This course is the second in a specialization for Machine Learning for Supply Chain Fundamentals. In this course, we explore all aspects of time series, especially for demand prediction. We'll start by gaining a foothold in the basic concepts surrounding time series, including stationarity, trend (drift), cyclicality, and seasonality. Then, we'll spend some time analyzing correlation methods in relation to time series (autocorrelation). In the 2nd half of the course, we'll focus on methods for demand prediction using time series, such as autoregressive models. Finally, we'll conclude with a project, predicting demand using ARIMA models in Python.

Schenken Sie Ihrer Karriere Coursera Plus mit einem Rabatt von $160 , der jährlich abgerechnet wird. Sparen Sie heute.
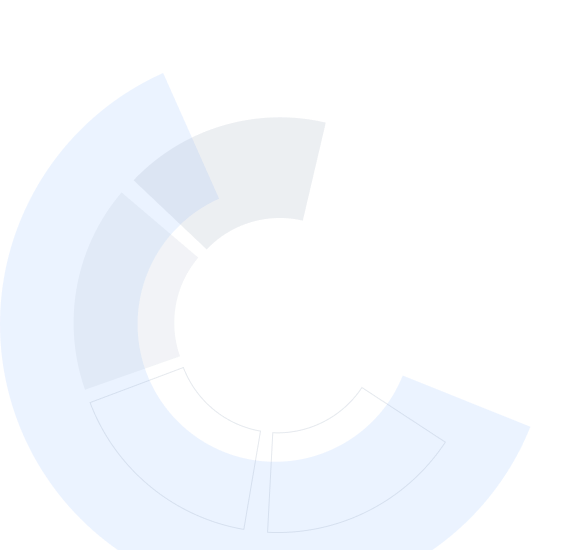
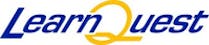
Demand Forecasting Using Time Series
