Dieser Kurs auf mittlerem Niveau führt in die mathematischen Grundlagen zur Ableitung der Hauptkomponentenanalyse (PCA) ein, einer grundlegenden Technik zur Dimensionalitätsreduktion. Wir behandeln einige grundlegende statistische Daten wie Mittelwerte und Varianzen, berechnen Abstände und Winkel zwischen Vektoren mit Hilfe innerer Produkte und leiten orthogonale Projektionen von Daten auf niedriger dimensionale Unterräume ab. Mit all diesen Werkzeugen werden wir dann die PCA als eine Methode ableiten, die den durchschnittlichen quadratischen Rekonstruktionsfehler zwischen Datenpunkten und ihrer Rekonstruktion minimiert. Am Ende dieses Kurses werden Sie mit wichtigen mathematischen Konzepten vertraut sein und Sie können die PCA ganz alleine implementieren. Wenn Sie Schwierigkeiten haben, finden Sie eine Reihe von Jupyter-Notebooks, mit denen Sie die Eigenschaften der Techniken erkunden können und die Ihnen zeigen, was Sie tun müssen, um auf den richtigen Weg zu kommen. Wenn Sie bereits Experte sind, kann dieser Kurs einige Ihrer Kenntnisse auffrischen. Die Vorlesungen, Beispiele und Übungen erfordern: 1. Eine gewisse Fähigkeit zum abstrakten Denken 2. Gute Kenntnisse in linearer Algebra (z.B. Matrix- und Vektoralgebra, lineare Unabhängigkeit, Basis) 3. Grundkenntnisse in multivariater Kalkulation (z.B. partielle Ableitungen, grundlegende Optimierung) 