Explore the dynamic world of satellite remote sensing data through a comprehensive bootcamp that equips you with essential skills using open-source tools. Beginning with the fundamentals, you'll be introduced to the core concepts of remote sensing, including various data types and the tools essential for their analysis, such as R and QGIS. As you progress, you will delve into the intricacies of optical remote sensing, learning to download, preprocess, and interpret Landsat data while mastering tools like the Semi-Automatic Classification Plugin in QGIS.

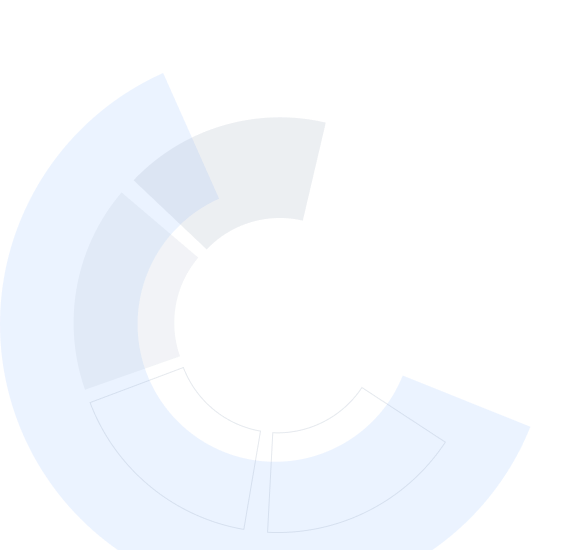
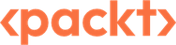
Satellite Remote Sensing Data Bootcamp With Opensource Tools

Instructor: Packt - Course Instructors
Sponsored by IEM UEM Group
Recommended experience
What you'll learn
Analyze different types of satellite remote sensing data
Preprocess optical data using atmospheric correction techniques
Classify remote sensing data using both supervised and unsupervised methods
Handle SAR data, including preprocessing and speckle filtering
Skills you'll gain
- Machine Learning Methods
- GIS Software
- Spatial Analysis
- Data Engineering
- Data Processing
- Statistical Machine Learning
- Data Analysis
- Machine Learning
- Geospatial Information and Technology
- Applied Machine Learning
- Spatial Data Analysis
- Artificial Intelligence and Machine Learning (AI/ML)
- Data Science
- Information Management
- Geographic Information Systems
- Data Cleansing
- Extract, Transform, Load
- Data Validation
- Data Manipulation
- Unsupervised Learning
Details to know

