Embark on an enlightening journey into the realm of Generative Adversarial Networks (GANs), where you will master the art of AI-driven image synthesis. This course begins with a solid foundation, introducing you to the basic concepts and components of GANs, such as the Generator and Discriminator. From there, you will delve into the intricacies of fully connected and deep convolutional GANs, understanding their architectures, and learning how to implement and optimize them effectively.

Schenken Sie Ihrer Karriere Coursera Plus mit einem Rabatt von $160 , der jährlich abgerechnet wird. Sparen Sie heute.
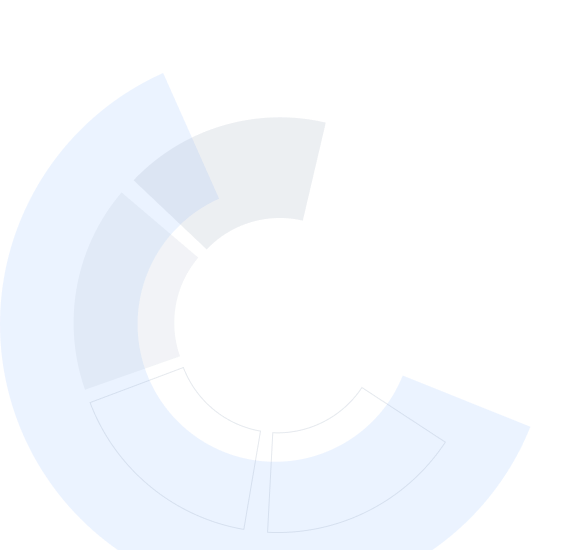
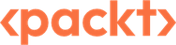
Advanced Generative Adversarial Networks (GANs)
Dieser Kurs ist Teil von Spezialisierung Keras Deep Learning & Generative Adversarial Networks (GAN)

Dozent: Packt - Course Instructors
Bei enthalten
Empfohlene Erfahrung
Was Sie lernen werden
Understand the principles and architecture of GANs
Explain how to implement and train GAN models for image synthesis
Apply techniques to optimize GAN models for improved performance
Evaluate and interpret GAN-generated images
Kompetenzen, die Sie erwerben