4. Grundkenntnisse in Python-Programmierung und Numpy Haftungsausschluss: Dieser Kurs ist wesentlich abstrakter und erfordert mehr Programmierkenntnisse als die beiden anderen Kurse der Specialisation. Diese Art von abstraktem Denken, algebraischer Manipulation und Programmierung ist jedoch notwendig, wenn Sie Algorithmen des maschinellen Lernens verstehen und entwickeln möchten.

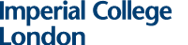
Mathematik für maschinelles Lernen: PCA
Dieser Kurs ist Teil von Spezialisierung Mathematik für maschinelles Lernen
Unterrichtet auf Englisch
Einige Inhalte können nicht übersetzt werden

Dozent: Marc Peter Deisenroth
90.494 bereits angemeldet
Bei enthalten
Kurs
(3,083 Bewertungen)
80%
Was Sie lernen werden
Mathematische Konzepte anhand realer Daten umsetzen
Ableitung der PCA aus einer Projektionsperspektive
Verstehen, wie orthogonale Projektionen funktionieren
Master PCA
Kompetenzen, die Sie erwerben
- Kategorie: Dimensionalitätsreduktion
- Kategorie: Python-Programmierung
- Kategorie: Lineare Algebra
Wichtige Details

Zu Ihrem LinkedIn-Profil hinzufügen
11 Quizzes
Kurs
(3,083 Bewertungen)
80%
Erfahren Sie, wie Mitarbeiter führender Unternehmen gefragte Kompetenzen erwerben.
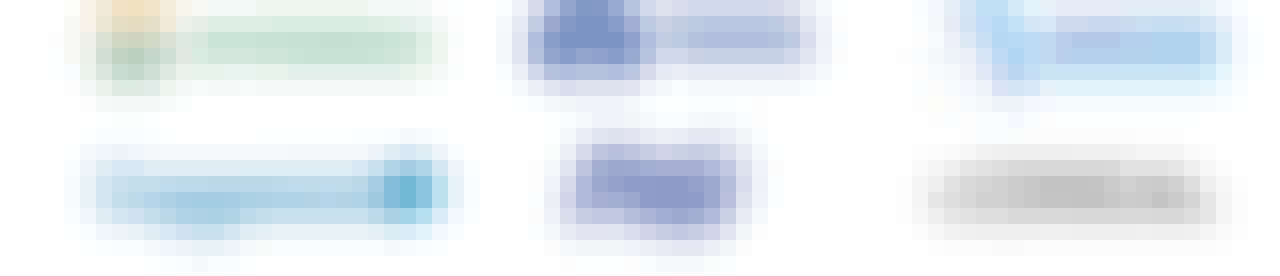
Erweitern Sie Ihre Fachkenntnisse
- Lernen Sie neue Konzepte von Branchenexperten
- Gewinnen Sie ein Grundverständnis bestimmter Themen oder Tools