Add to your LinkedIn profile
3 assignments
October 2024
See how employees at top companies are mastering in-demand skills
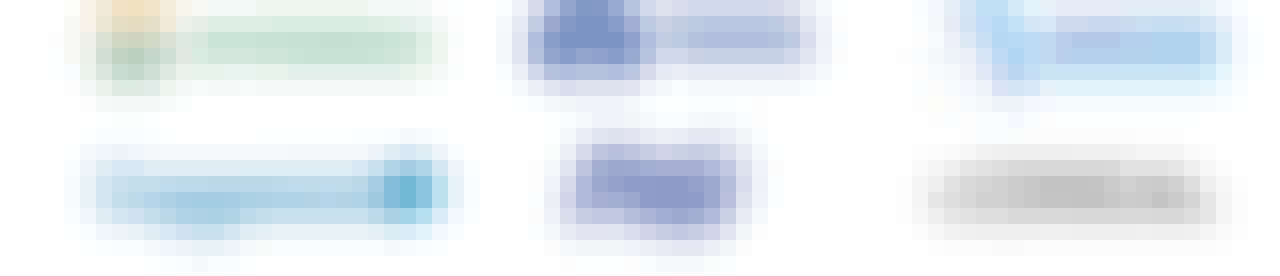
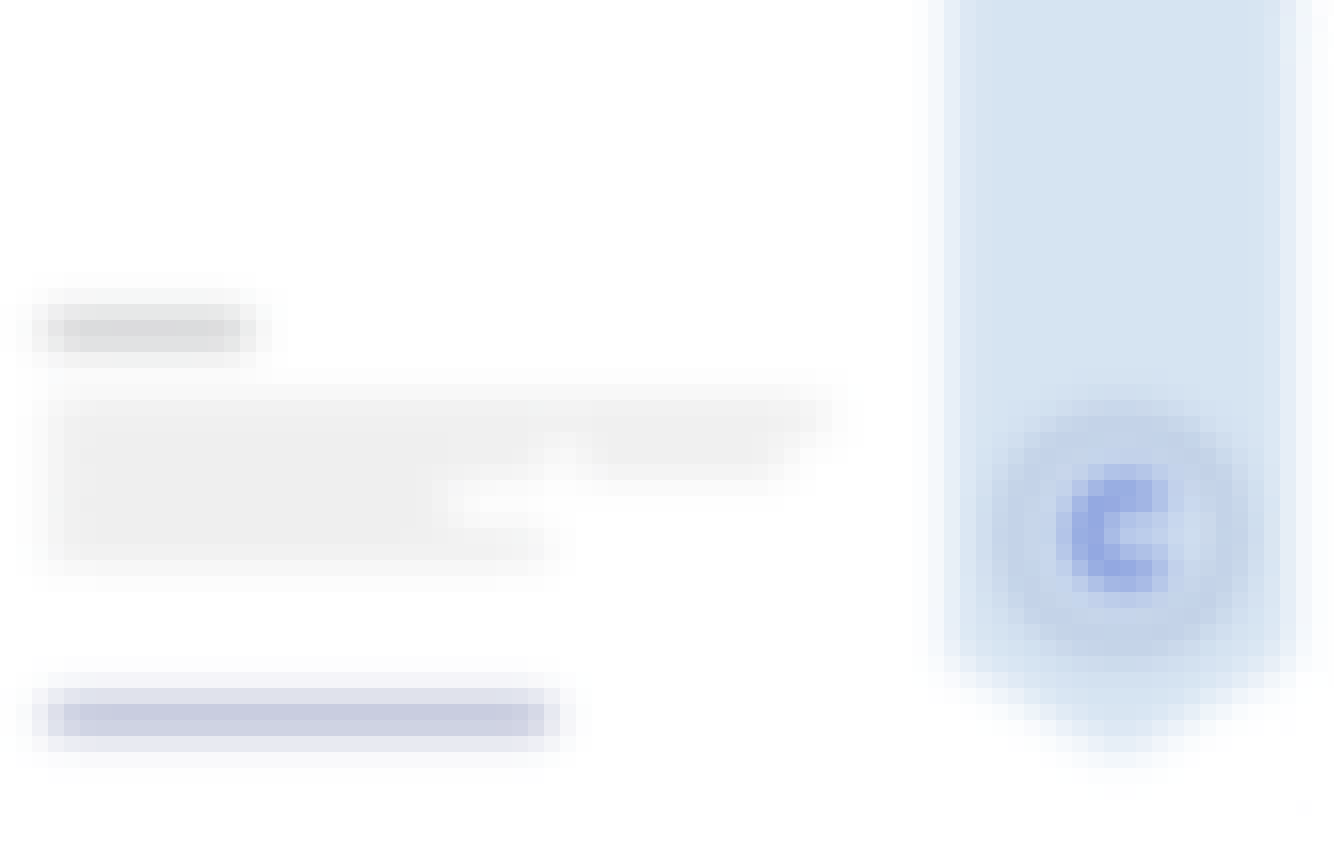
Earn a career certificate
Add this credential to your LinkedIn profile, resume, or CV
Share it on social media and in your performance review
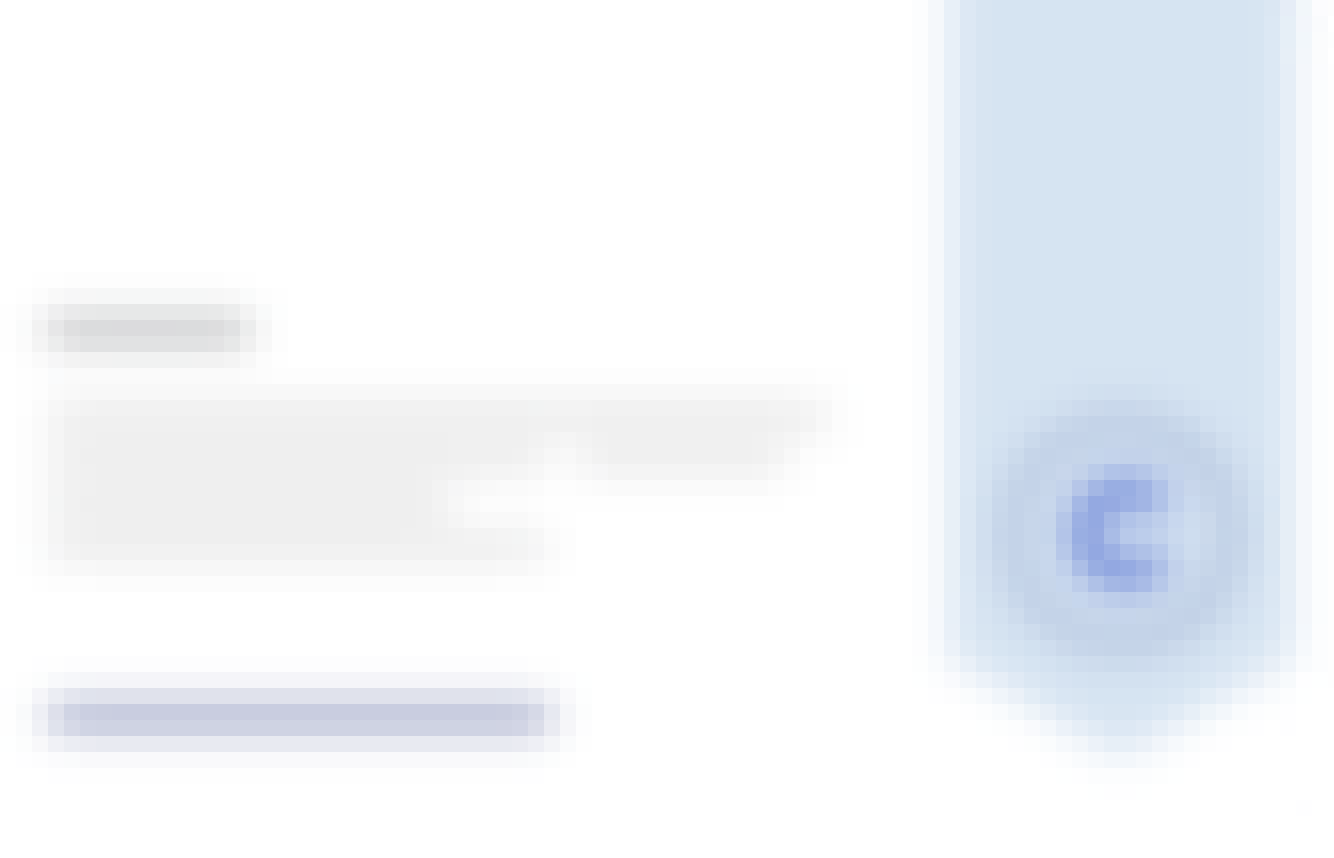
There are 6 modules in this course
In this module, we will lay the groundwork for your journey into satellite remote sensing data analysis. You'll begin by learning about the course structure, then explore the fundamentals of remote sensing, different data types, and the essential tools you will use throughout the course. By the end of this module, you'll have a solid understanding of the basics and be ready to dive deeper into the practical aspects of the field.
What's included
7 videos1 reading
In this module, we will delve into the world of optical remote sensing data, starting with the fundamental principles that govern its collection. You'll examine the different types of optical data and how they are used, particularly focusing on Landsat data. Additionally, you'll explore the specifics of Landsat sensors and gain hands-on experience in using QGIS to download and view this data. By the end of this section, you'll be equipped with the knowledge and skills needed to work with optical remote sensing data in your analyses.
What's included
6 videos
In this module, we will focus on the crucial steps involved in pre-processing optical remote sensing data. You'll learn why pre-processing is essential, particularly for improving data accuracy. The module will guide you through performing atmospheric correction on Landsat data using R, and introduce you to the Semi-Automatic Classification Plugin in QGIS for efficient pre-processing. Additionally, you'll assess the quality of atmospherically corrected outputs and explore the practical applications of pre-processed data. By the end of this section, you'll have the skills to refine raw satellite data for meaningful analysis.
What's included
6 videos1 assignment
