Ce cours propose une brève introduction au calcul à plusieurs variables nécessaire à la construction de nombreuses techniques courantes d'apprentissage automatique. Nous commençons au tout début par un rappel de la formulation "rise over run" d'une pente, avant de la convertir en la définition formelle du gradient d'une fonction. Nous commençons ensuite à construire un ensemble d'outils pour rendre le calcul plus facile et plus rapide. Ensuite, nous apprenons à calculer des vecteurs qui pointent vers le haut d'une colline sur des surfaces multidimensionnelles et nous mettons même cela en pratique à l'aide d'un jeu interactif. Nous examinons comment nous pouvons utiliser le calcul pour construire des approximations de fonctions, ainsi que pour nous aider à quantifier la précision que nous pouvons attendre de ces approximations. Nous passerons également un peu de temps à parler de l'utilisation du calcul dans l'entraînement des réseaux neuronaux, avant de vous montrer comment il est appliqué dans les modèles de régression linéaire. Ce cours a pour but d'offrir une compréhension intuitive du calcul, ainsi que le langage nécessaire pour rechercher des concepts par vous-même lorsque vous êtes bloqué. Nous espérons que, sans entrer dans les détails, vous repartirez avec la confiance nécessaire pour vous plonger dans des cours d'apprentissage automatique plus ciblés à l'avenir.

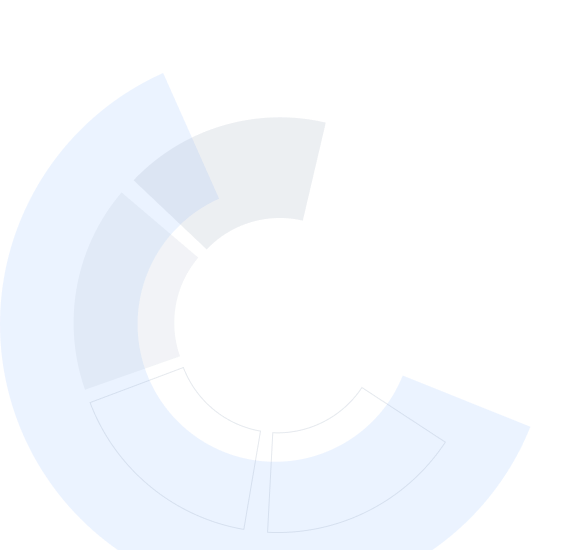
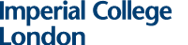
Mathématiques pour l'apprentissage automatique : Calcul à plusieurs variables
Ce cours fait partie de Spécialisation Mathématiques pour l'apprentissage automatique



Instructeurs : Samuel J. Cooper
144 000 déjà inscrits
Inclus avec
(5,647 avis)
Compétences que vous acquerrez
- Catégorie : Régression linéaire
- Catégorie : Calcul vectoriel
- Catégorie : Calcul à plusieurs variables
