This second course of the AI Product Management Specialization by Duke University's Pratt School of Engineering focuses on the practical aspects of managing machine learning projects. The course walks through the keys steps of a ML project from how to identify good opportunities for ML through data collection, model building, deployment, and monitoring and maintenance of production systems. Participants will learn about the data science process and how to apply the process to organize ML efforts, as well as the key considerations and decisions in designing ML systems.

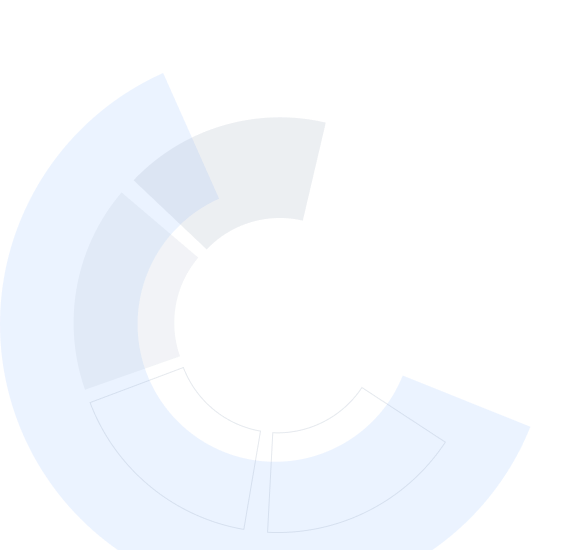
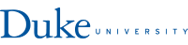
Managing Machine Learning Projects
This course is part of AI Product Management Specialization

Instructor: Jon Reifschneider
Sponsored by IEM UEM Group
16,656 already enrolled
(202 reviews)
Recommended experience
Skills you'll gain
- Project Management
- Data Quality
- Project Management Life Cycle
- Performance Metric
- Project Implementation
- Data Governance
- Computer Science
- Performance Management
- Artificial Intelligence
- Artificial Intelligence and Machine Learning (AI/ML)
- MLOps (Machine Learning Operations)
- Applied Machine Learning
- Machine Learning
- Product Development
- Solution Design
- Performance Measurement
- Technical Design
- Product Management
- Data Management
Details to know