In this module, we will explore the diverse applications of optical remote sensing data across various analytical processes. You'll begin by mastering band manipulation in QGIS, followed by the application of band math to derive critical insights. The module will introduce you to texture indices and tasseled cap transformations, offering both theoretical knowledge and practical implementation using GRASS GIS and ESA SNAP. Additionally, you'll delve into vegetation indices and learn how to reduce data dimensionality for more efficient analysis. By the end of this section, you'll be well-versed in multiple advanced techniques for leveraging optical data in your projects
What's included
13 videos
In this module, we will delve into the classification of remote sensing satellite data, covering both unsupervised and supervised methods. You’ll begin by exploring the theory behind these approaches, followed by practical applications using ESA SNAP and QGIS. The module also introduces machine learning concepts and their integration into remote sensing classification, guiding you through creating training data and applying advanced algorithms to satellite imagery. By the end of this section, you’ll be equipped with comprehensive skills to classify and analyze remote sensing data accurately and efficiently
What's included
9 videos
In this module, we will explore active remote sensing data, focusing on Synthetic Aperture Radar (SAR). You'll begin by understanding the reasons for using active remote sensing over passive methods, with a particular emphasis on SAR technology. The module will guide you through the process of obtaining ALOS PALSAR data and applying essential pre-processing steps. You'll also learn to filter speckles from SAR imagery to improve data quality, and finally, you'll extract back-scatter values, a critical step for interpreting SAR data. By the end of this section, you'll have a solid foundation in working with active remote-sensing data
What's included
5 videos2 assignments
Instructor

Offered by
Why people choose Coursera for their career




Recommended if you're interested in Physical Science and Engineering
École Polytechnique Fédérale de Lausanne
University of Toronto
UNSW Sydney (The University of New South Wales)

Open new doors with Coursera Plus
Unlimited access to 10,000+ world-class courses, hands-on projects, and job-ready certificate programs - all included in your subscription
Advance your career with an online degree
Earn a degree from world-class universities - 100% online
Join over 3,400 global companies that choose Coursera for Business
Upskill your employees to excel in the digital economy