This is the third and final course in the Linear Algebra Specialization that focuses on the theory and computations that arise from working with orthogonal vectors. This includes the study of orthogonal transformation, orthogonal bases, and orthogonal transformations. The course culminates in the theory of symmetric matrices, linking the algebraic properties with their corresponding geometric equivalences. These matrices arise more often in applications than any other class of matrices.

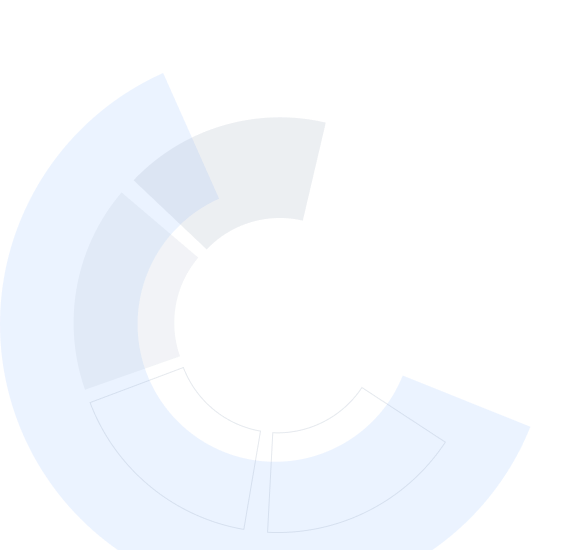
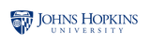
Linear Algebra: Orthogonality and Diagonalization
This course is part of Linear Algebra from Elementary to Advanced Specialization

Instructor: Joseph W. Cutrone, PhD
Top Instructor
Sponsored by IEM UEM Group
1,712 already enrolled
(30 reviews)
Details to know

Add to your LinkedIn profile
11 assignments
See how employees at top companies are mastering in-demand skills
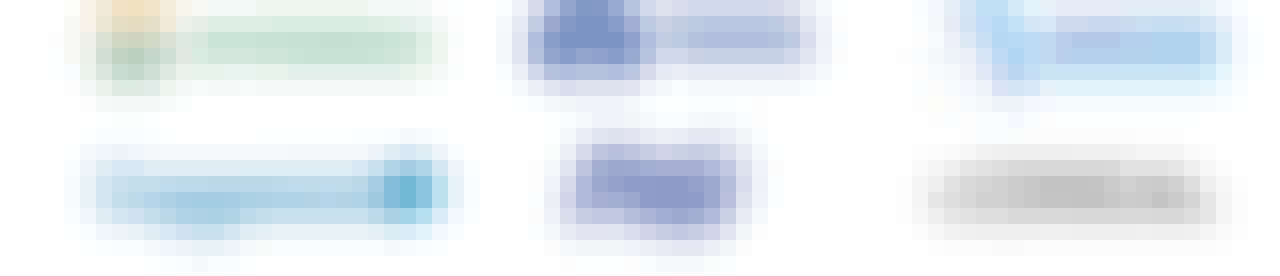
Build your subject-matter expertise
- Learn new concepts from industry experts