- Erwerben Sie berufsrelevante Kompetenzen durch praktische Projekte
- Erwerben Sie ein Berufszertifikat zur Vorlage
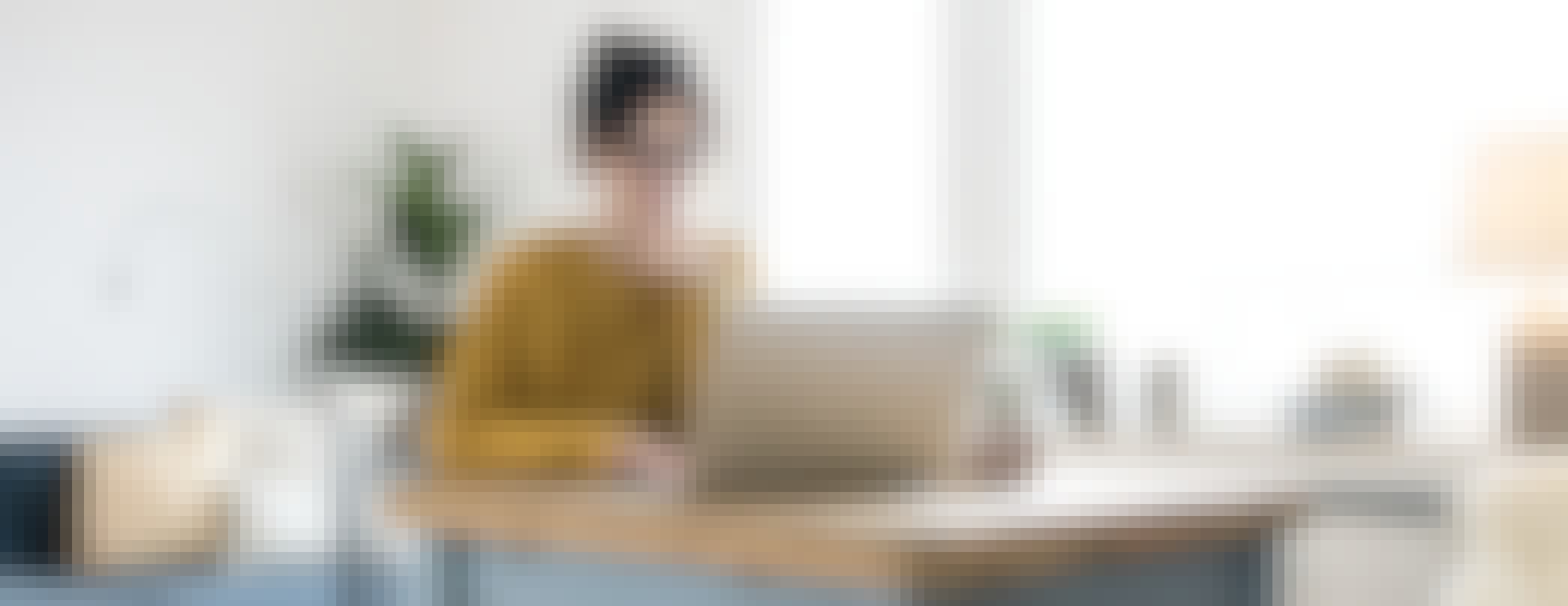
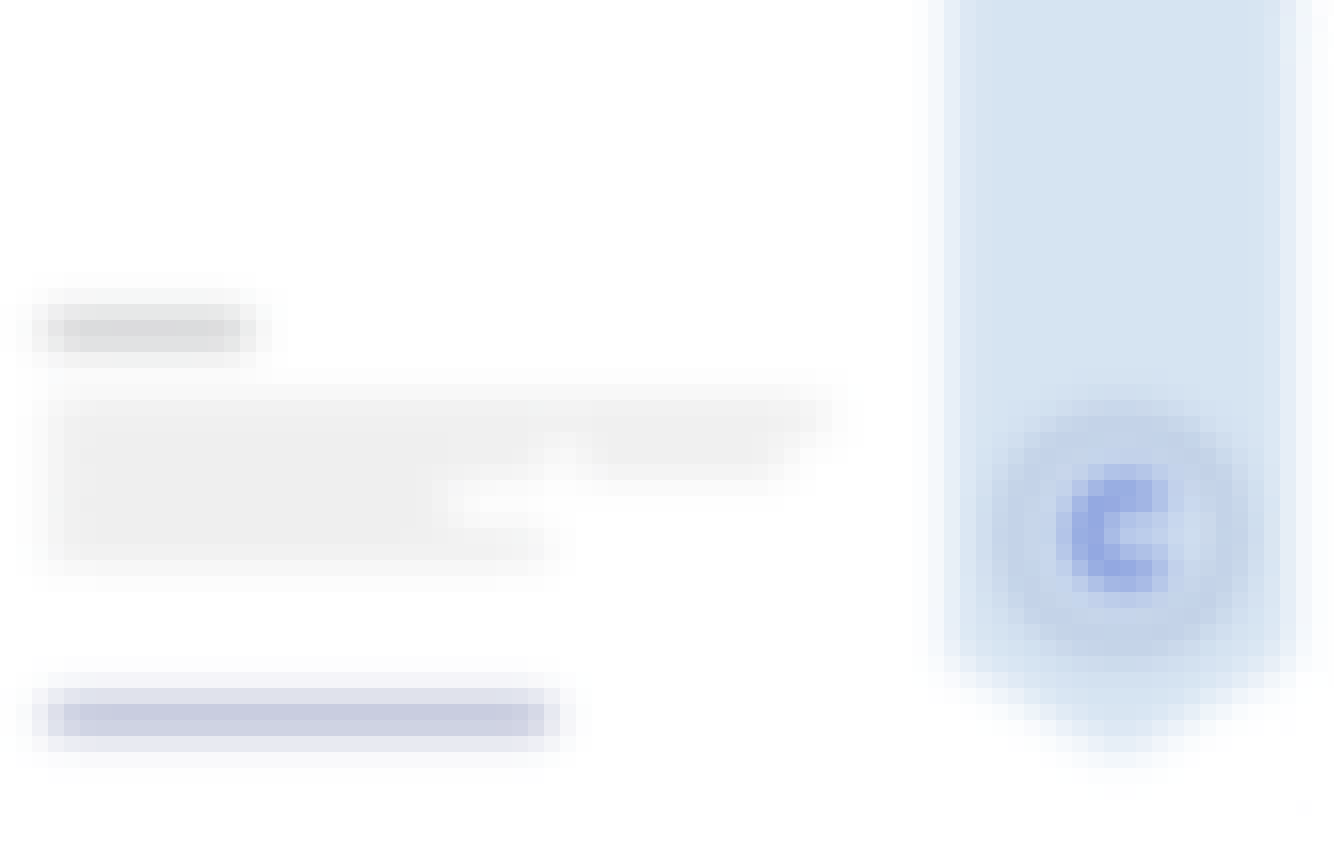
Erwerben Sie ein Karrierezertifikat.
Fügen Sie diese Qualifikation zur Ihrem LinkedIn-Profil oder Ihrem Lebenslauf hinzu.
Teilen Sie es in den sozialen Medien und in Ihrer Leistungsbeurteilung.
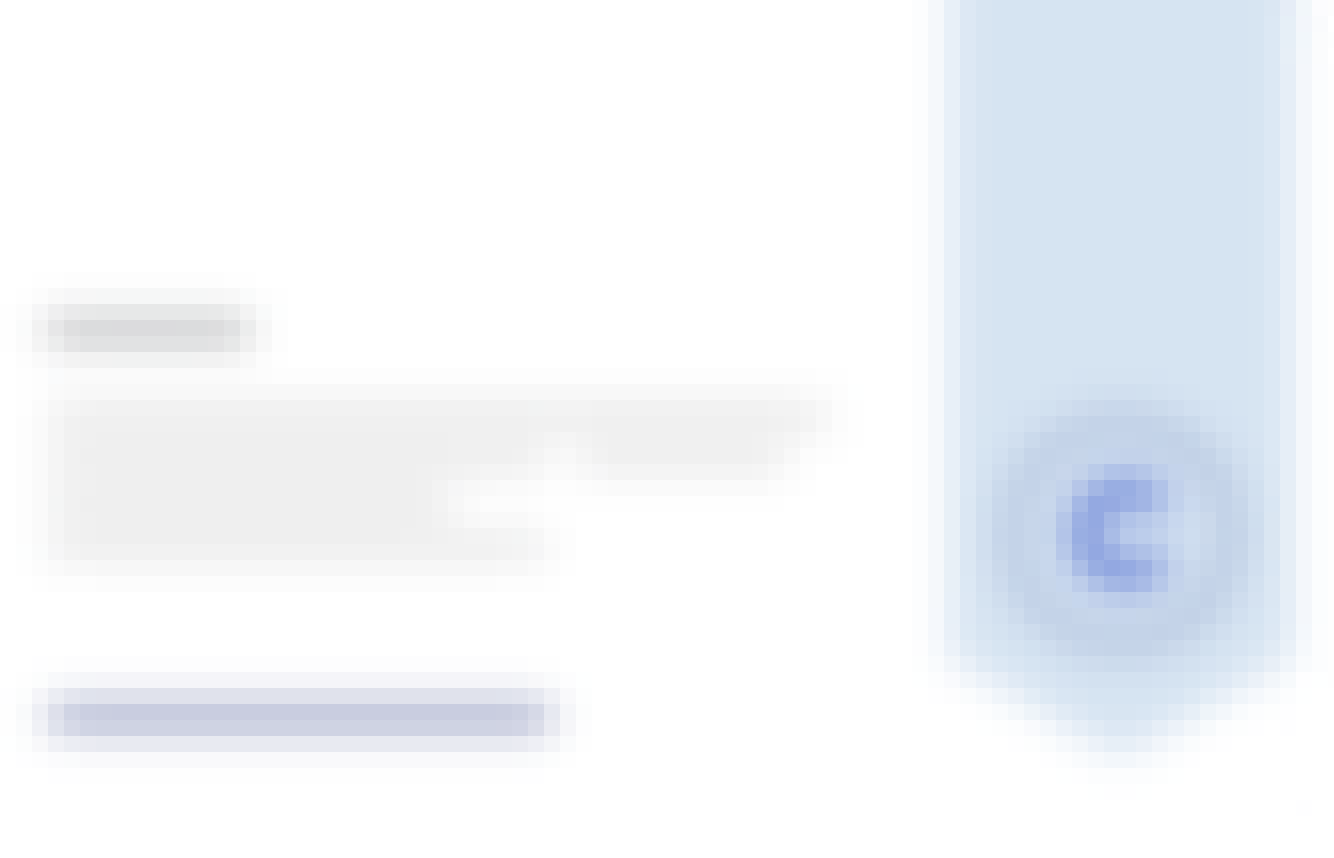
In diesem Kurs gibt es 4 Module
Die Hauptkomponentenanalyse (PCA) ist einer der wichtigsten Algorithmen zur Dimensionalitätsreduktion beim maschinellen Lernen. In diesem Kurs legen wir die mathematischen Grundlagen, um die PCA von einem geometrischen Standpunkt aus abzuleiten und zu verstehen. In diesem Modul lernen wir, wie man Datensätze (z.B. Bilder) mit Hilfe grundlegender Statistiken, wie dem Mittelwert und der Varianz, zusammenfasst. Wir betrachten auch die Eigenschaften von Mittelwert und Varianz, wenn wir den ursprünglichen Datensatz verschieben oder skalieren. Wir vermitteln Ihnen sowohl mathematische Intuition als auch die Fähigkeiten zur Ableitung der Ergebnisse. Wir werden unsere Ergebnisse auch in Code implementieren (Jupyter-Notebooks), so dass wir unser mathematisches Verständnis für die Berechnung von Mittelwerten von Bilddatensätzen üben können. Daher sind einige Python/Numpy-Kenntnisse erforderlich, um