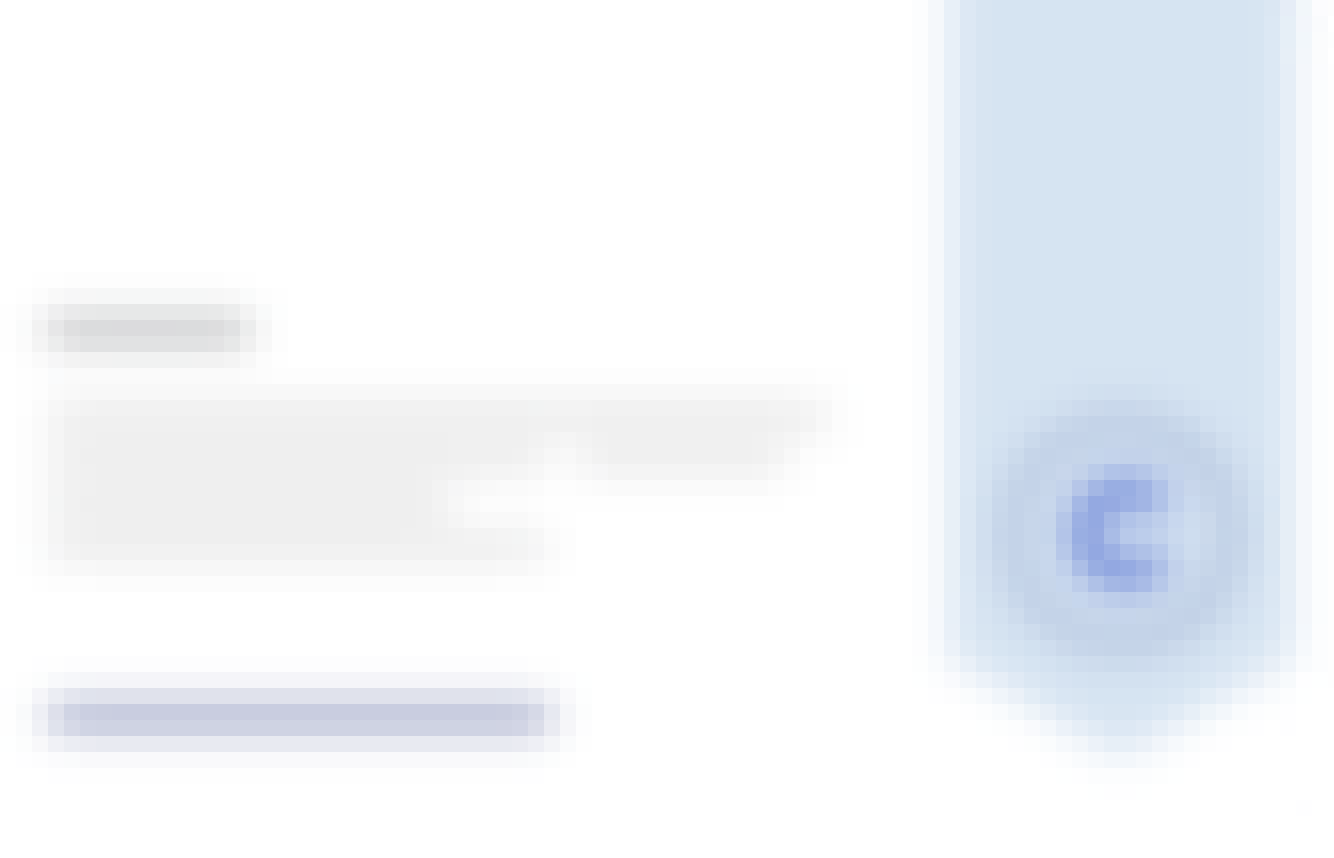
There are 5 modules in this course
The purpose of this module is for students to understand how the course will be run, topics, how they will be assessed, and expectations.
What's included
3 videos4 readings1 programming assignment1 discussion prompt1 ungraded lab
The single most important concept for using GPUs to solve complex and large-scale problems, is management of threads. CUDA provides two- and three-dimensional logical abstractions of threads, blocks and grids. Students will develop programs that utilize threads, blocks, and grids to process large 2 to 3-dimensional data sets.
What's included
8 videos1 reading2 assignments2 programming assignments1 ungraded lab
To manage the access and modification of data in physical memory effectively, students will need to load data into CPU (host) and GPU (global) general-purpose memory. Students will create software that allocates host memory and transfers it into global memory for use by threads. Students will also learn the capabilities and speeds of these types of memories.
What's included
8 videos1 assignment1 programming assignment1 discussion prompt2 ungraded labs
To improve performance in GPU software, students will need to utilized mutable (shared) and static (constant) memory. They will use them to apply masks to all items of a data set, to manage the communication between threads, and use for caching in complex programs.
What's included
6 videos1 assignment1 programming assignment1 discussion prompt1 ungraded lab
In this module, students will learn the benefits and constraints of GPUs most hyper-localized memory, registers. While using this type of memory will be natural for students, gaining the largest performance boost from it, like all forms of memory, will require thoughtful design of software. Students will develop implementations of algorithms using each type of memory and generate performance analysis.
What's included
5 videos1 assignment1 programming assignment1 discussion prompt1 ungraded lab
Instructor

Offered by
Why people choose Coursera for their career




Recommended if you're interested in Computer Science
Coursera Project Network
University of Colorado Boulder
University of California, Santa Cruz

Open new doors with Coursera Plus
Unlimited access to 10,000+ world-class courses, hands-on projects, and job-ready certificate programs - all included in your subscription
Advance your career with an online degree
Earn a degree from world-class universities - 100% online
Join over 3,400 global companies that choose Coursera for Business
Upskill your employees to excel in the digital economy