- Kategorie: Keras
- Kategorie: Generative Adversarial Networks
- Kategorie: Deep Learning
- Kategorie: TensorFlow
- Kategorie: AI Image Synthesis
Wichtige Details

Zu Ihrem LinkedIn-Profil hinzufügen
September 2024
15 Aufgaben
Erfahren Sie, wie Mitarbeiter führender Unternehmen gefragte Kompetenzen erwerben.
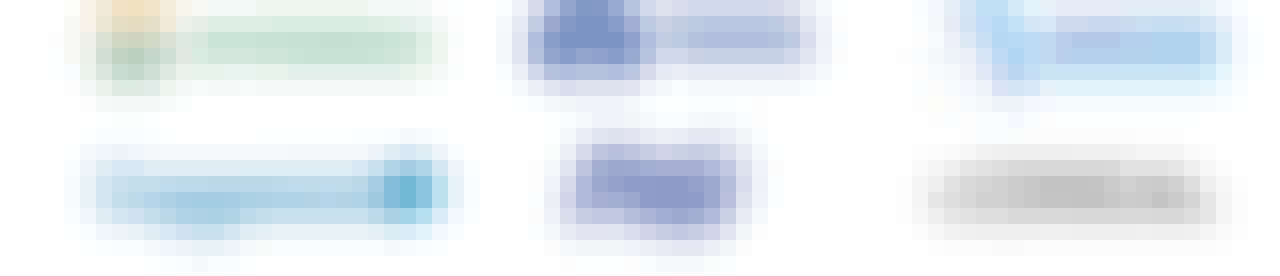
Erweitern Sie Ihre Fachkenntnisse
- Lernen Sie neue Konzepte von Branchenexperten
- Gewinnen Sie ein Grundverständnis bestimmter Themen oder Tools
- Erwerben Sie berufsrelevante Kompetenzen durch praktische Projekte
- Erwerben Sie ein Berufszertifikat zur Vorlage
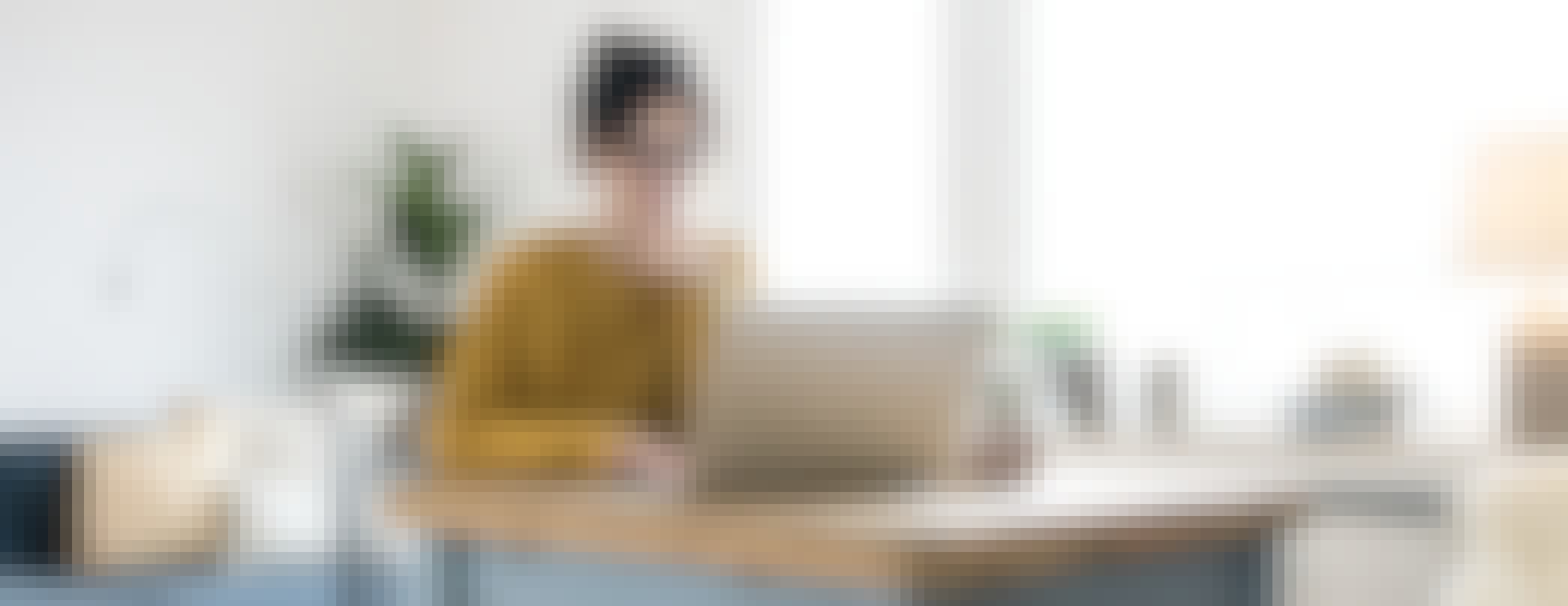
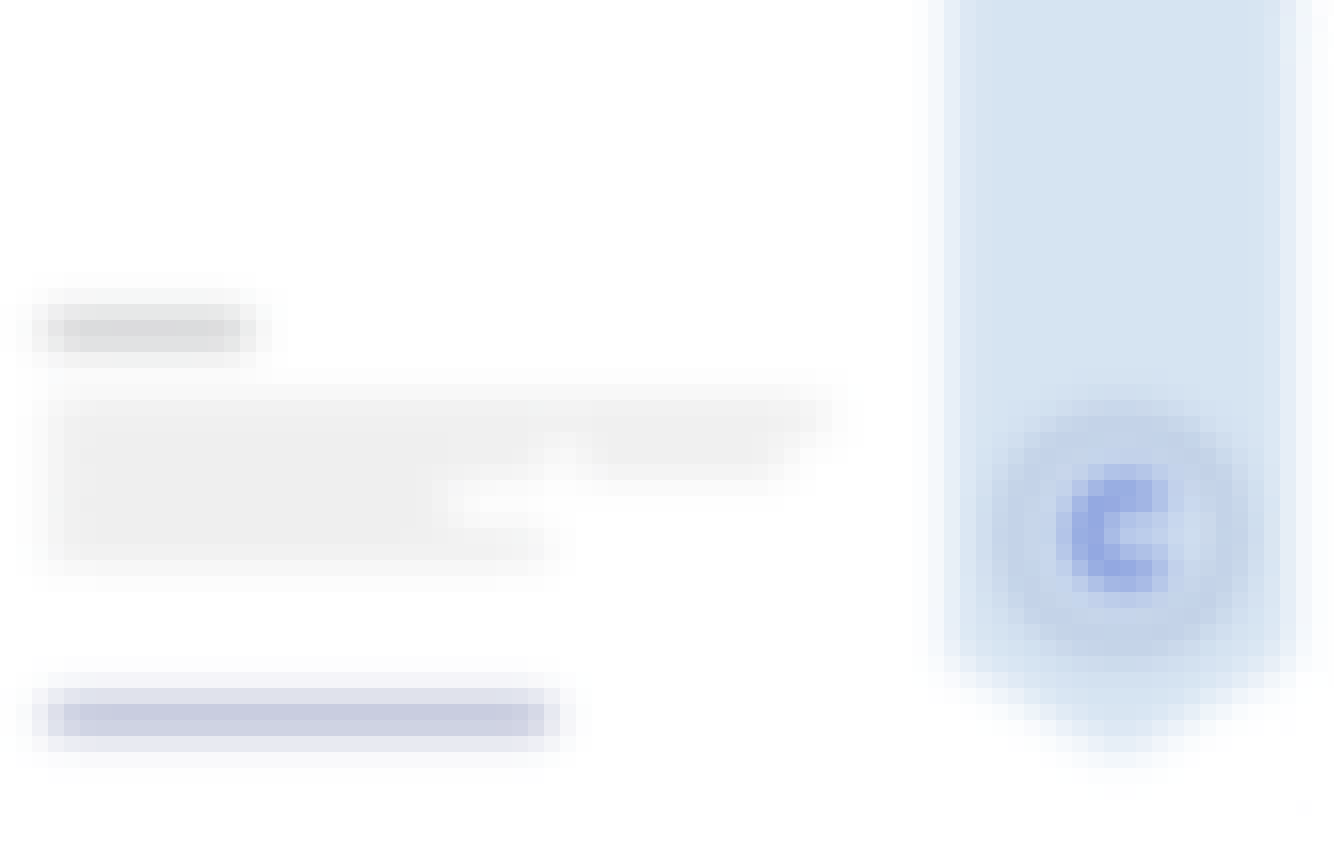
Erwerben Sie ein Karrierezertifikat.
Fügen Sie diese Qualifikation zur Ihrem LinkedIn-Profil oder Ihrem Lebenslauf hinzu.
Teilen Sie es in den sozialen Medien und in Ihrer Leistungsbeurteilung.
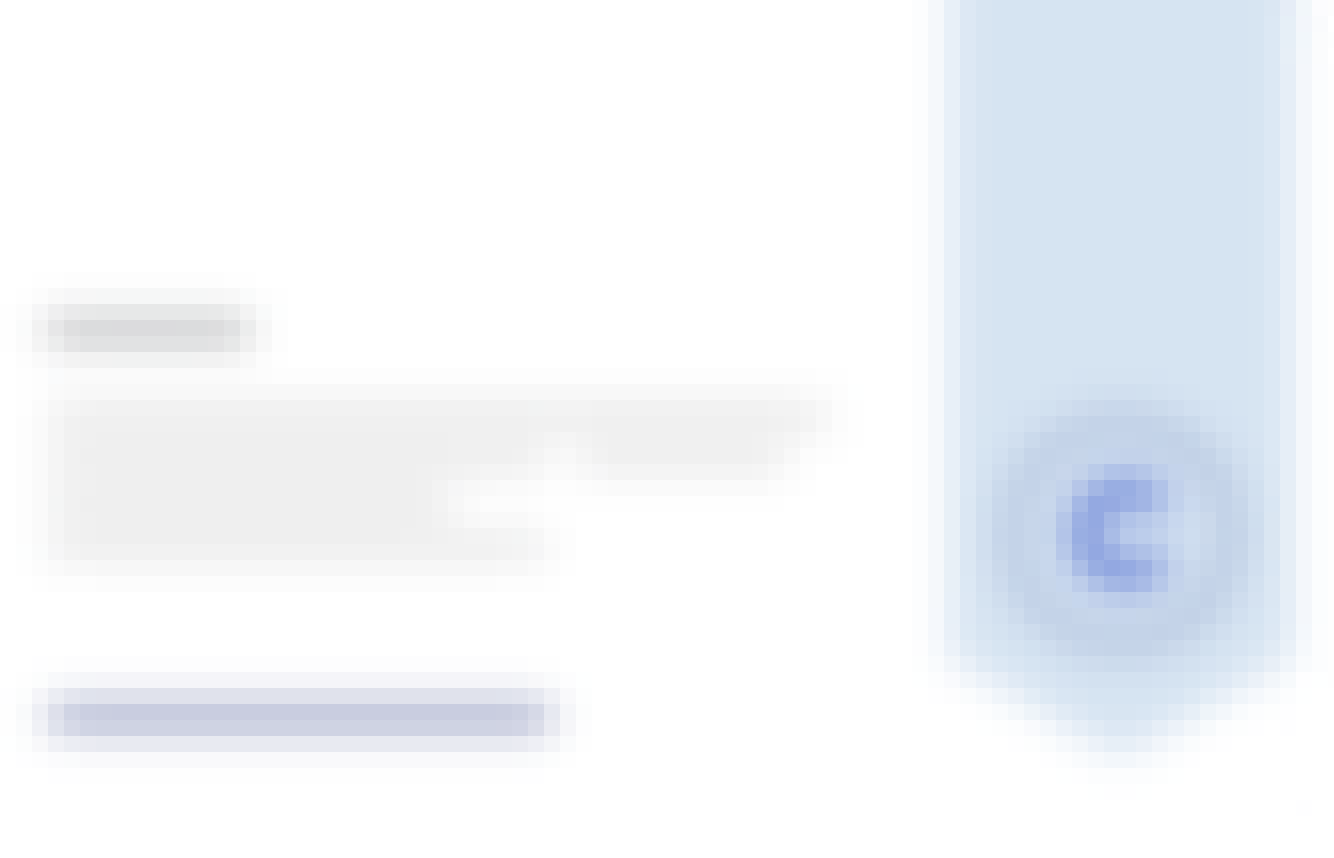
In diesem Kurs gibt es 42 Module
In this module, we will delve into the process of transfer learning using the ResNet50 model on Google Colab's GPU. You will learn step-by-step how to harness the power of pre-trained models for image classification tasks, optimizing performance with Google Colab's robust computing resources.