- Catégorie : Descente de gradient
Détails à connaître

Ajouter à votre profil LinkedIn
25 devoirs
Découvrez comment les employés des entreprises prestigieuses maîtrisent des compétences recherchées
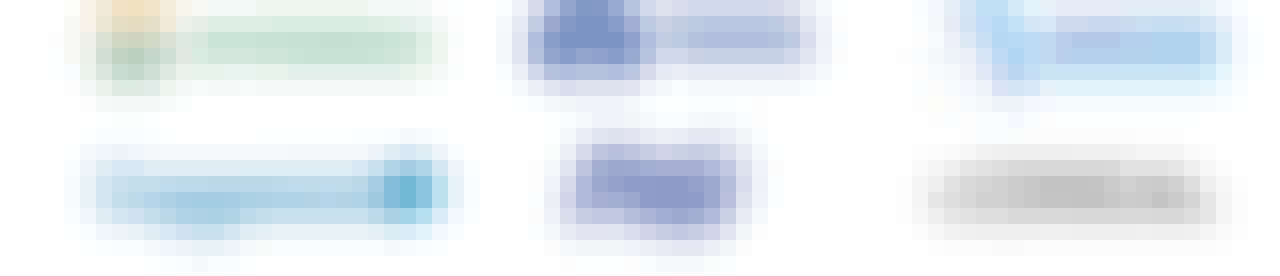
Élaborez votre expertise du sujet
- Apprenez de nouveaux concepts auprès d'experts du secteur
- Acquérez une compréhension de base d'un sujet ou d'un outil
- Développez des compétences professionnelles avec des projets pratiques
- Obtenez un certificat professionnel partageable
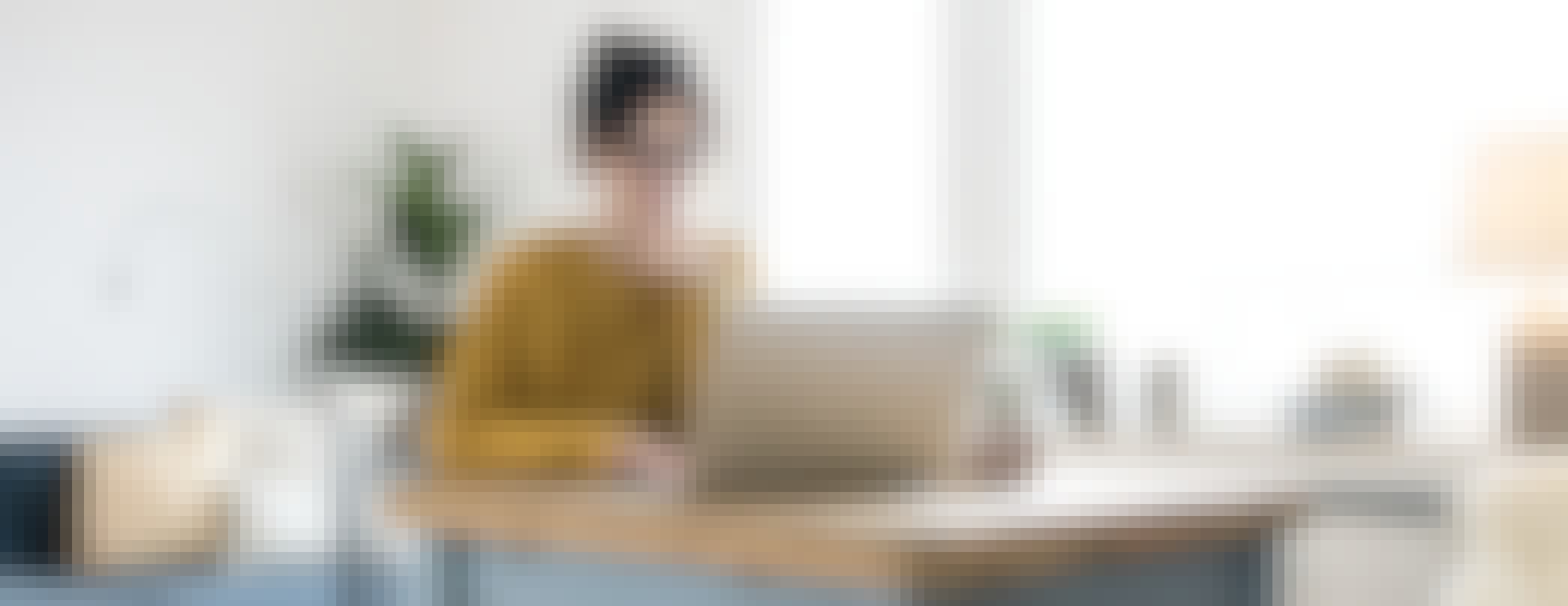
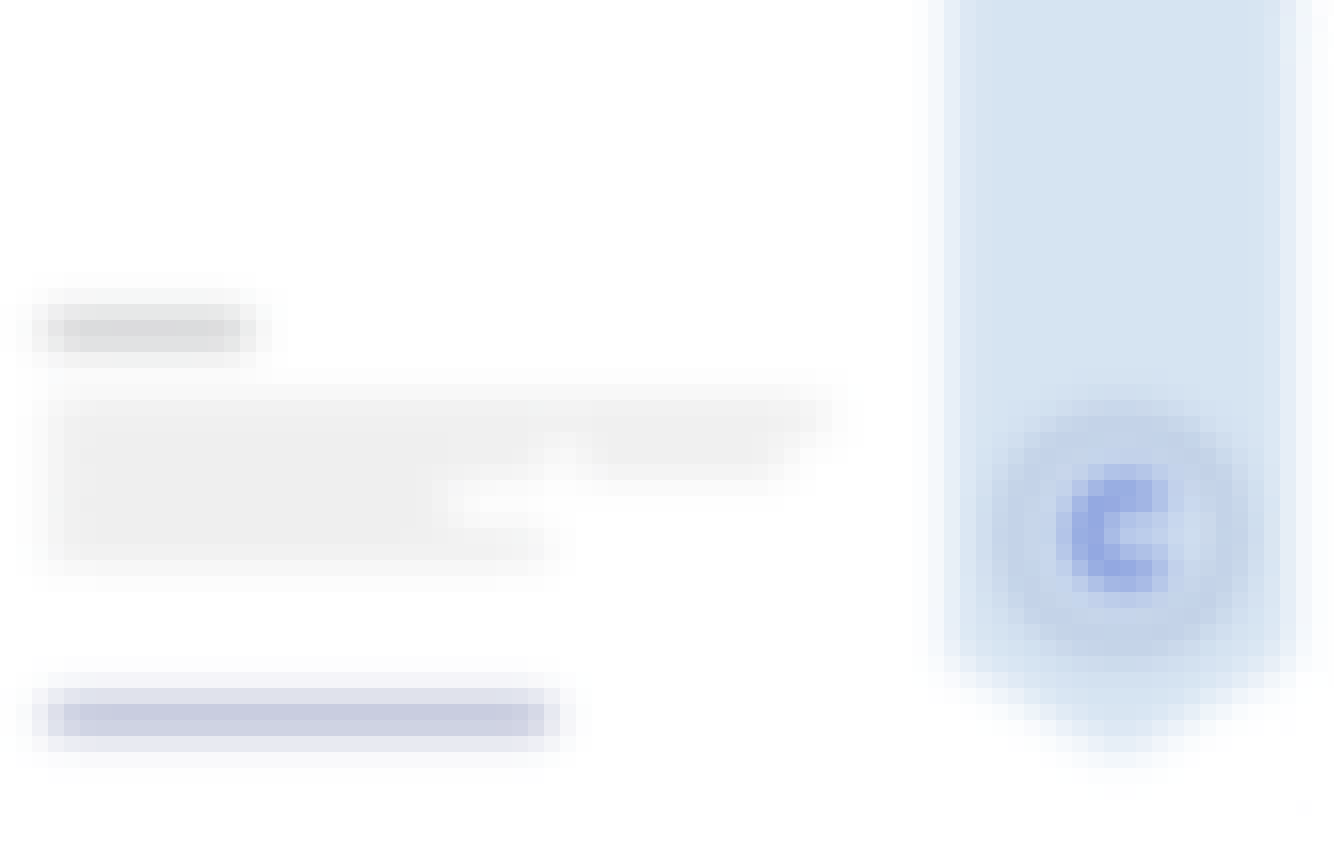
Obtenez un certificat professionnel
Ajoutez cette qualification à votre profil LinkedIn ou à votre CV
Partagez-le sur les réseaux sociaux et dans votre évaluation de performance
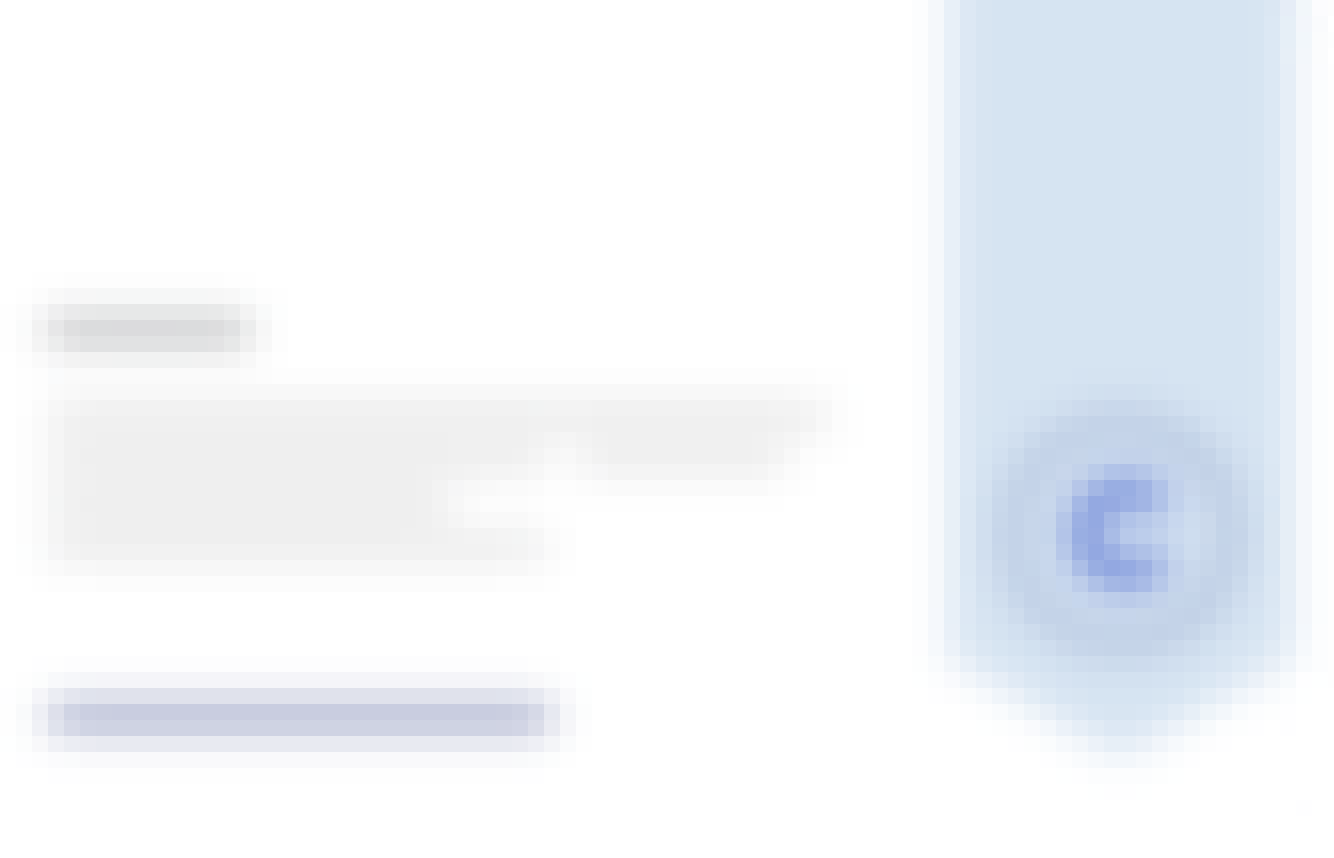
Il y a 6 modules dans ce cours
Comprendre le calcul est essentiel pour comprendre l'apprentissage automatique ! Vous pouvez considérer le calcul comme un simple ensemble d'outils permettant d'analyser la relation entre les fonctions et leurs entrées. Souvent, dans l'apprentissage automatique, nous essayons de trouver les entrées qui permettent à une fonction de correspondre au mieux aux données. Nous commençons ce module par les bases, en rappelant ce qu'est une fonction et où nous pouvons en rencontrer une. Ensuite, nous expliquons comment, lorsque l'on dessine une fonction sur un graphique, la pente décrit le taux de changement de la sortie par rapport à une entrée. En utilisant cette intuition visuelle, nous déduisons ensuite une définition mathématique robuste de la dérivée, que nous utilisons ensuite pour différencier quelques fonctions intéressantes. Enfin, en étudiant quelques exemples, nous développons quatre règles pratiques permettant de gagner du temps et d'accélérer la différenciation pour de nombreux scénarios courants.