Dieser Kurs ist Teil von Spezialisierung Machine Learning for Supply Chains


Dozenten: Rajvir Dua
3.437 bereits angemeldet
Bei enthalten
(31 Bewertungen)
Empfohlene Erfahrung
Was Sie lernen werden
Building ARIMA models in Python to make demand predictions
Developing the framework for more advanced neural netowrks (such as LSTMs) by understanding autocorrelation and autoregressive models.
Kompetenzen, die Sie erwerben
- Kategorie: Python Programming
- Kategorie: Autoregressive Integrated Moving Average (ARIMA)
- Kategorie: Time Series
- Kategorie: Machine Learning
- Kategorie: Demand Forecasting
Wichtige Details

Zu Ihrem LinkedIn-Profil hinzufügen
5 Aufgaben
Erfahren Sie, wie Mitarbeiter führender Unternehmen gefragte Kompetenzen erwerben.
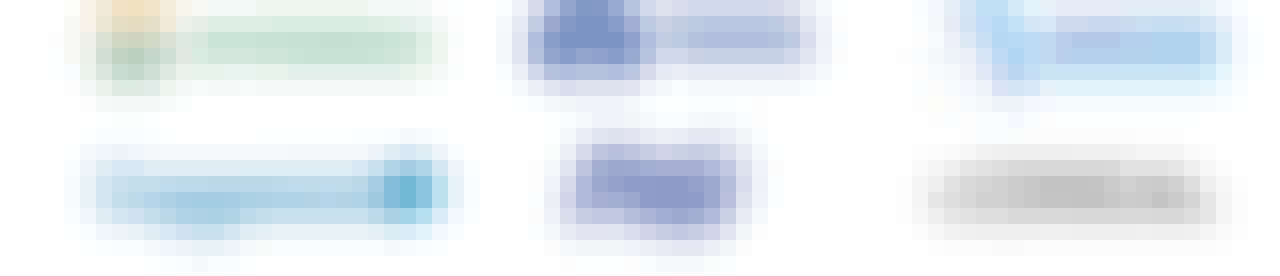
Erweitern Sie Ihre Fachkenntnisse
- Lernen Sie neue Konzepte von Branchenexperten
- Gewinnen Sie ein Grundverständnis bestimmter Themen oder Tools