Das ist alles enthalten
1 Video2 Lektüren
In this module, we will delve into the captivating world of neural networks, exploring some of the most popular and influential types. You will learn about feedforward neural networks, convolutional neural networks (CNNs), and recurrent neural networks (RNNs), understanding their architecture, strengths, and applications.
Das ist alles enthalten
1 Video
In this module, we will embark on a journey into the realm of Generative Adversarial Networks (GANs), an innovative and powerful concept in machine learning and artificial intelligence. You will gain insights into the core components and the groundbreaking applications of GANs.
Das ist alles enthalten
1 Video1 Aufgabe
In this module, we will delve into a practical example of transpose convolution, also known as deconvolution, using a grayscale image. You will learn to assemble and process images using Keras' Sequential class, converting images to NumPy arrays, and performing deconvolution operations.
Das ist alles enthalten
3 Videos
In this module, we will delve into the intricacies of the Generator and Discriminator mechanisms in GANs. You will gain a comprehensive understanding of how these core components operate and interact to enable the functionality of GANs.
Das ist alles enthalten
1 Video
In this module, we will dive into the world of fully connected simple GANs using the MNIST dataset. You will receive a comprehensive introduction to the project setup and the foundational concepts of implementing GANs with the MNIST dataset.
Das ist alles enthalten
1 Video1 Aufgabe
In this module, we will embark on the critical phase of loading the MNIST dataset for our fully connected GAN. You will learn how to prepare and load data, setting the foundation for robust model training.
Das ist alles enthalten
1 Video
In this module, we will explore the process of defining the Generator function for a fully connected GAN. You will learn to structure and organize the project, transitioning from planning to code implementation.
Das ist alles enthalten
2 Videos
In this module, we will define the Discriminator function for our fully connected GAN. You will learn how to create this critical component, completing the foundation of the GAN architecture.