- Gain a foundational understanding of a subject or tool
- Develop job-relevant skills with hands-on projects
- Earn a shareable career certificate
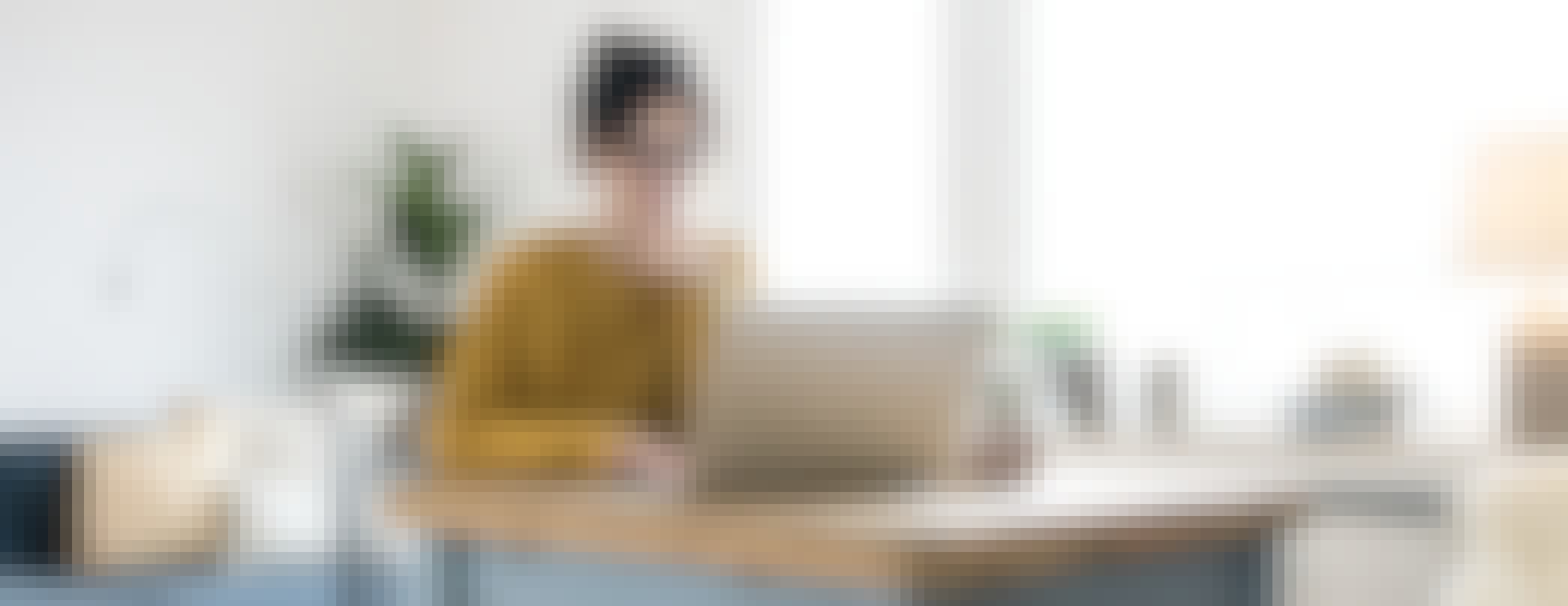
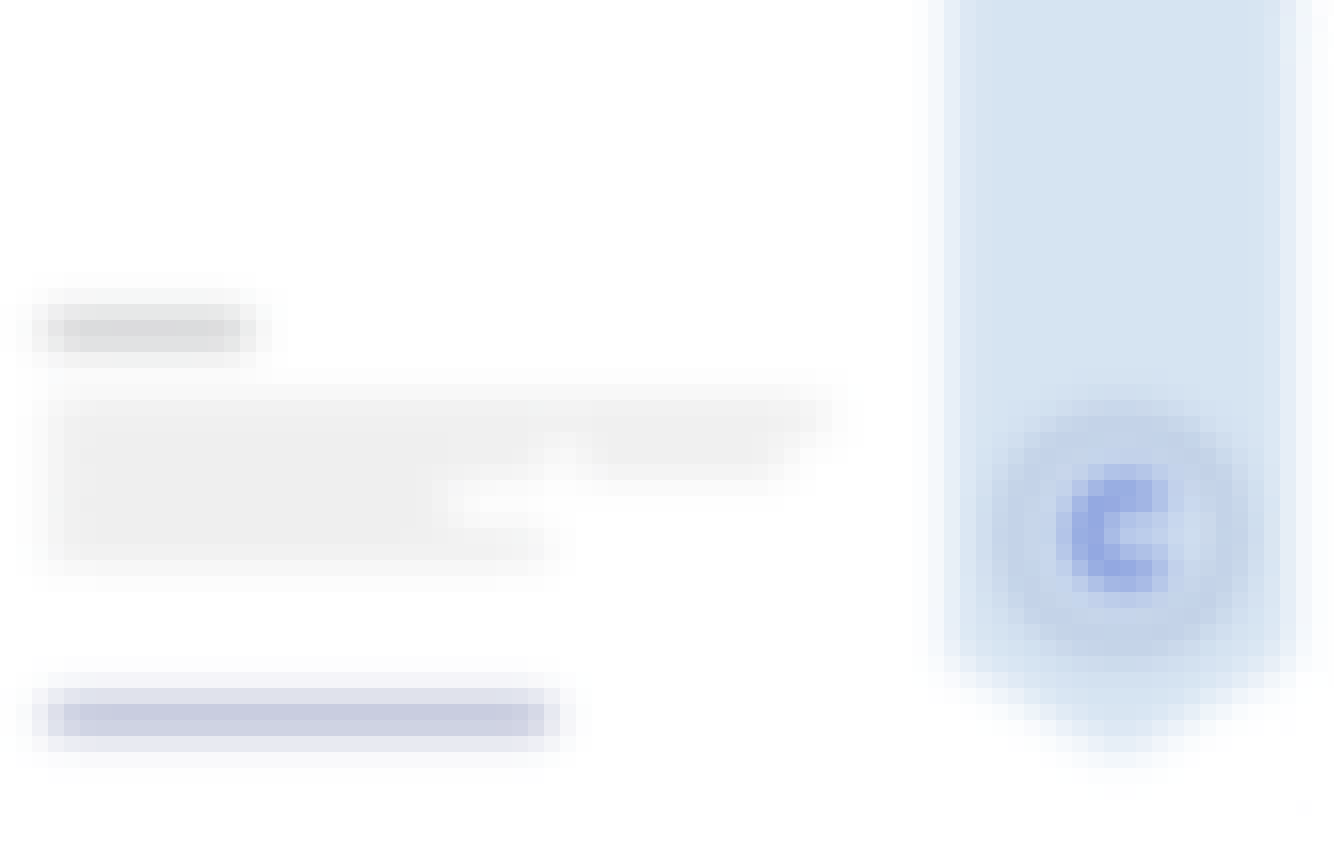
Earn a career certificate
Add this credential to your LinkedIn profile, resume, or CV
Share it on social media and in your performance review
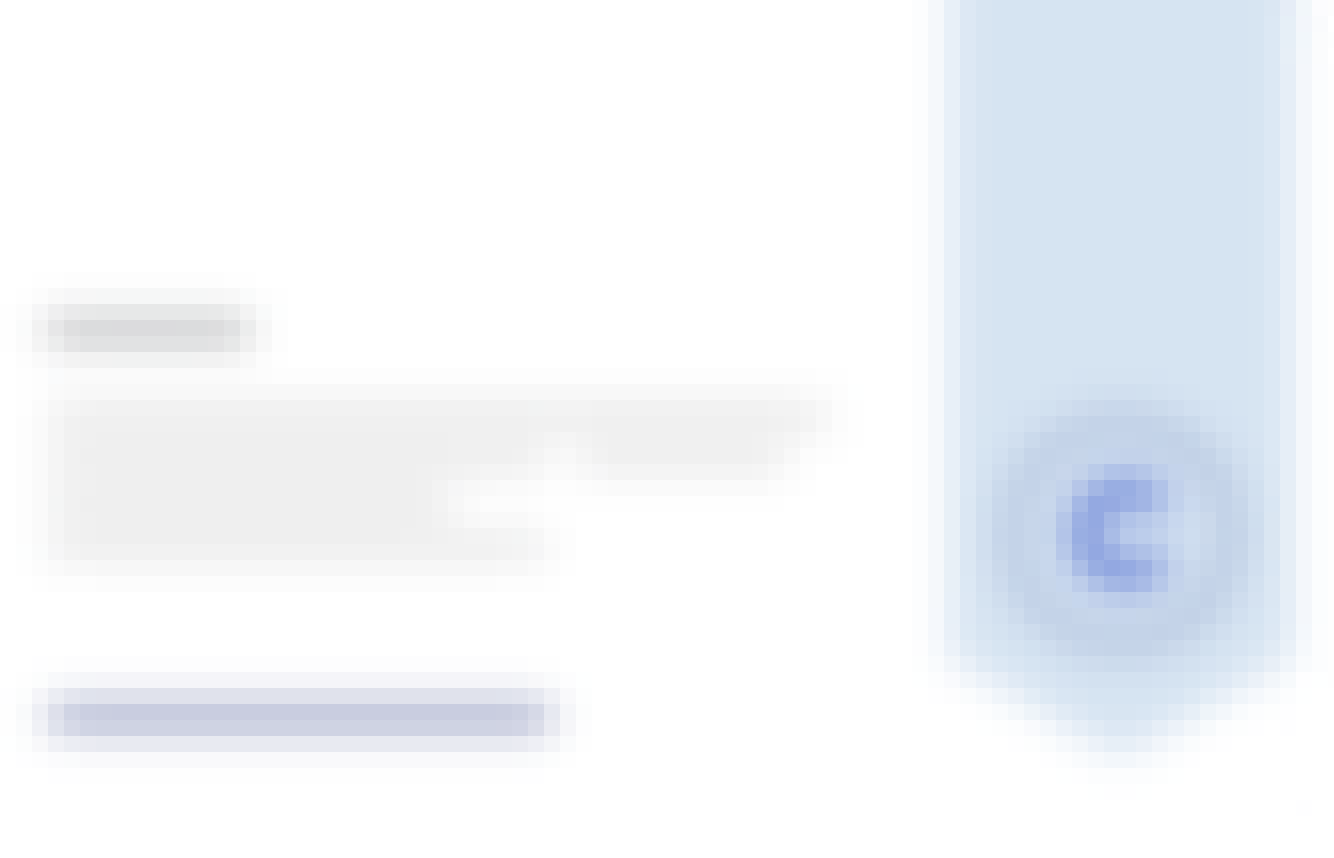
There are 4 modules in this course
In this module, we define a new operation on vectors called the dot product. This operation is a function that returns a scalar related to the angle between the vectors, distance between vectors, and length of vectors. After working through the theory and examples, we hone in on both unit (length one) and orthogonal (perpendicular) vectors. These special vectors will be pivotal in our course as we start to define linear transformations and special matrices that use only these vectors.
What's included
2 videos2 readings3 assignments