- Erwerben Sie berufsrelevante Kompetenzen durch praktische Projekte
- Erwerben Sie ein Berufszertifikat zur Vorlage
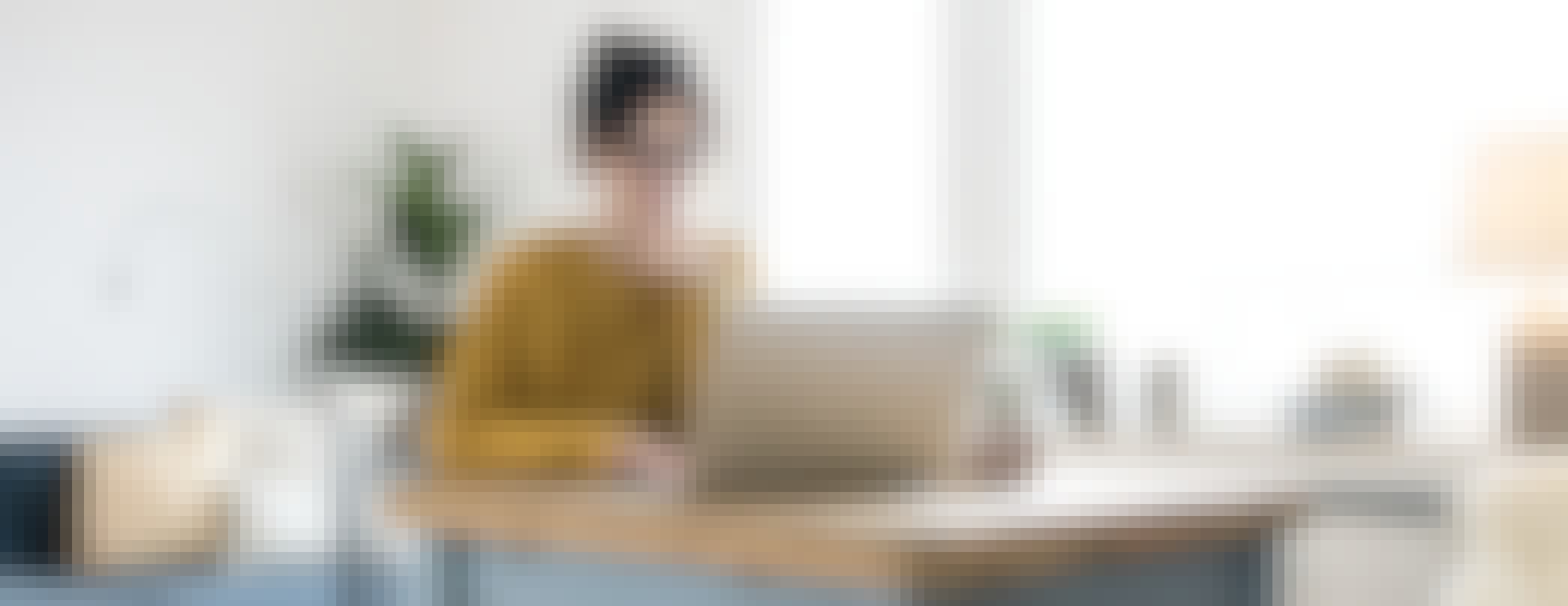
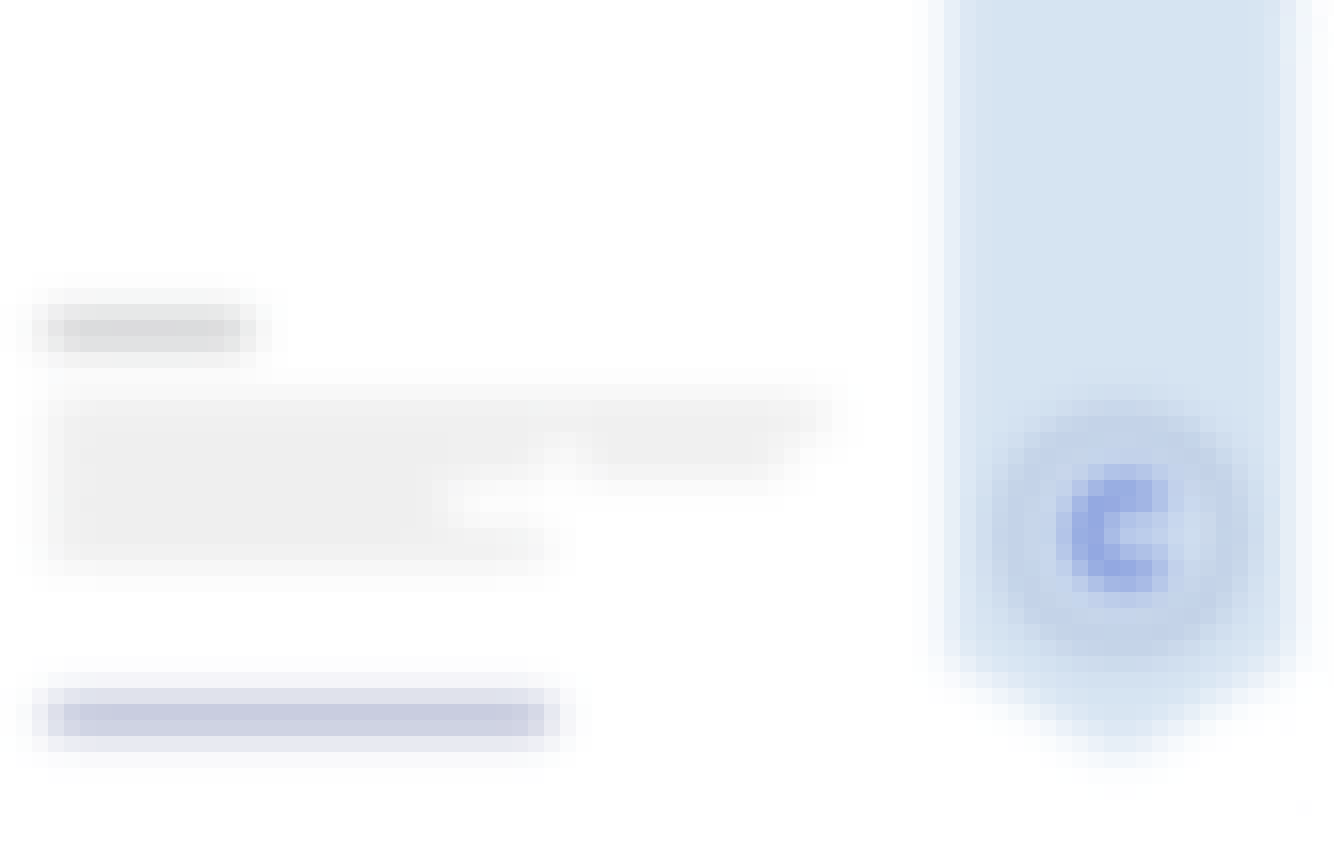
Erwerben Sie ein Karrierezertifikat.
Fügen Sie diese Qualifikation zur Ihrem LinkedIn-Profil oder Ihrem Lebenslauf hinzu.
Teilen Sie es in den sozialen Medien und in Ihrer Leistungsbeurteilung.
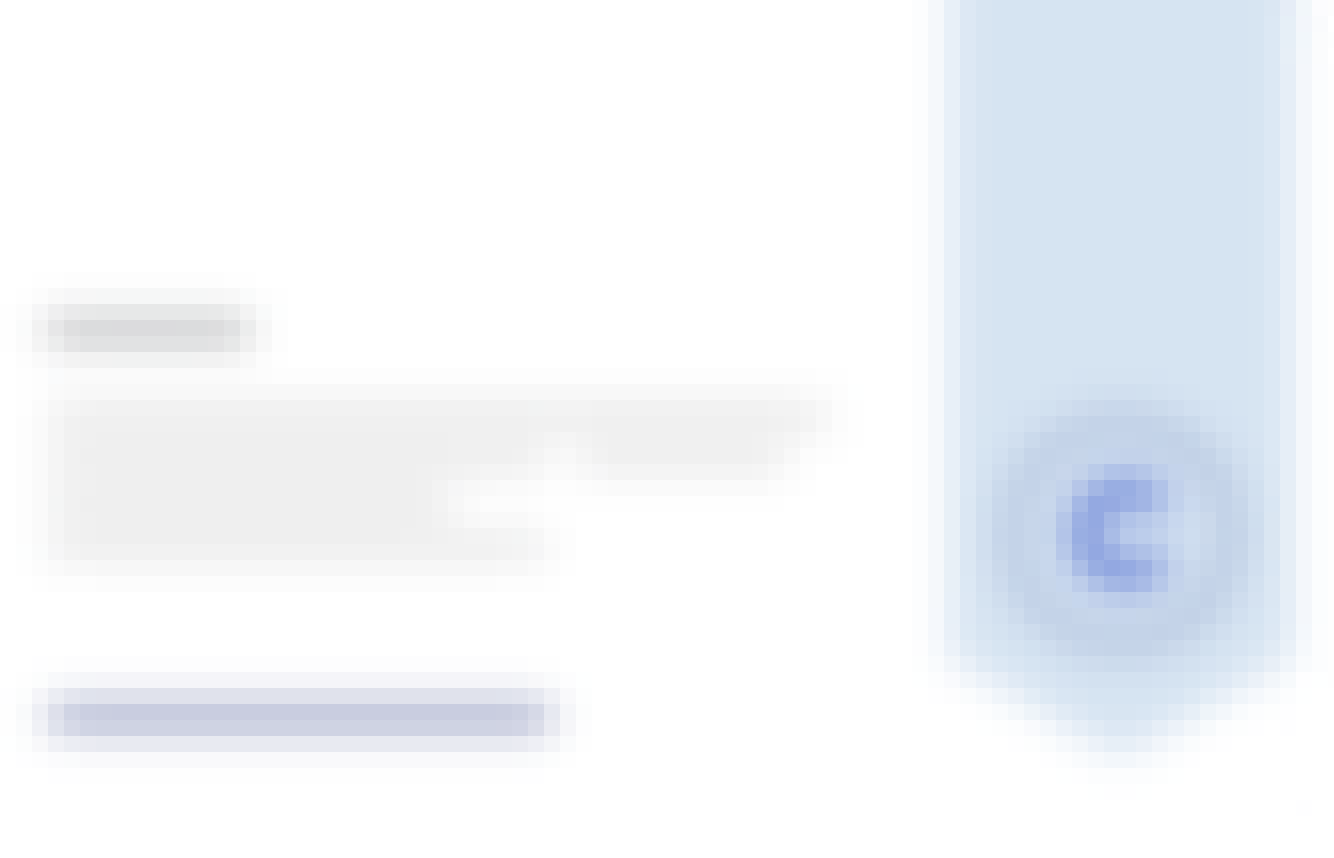
In diesem Kurs gibt es 4 Module
In this module, we'll get our feet wet with time series in Python. We'll start by getting familiar with where time series fits in to the machine learning landscape. Then, we'll learn about the main types of time series and their distinguishing factors, including period, frequency, and stationarity. After pausing to learn how to plot timeseries in Python, we'll explore the differences between seasonality and cyclicality.