Das ist alles enthalten
2 Videos1 Aufgabe
In this module, we will unveil the pivotal process of combining the Generator and Discriminator models. You will learn to merge these key components, enabling the GAN's image generation capabilities.
Das ist alles enthalten
1 Video
In this module, we will guide you through the process of compiling both the Discriminator and combined GAN models. You will learn to configure these crucial components, bridging the gap between theory and implementation.
Das ist alles enthalten
1 Video
In this module, we will delve into the training process of the Discriminator model. You will learn to construct training loops and optimize the Discriminator, enhancing its ability to distinguish real images from generated ones.
Das ist alles enthalten
3 Videos1 Aufgabe
In this module, we will demystify the process of training the Generator model. You will learn to optimize its parameters, implement backpropagation and gradient descent, and understand the loss functions involved in refining the Generator's abilities.
Das ist alles enthalten
1 Video
In this module, we will guide you through capturing and storing crucial training metrics at specific intervals. You will learn to save logs, creating a detailed record of the GAN's progress for analysis and decision-making.
Das ist alles enthalten
1 Video
In this module, we will guide you through the technique of plotting logs at intervals. You will learn to visualize the progression of the GAN's training, interpreting the plotted metrics to understand and analyze model performance.
Das ist alles enthalten
1 Video1 Aufgabe
In this module, we will focus on displaying generated sample images. You will learn to enhance the graph by visualizing generated images, monitoring the GAN's output quality during iterations, and analyzing the visual progress of the training.
Das ist alles enthalten
2 Videos
In this module, we will navigate the process of saving the trained Generator model. You will learn to preserve the Generator's learned features and weights, enabling future use and continuous generation of new content.
Das ist alles enthalten
1 Video
In this module, we will guide you through the process of generating fake images using a saved GAN model. You will learn to load and utilize the pre-trained model, creating synthetic images and exploring the creative possibilities of GANs.
Das ist alles enthalten
1 Video1 Aufgabe
In this module, we will explore the differences between fully connected GANs and deep convolutional GANs (DCGANs). You will learn to compare their architectures, understand their strengths and applications, and analyze how the choice of GAN type affects image synthesis quality.
Das ist alles enthalten
1 Video
In this module, we will guide you through the steps of preparing and loading the MNIST handwritten digits dataset. You will learn to set the stage for training a DCGAN, exploring the creative potential of this iconic dataset.
Das ist alles enthalten
1 Video
In this module, we will define the Generator function for a deep convolutional GAN. You will learn to adapt previous code for DCGANs and implement the Generator function, laying the groundwork for intricate image generation.
Das ist alles enthalten
2 Videos1 Aufgabe
In this module, we will guide you through defining the Discriminator function for a DCGAN. You will learn to design this critical component, understanding its architecture and principles to improve GAN performance.
Das ist alles enthalten
1 Video
In this module, we will guide you through the process of combining and compiling the DCGAN model. You will learn to merge the Generator and Discriminator components, optimizing the model for intricate and realistic image generation.
Das ist alles enthalten
1 Video
In this module, we will delve into the intricate process of training the DCGAN model. You will learn to implement advanced deep learning techniques, analyzing the results to refine and enhance the image generation process.
Das ist alles enthalten
1 Video1 Aufgabe
In this module, we will explore training the DCGAN model using Google Colab's GPU. You will learn to leverage the robust computing power of Google Colab to optimize and enhance the efficiency and speed of the training process.
Das ist alles enthalten
1 Video
In this module, we will guide you through the steps of preparing and loading the Fashion MNIST dataset. You will learn to set the stage for training a DCGAN, exploring the potential for generating fashion-related images.
Das ist alles enthalten
1 Video
In this module, we will guide you through training the DCGAN model on the Fashion MNIST dataset using Google Colab's GPU. You will learn to implement techniques to optimize model performance, generating high-quality fashion images with the trained model.
Das ist alles enthalten
1 Video1 Aufgabe
In this module, we will explore loading the CIFAR-10 dataset and defining the Generator function. You will learn to prepare and load the dataset, designing the Generator function to enable intricate image generation with DCGANs.
Das ist alles enthalten
2 Videos
In this module, we will dive into defining the Discriminator function for a DCGAN using the CIFAR-10 dataset. You will learn to design and implement a robust Discriminator, enhancing the effectiveness of image classification.