diesen Kurs zu bestehen. Hinweis: Wenn Sie die beiden anderen Kurse dieser Specializations belegt haben, wird dieser Kurs schwieriger sein (vor allem wegen der Programmieraufgaben). Wenn Sie jedoch die erste Woche dieses Kurses überstehen, werden Sie mit hoher Wahrscheinlichkeit auch den gesamten Kurs schaffen.
Das ist alles enthalten
8 Videos6 Lektüren3 Quizzes1 Programmieraufgabe1 Diskussionsthema2 Unbewertete Labore1 Plug-in
Daten können als Vektoren interpretiert werden. Vektoren ermöglichen es uns, über geometrische Konzepte wie Längen, Abstände und Winkel zu sprechen, um die Ähnlichkeit zwischen Vektoren zu beschreiben. Dies wird im weiteren Verlauf des Kurses wichtig werden, wenn wir die PCA besprechen. In diesem Modul werden wir das Konzept des inneren Produkts einführen und üben. Innere Produkte ermöglichen es uns, über geometrische Konzepte in Vektorräumen zu sprechen. Genauer gesagt beginnen wir mit dem Punktprodukt (das wir vielleicht noch aus der Schule kennen) als Spezialfall eines inneren Produkts und gehen dann zu einem allgemeineren Konzept eines inneren Produkts über, das in einigen Bereichen des maschinellen Lernens eine wesentliche Rolle spielt, z.B. bei Kernelmaschinen (dazu gehören Support Vector Machines und Gaußsche Prozesse). In diesem Modul gibt es viele Übungen, um das Konzept der inneren Produkte zu üben und zu verstehen.