Das ist alles enthalten
7 Videos3 Lektüren2 Aufgaben1 Diskussionsthema
In this module, we'll dive into the ideas behind autocorrelation and independence. We'll start by digging into the math of correlation and how it can be used to characterize the relationship between two variables. Next, we'll define its relationship to independence and explain where these ideas can be used. Finally, we'll combine correlation with time series attributes, such as trend, seasonality, and stationarity to derive autocorrelation. We'll go through both some of the theory behind autocorrelation, and how to code it in Python.
Das ist alles enthalten
8 Videos2 Lektüren2 Aufgaben1 Diskussionsthema
In this module, we'll start by reviewing some of the basic concepts behind linear regression. Then, we'll extend this knowledge to feed into lagged regression, an effective way to use regression techniques on time series. Once we have a solid foothold in basic and lagged regression, we'll explore modern methods such as ARIMA (autoregressive integrated moving average). All of this is building the framework for more advanced machine learning models such as LSTMs (long short-term memory network).
Das ist alles enthalten
4 Videos1 Lektüre1 Aufgabe1 Programmieraufgabe1 Diskussionsthema1 Unbewertetes Labor
In the final course project, we'll make demand predictions using ARIMA models.
Das ist alles enthalten
1 Programmieraufgabe1 Unbewertetes Labor
von
Empfohlen, wenn Sie sich für Machine Learning interessieren
Google Cloud
LearnQuest
Queen Mary University of London
Warum entscheiden sich Menschen für Coursera für ihre Karriere?




Bewertungen von Lernenden
Zeigt 3 von 31
31 Bewertungen
- 5 stars
46,87 %
- 4 stars
6,25 %
- 3 stars
9,37 %
- 2 stars
15,62 %
- 1 star
21,87 %
Geprüft am 12. Sep. 2022

Neue Karrieremöglichkeiten mit Coursera Plus
Unbegrenzter Zugang zu über 7.000 erstklassigen Kursen, praktischen Projekten und Zertifikatsprogrammen, die Sie auf den Beruf vorbereiten – alles in Ihrem Abonnement enthalten
Bringen Sie Ihre Karriere mit einem Online-Abschluss voran.
Erwerben Sie einen Abschluss von erstklassigen Universitäten – 100 % online
Schließen Sie sich mehr als 3.400 Unternehmen in aller Welt an, die sich für Coursera for Business entschieden haben.
Schulen Sie Ihre Mitarbeiter*innen, um sich in der digitalen Wirtschaft zu behaupten.
Häufig gestellte Fragen
Access to lectures and assignments depends on your type of enrollment. If you take a course in audit mode, you will be able to see most course materials for free. To access graded assignments and to earn a Certificate, you will need to purchase the Certificate experience, during or after your audit. If you don't see the audit option:
The course may not offer an audit option. You can try a Free Trial instead, or apply for Financial Aid.
The course may offer 'Full Course, No Certificate' instead. This option lets you see all course materials, submit required assessments, and get a final grade. This also means that you will not be able to purchase a Certificate experience.
When you enroll in the course, you get access to all of the courses in the Specialization, and you earn a certificate when you complete the work. Your electronic Certificate will be added to your Accomplishments page - from there, you can print your Certificate or add it to your LinkedIn profile. If you only want to read and view the course content, you can audit the course for free.
If you subscribed, you get a 7-day free trial during which you can cancel at no penalty. After that, we don’t give refunds, but you can cancel your subscription at any time. See our full refund policy.