In Retrieval Optimization: Tokenization to Vector Quantization, taught by Kacper Łukawski, Developer Relations Lead of Qdrant, you’ll learn all about tokenization and also how to optimize vector search in your large-scale customer-facing RAG applications. You’ll explore the technical details of how vector search works and how to optimize it for better performance.

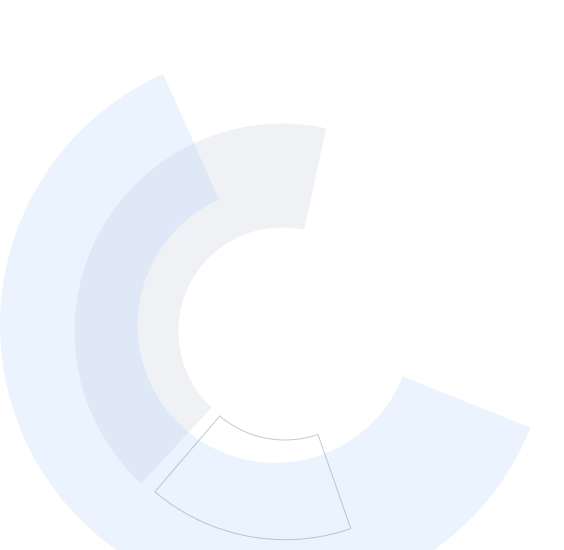
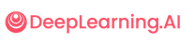
Recommended experience
What you'll learn
Learn how tokenization works in large language and embedding models and how the tokenizer can affect the quality of your search.
Explore how different tokenization techniques including Byte-Pair Encoding, WordPiece, and Unigram are trained and work.
Understand how to measure the quality of your retrieval and how to optimize your search by adjusting HNSW parameters and vector quantizations.
Details to know
October 2024
Only available on desktop
See how employees at top companies are mastering in-demand skills
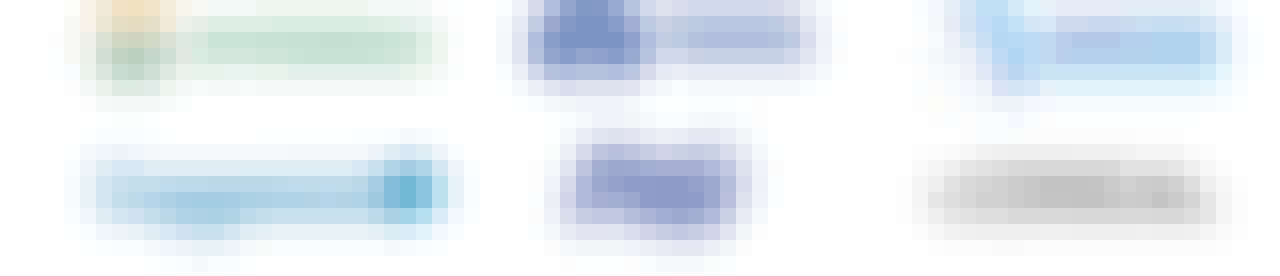
Learn, practice, and apply job-ready skills in less than 2 hours
- Receive training from industry experts
- Gain hands-on experience solving real-world job tasks
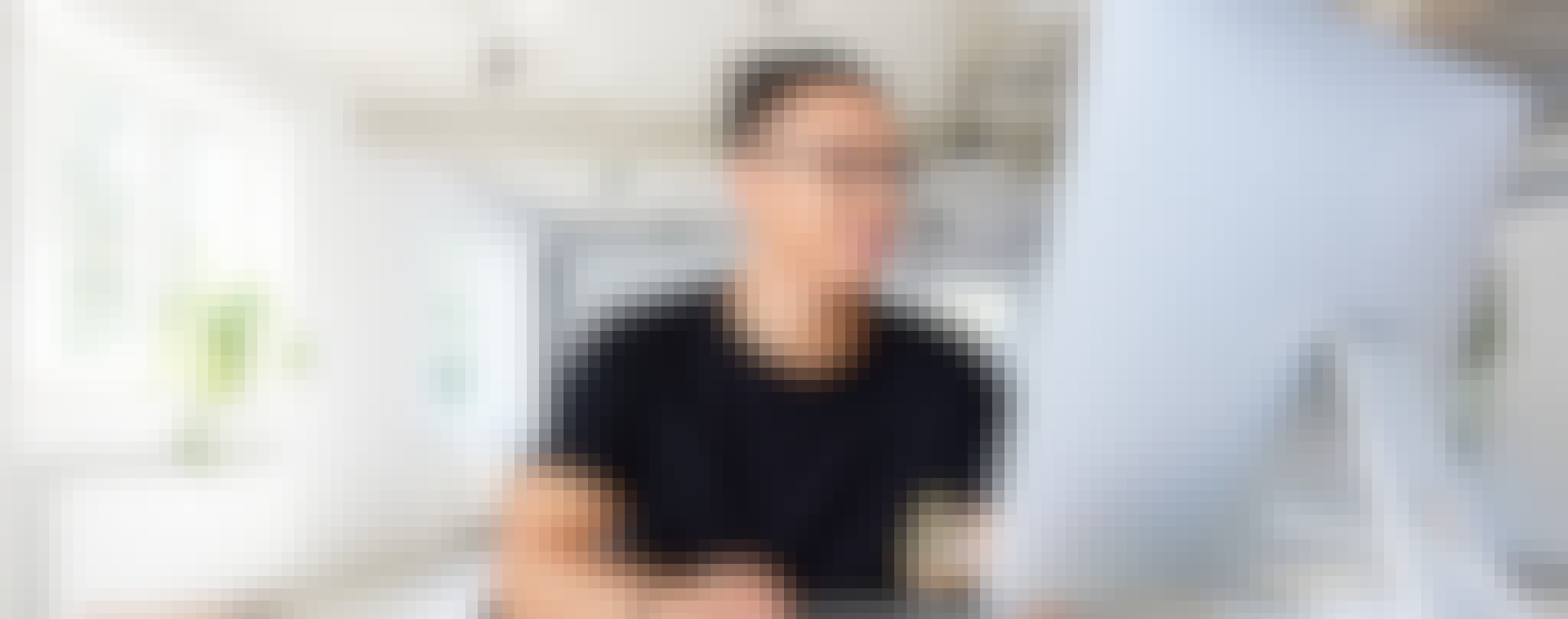
About this project
Instructor

Offered by
How you'll learn
Hands-on, project-based learning
Practice new skills by completing job-related tasks with step-by-step instructions.
No downloads or installation required
Access the tools and resources you need in a cloud environment.
Available only on desktop
This project is designed for laptops or desktop computers with a reliable Internet connection, not mobile devices.
Why people choose Coursera for their career




You might also like
Stanford University
Coursera Project Network
New to Software Development? Start here.

Open new doors with Coursera Plus
Unlimited access to 7,000+ world-class courses, hands-on projects, and job-ready certificate programs - all included in your subscription
Advance your career with an online degree
Earn a degree from world-class universities - 100% online
Join over 3,400 global companies that choose Coursera for Business
Upskill your employees to excel in the digital economy
Frequently asked questions
In Projects, you'll complete an activity or scenario by following a set of instructions in an interactive hands-on environment. Projects are completed in a real cloud environment and within real instances of various products as opposed to a simulation or demo environment.
By purchasing a Project, you'll get everything you need to complete the Project including temporary access to any product required to complete the Project.
Even though Projects are technically available on mobile devices, we highly recommend that you complete Projects on a laptop or desktop only.