Add to your LinkedIn profile
5 assignments
See how employees at top companies are mastering in-demand skills
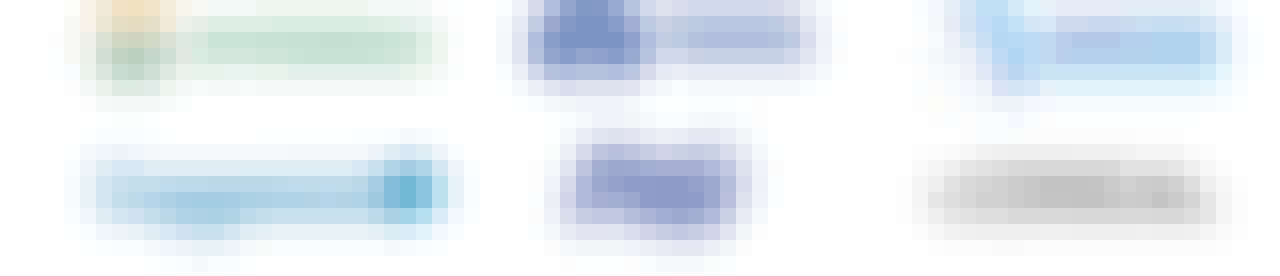
Build your subject-matter expertise
- Learn new concepts from industry experts
- Gain a foundational understanding of a subject or tool
- Develop job-relevant skills with hands-on projects
- Earn a shareable career certificate
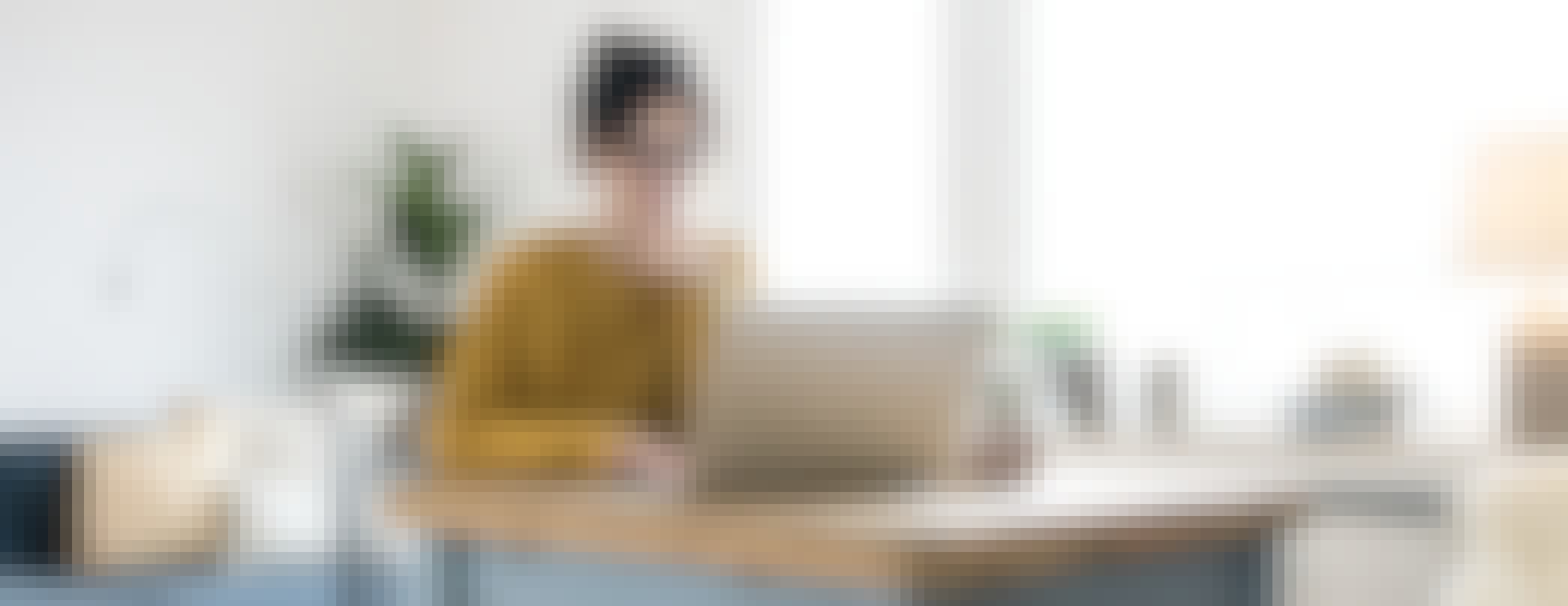
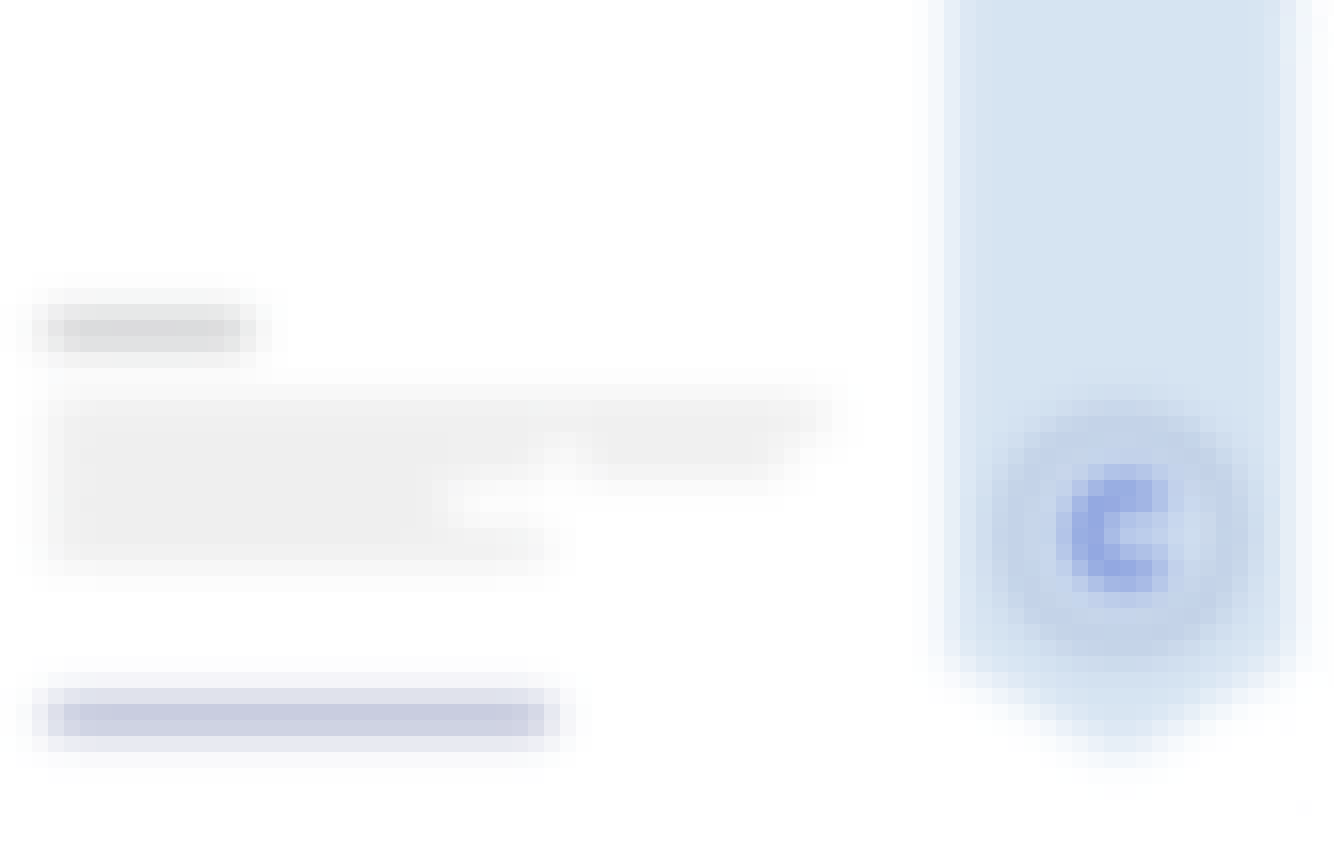
Earn a career certificate
Add this credential to your LinkedIn profile, resume, or CV
Share it on social media and in your performance review
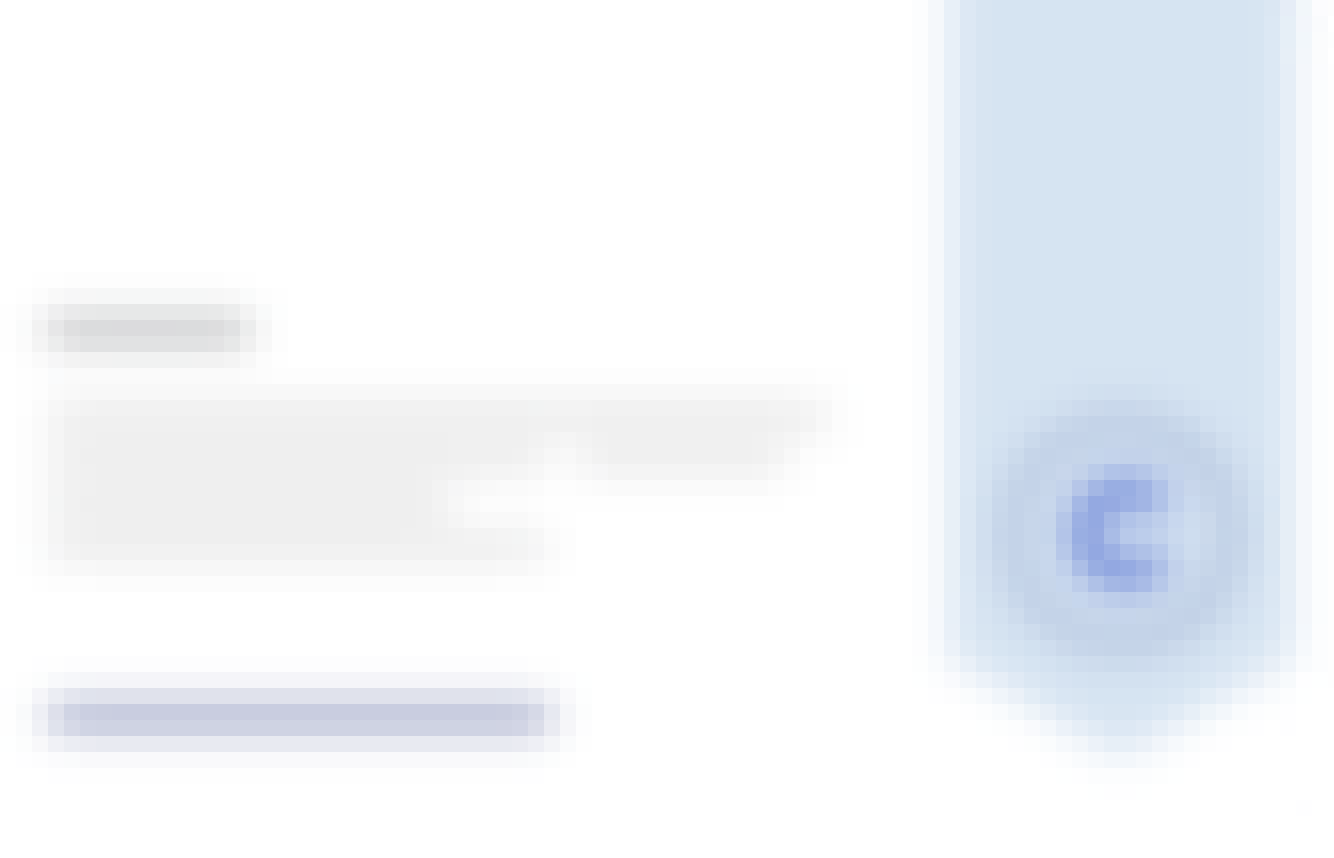
There are 5 modules in this course
In this module we will discuss how to identify problems worth solving, how to determine whether ML is a good fit as part of the solution, and how to validate solution concepts. We will also learn why heuristics are useful in modeling projects and the advantages and disadvantages of ML relative to heuristics.
What's included
9 videos4 readings1 assignment2 discussion prompts
In this module we will focus on the CRISP-DM data science process and how it can be used to organize ML projects. We will begin by understanding what is unique about ML project relative to normal software projects, and then discuss approaches to manage the inherent risks of ML projects. We will also walk through the key roles on a ML project team and how to organize work.