Das ist alles enthalten
8 Videos1 Lektüre4 Quizzes1 Programmieraufgabe2 Unbewertete Labore
In diesem Modul befassen wir uns mit orthogonalen Projektionen von Vektoren, die sich in einem hochdimensionalen Vektorraum befinden, auf niederdimensionale Unterräume. Dies wird im nächsten Modul eine wichtige Rolle spielen, wenn wir die PCA ableiten. Wir beginnen mit einer geometrischen Begründung, was eine orthogonale Projektion ist und arbeiten uns durch die entsprechende Ableitung. Am Ende werden wir eine einzige Gleichung haben, mit der wir jeden beliebigen Vektor auf einen niederdimensionalen Unterraum projizieren können. Wir werden aber auch verstehen, wie diese Gleichung zustande gekommen ist. Wie in den anderen Modulen werden wir sowohl mit Stift und Papier üben als auch ein kleines Programmierbeispiel mit einem Jupyter-Notebook durchführen.
Das ist alles enthalten
6 Videos1 Lektüre2 Quizzes1 Programmieraufgabe1 Unbewertetes Labor
Wir können uns die Dimensionalitätsreduktion als eine Möglichkeit vorstellen, Daten mit einem gewissen Verlust zu komprimieren, ähnlich wie bei jpg oder mp3. Die Hauptkomponentenanalyse (PCA) ist eine der grundlegendsten Techniken zur Dimensionalitätsreduktion, die beim maschinellen Lernen eingesetzt wird. In diesem Modul verwenden wir die Ergebnisse aus den ersten drei Modulen dieses Kurses und leiten die PCA von einem geometrischen Standpunkt aus ab. Innerhalb dieses Kurses ist dieses Modul das anspruchsvollste. Wir werden eine explizite Herleitung der PCA sowie einige Kodierungsübungen durchführen, die uns zu geübten Anwendern der PCA machen werden.
Das ist alles enthalten
10 Videos5 Lektüren2 Quizzes1 Programmieraufgabe2 Unbewertete Labore1 Plug-in
Dozent

Empfohlen, wenn Sie sich für Maschinelles Lernen interessieren
Imperial College London
Imperial College London
Institut Mines-Télécom
Warum entscheiden sich Menschen für Coursera für ihre Karriere?




Bewertungen von Lernenden
Zeigt 3 von 3083
3.083 Bewertungen
- 5 stars
51,27 %
- 4 stars
22,27 %
- 3 stars
12,70 %
- 2 stars
6,59 %
- 1 star
7,14 %
Geprüft am 6. Juli 2021
Geprüft am 19. Juli 2022
Geprüft am 27. Dez. 2019

Neue Karrieremöglichkeiten mit Coursera Plus
Unbegrenzter Zugang zu über 7.000 erstklassigen Kursen, praktischen Projekten und Zertifikatsprogrammen, die Sie auf den Beruf vorbereiten – alles in Ihrem Abonnement enthalten
Bringen Sie Ihre Karriere mit einem Online-Abschluss voran.
Erwerben Sie einen Abschluss von erstklassigen Universitäten – 100 % online
Schließen Sie sich mehr als 3.400 Unternehmen in aller Welt an, die sich für Coursera for Business entschieden haben.
Schulen Sie Ihre Mitarbeiter*innen, um sich in der digitalen Wirtschaft zu behaupten.
Häufig gestellte Fragen
Sie benötigen gute Python-Kenntnisse, um den Kurs zu absolvieren.
Dieser Kurs ist deutlich schwieriger und hat einen anderen Stil: Er verwendet abstraktere Konzepte und erfordert viel mehr Programmiererfahrung als die beiden anderen Kurse. Wenn Sie die vollständige Spezialisierung abschließen, werden Sie daher mit einem viel vielfältigeren Set an Fähigkeiten ausgestattet sein.
Der Zugang zu Vorlesungen und Aufgaben hängt von der Art Ihrer Einschreibung ab. Wenn Sie einen Kurs im Prüfungsmodus belegen, können Sie die meisten Kursmaterialien kostenlos einsehen. Um auf benotete Aufgaben zuzugreifen und ein Zertifikat zu erwerben, müssen Sie die Zertifikatserfahrung während oder nach Ihrer Prüfung erwerben. Wenn Sie die Prüfungsoption nicht sehen:
Der Kurs bietet möglicherweise keine Prüfungsoption. Sie können stattdessen eine kostenlose Testversion ausprobieren oder finanzielle Unterstützung beantragen.
Der Kurs bietet möglicherweise stattdessen die Option 'Vollständiger Kurs, kein Zertifikat'. Mit dieser Option können Sie alle Kursmaterialien einsehen, die erforderlichen Bewertungen abgeben und eine Abschlussnote erhalten. Dies bedeutet auch, dass Sie kein Zertifikat erwerben können.