In this module we will study the special type of transformation called the orthogonal projection. We have already seen the formula for the orthogonal projection onto a line so now we generalize the formula to the case of projection onto any subspace W. The formula will require basis vectors that are both orthogonal and normalize and we show, using the Gram-Schmidt Process, how to meet these requirements given any non-empty basis.
What's included
3 videos3 readings4 assignments
In this module we look to diagonalize symmetric matrices. The symmetry displayed in the matrix A turns out to force a beautiful relationship between the eigenspaces. The corresponding eigenspaces turn out to be mutually orthogonal. After normalizing, these orthogonal eigenvectors give a very special basis of R^n with extremely useful applications to data science, machine learning, and image processing. We introduce the notion of quadratic forms, special functions of degree two on vectors , which use symmetric matrices in their definition. Quadratic forms are then completely classified based on the properties of their eigenvalues.
What's included
2 videos2 readings3 assignments
What's included
1 assignment
Instructor

Offered by
Why people choose Coursera for their career




Learner reviews
30 reviews
- 5 stars
93.33%
- 4 stars
6.66%
- 3 stars
0%
- 2 stars
0%
- 1 star
0%
Showing 3 of 30
Reviewed on Nov 4, 2024
It is great, the guy on the videos knows a lot, its a pity he writes so fast :))
Reviewed on Dec 8, 2024
Teach good. It explore some of my blind areas about diagonalization, eigen and orthogonal, repeated roots concern, etc.
Recommended if you're interested in Data Science
Johns Hopkins University
Johns Hopkins University
IIT Roorkee
The University of Sydney

Open new doors with Coursera Plus
Unlimited access to 10,000+ world-class courses, hands-on projects, and job-ready certificate programs - all included in your subscription
Advance your career with an online degree
Earn a degree from world-class universities - 100% online
Join over 3,400 global companies that choose Coursera for Business
Upskill your employees to excel in the digital economy