What's included
8 videos2 readings1 assignment1 discussion prompt
In this module we will explore the key data-related issues that arise in ML projects. Data is the foundation of successful machine learning, and gathering data of sufficient quantity and quality with the right set of attributes is the key to a successful project. We will discuss the key considerations in sourcing data, cleaning data, and developing and selecting a feature set to use in modeling. The module will conclude with a discussion on best practices to ensure reproducibility of your data pipeline.
What's included
8 videos2 readings1 assignment1 discussion prompt
In this module we will discuss the key decisions to make in designing ML systems, such as cloud vs. edge and online vs. batch, and compare the benefits of each type of system. We will then discuss the primary technology decisions to make in a ML project and introduce the common tools and technologies used to build ML models.
What's included
8 videos2 readings1 assignment1 discussion prompt
The final module in the course focuses on identifying and mitigating the key issues which ML models experience once they are in production. We will discuss how to set up a robust ML system monitoring capability and define a model maintenance plan to maintain high performance of a production model. We will conclude with a discussion on the importance of versioning in ML systems to facilitate continued rapid iteration even after deployment.
What's included
8 videos2 readings1 assignment1 peer review1 discussion prompt1 plugin
Instructor

Offered by
Why people choose Coursera for their career




Learner reviews
202 reviews
- 5 stars
83.66%
- 4 stars
11.38%
- 3 stars
2.97%
- 2 stars
0.99%
- 1 star
0.99%
Showing 3 of 202
Reviewed on Jun 29, 2023
I appreciate the use cases that were shared throughout the course. It helped tremendously.
Reviewed on Sep 29, 2024
Helps you very much to identify good potential AI projects
Reviewed on Sep 3, 2023
The peer rating for the final project is interesting, if someone who does not get what is being asked for the final project is going to rate my final project. Saw some interesting examples.
Recommended if you're interested in Data Science
Amazon Web Services
Duke University
DeepLearning.AI

Open new doors with Coursera Plus
Unlimited access to 10,000+ world-class courses, hands-on projects, and job-ready certificate programs - all included in your subscription
Advance your career with an online degree
Earn a degree from world-class universities - 100% online
Join over 3,400 global companies that choose Coursera for Business
Upskill your employees to excel in the digital economy