Das ist alles enthalten
1 Video
In this module, we will provide a comprehensive walkthrough of training the DCGAN model on the CIFAR-10 dataset. You will learn to implement deep learning techniques and analyze the results to refine and improve the model.
Das ist alles enthalten
1 Video1 Aufgabe
In this module, we will guide you through training the DCGAN model on the CIFAR-10 dataset using Google Colab's GPU. You will learn to optimize the training process, leveraging robust computing power to generate high-quality images with the trained model.
Das ist alles enthalten
1 Video
In this module, we will explore the fundamental differences between Vanilla GANs and Conditional GANs. You will learn to compare their architectures, understand their training objectives and applications, and explore the unique strengths and purposes of each approach.
Das ist alles enthalten
1 Video
In this module, we will embark on defining the basic Generator function for a Conditional GAN. You will learn the importance of conditional data and implement the Generator function to enable controlled and realistic data generation.
Das ist alles enthalten
1 Video1 Aufgabe
In this module, we will delve into the crucial aspect of embedding labels into generated images. You will learn to enhance the Generator's capability by embedding labels, enabling targeted and contextually relevant image generation.
Das ist alles enthalten
2 Videos
In this module, we will guide you through defining the basic Discriminator function for a Conditional GAN. You will learn to design this foundational component, understanding its role and implementing it for effective image classification.
Das ist alles enthalten
1 Video
In this module, we will explore the technique of enhancing the Discriminator through label embedding. You will learn to boost the discriminative power of the GAN by embedding labels, improving the effectiveness of your GAN-based projects.
Das ist alles enthalten
1 Video1 Aufgabe
In this module, we will focus on combining and compiling the Conditional GAN model. You will learn to merge the Generator and Discriminator components, optimizing the model for generating realistic and controlled data samples.
Das ist alles enthalten
1 Video
In this module, we will progress to the training phase of the Conditional GAN model. You will learn to train both the Generator and Discriminator, implementing advanced techniques and analyzing results to refine and improve model performance.
Das ist alles enthalten
2 Videos
In this module, we will showcase the process of generating and displaying images with the trained Conditional GAN. You will learn to monitor the quality and relevance of generated images, analyzing the visual output to understand the GAN's capabilities.
Das ist alles enthalten
1 Video1 Aufgabe
In this module, we will delve into training the Conditional GAN model on the MNIST dataset using Google Colab's GPU. You will learn to optimize training with robust computing resources, generating high-quality images with the trained model.
Das ist alles enthalten
1 Video
In this module, we will guide you through training the Conditional GAN model on the Fashion MNIST dataset using Google Colab's GPU. You will learn to enhance the training process with powerful computing resources, generating high-quality fashion images.
Das ist alles enthalten
1 Video1 Aufgabe
In this final module, we will explore other popular GANs and their applications. You will gain access to source code links and repositories for further learning, enhancing your understanding and implementation of GANs in various projects.
Das ist alles enthalten
1 Video1 Lektüre1 Aufgabe
Dozent

von
Empfohlen, wenn Sie sich für Machine Learning interessieren
Warum entscheiden sich Menschen für Coursera für ihre Karriere?





Neue Karrieremöglichkeiten mit Coursera Plus
Unbegrenzter Zugang zu über 7.000 erstklassigen Kursen, praktischen Projekten und Zertifikatsprogrammen, die Sie auf den Beruf vorbereiten – alles in Ihrem Abonnement enthalten
Bringen Sie Ihre Karriere mit einem Online-Abschluss voran.
Erwerben Sie einen Abschluss von erstklassigen Universitäten – 100 % online
Schließen Sie sich mehr als 3.400 Unternehmen in aller Welt an, die sich für Coursera for Business entschieden haben.
Schulen Sie Ihre Mitarbeiter*innen, um sich in der digitalen Wirtschaft zu behaupten.
Häufig gestellte Fragen
Yes, you can preview the first video and view the syllabus before you enroll. You must purchase the course to access content not included in the preview.
If you decide to enroll in the course before the session start date, you will have access to all of the lecture videos and readings for the course. You’ll be able to submit assignments once the session starts.
Once you enroll and your session begins, you will have access to all videos and other resources, including reading items and the course discussion forum. You’ll be able to view and submit practice assessments, and complete required graded assignments to earn a grade and a Course Certificate.