Inclus
10 vidéos4 lectures6 devoirs1 sujet de discussion1 plugin
En nous appuyant sur les bases du module précédent, nous généralisons maintenant nos outils de calcul pour traiter les systèmes multivariables. Cela signifie que nous pouvons prendre une fonction avec plusieurs entrées et déterminer l'influence de chacune d'entre elles séparément. Il n'est pas rare qu'une méthode d'apprentissage automatique nécessite l'analyse d'une fonction avec des milliers d'entrées. Nous introduirons donc également les structures d'algèbre linéaire nécessaires pour stocker les résultats de notre analyse de calcul à plusieurs variables de manière ordonnée.
Inclus
9 vidéos5 devoirs2 laboratoires non notés
Après avoir constaté que le calcul à plusieurs variables n'est pas plus compliqué que le calcul à une variable, nous allons maintenant nous concentrer sur les applications de la règle de la chaîne. Les réseaux neuronaux sont l'une des structures conceptuelles les plus populaires et les plus réussies dans le domaine de l'apprentissage automatique. Ils sont construits à partir d'un réseau de neurones connectés et s'inspirent de la structure des cerveaux biologiques. Le comportement de chaque neurone est influencé par un ensemble de paramètres de contrôle, dont chacun doit être optimisé pour s'adapter au mieux aux données. La règle de la chaîne multivariée peut être utilisée pour calculer l'influence de chaque paramètre des réseaux, ce qui permet de les mettre à jour pendant la formation.
Inclus
6 vidéos3 devoirs1 devoir de programmation1 sujet de discussion1 laboratoire non noté
La série de Taylor est une méthode permettant de réexprimer les fonctions sous forme de séries polynomiales. Cette approche est à la base de l'utilisation d'approximations linéaires simples pour des fonctions compliquées. Dans ce module, nous déduirons l'expression formelle de la série de Taylor univariée et discuterons de certaines conséquences importantes de ce résultat pour l'apprentissage automatique. Enfin, nous aborderons le cas multivarié et verrons comment le jacobien et le hessien entrent en jeu.
Inclus
9 vidéos5 devoirs1 plugin
Si nous voulons trouver les points minimum et maximum d'une fonction, nous pouvons utiliser le calcul à plusieurs variables pour y parvenir, par exemple pour optimiser les paramètres (l'espace) d'une fonction afin de l'adapter à certaines données. Nous commencerons par le faire en une dimension et utiliserons le gradient pour estimer où se trouvent les points zéro de cette fonction, puis nous itérerons avec la méthode de Newton-Raphson. Nous étendrons ensuite l'idée à plusieurs dimensions en trouvant le vecteur du gradient, Grad, qui est le vecteur du jacobien. Cela nous permettra de trouver notre chemin vers les minima et les maxima dans ce que l'on appelle la méthode de descente du gradient. Nous utiliserons ensuite Grad pour trouver les minima et maxima le long d'une contrainte dans l'espace, ce qui est la méthode des multiplicateurs de Lagrange.
Inclus
4 vidéos4 devoirs1 sujet de discussion1 laboratoire non noté
Afin d'optimiser les paramètres d'ajustement d'une fonction d'ajustement pour obtenir le meilleur ajustement possible pour certaines données, nous avons besoin d'un moyen de définir la qualité de notre ajustement. Cette qualité d'ajustement s'appelle le chi-deux, que nous appliquerons d'abord à l'ajustement d'une ligne droite (régression linéaire). Nous verrons ensuite comment optimiser notre fonction d'ajustement à l'aide du chi-deux dans le cas général en utilisant la méthode de descente du gradient. Enfin, nous verrons comment faire cela facilement en Python en quelques lignes de code, ce qui clôturera le cours.
Inclus
4 vidéos1 lecture2 devoirs1 devoir de programmation1 laboratoire non noté1 plugin
Instructeurs



Offert par
Recommandé si vous êtes intéressé(e) par Mathématiques et logique
Alberta Machine Intelligence Institute
Johns Hopkins University
University of Michigan
Pour quelles raisons les étudiants sur Coursera nous choisissent-ils pour leur carrière ?




Avis des étudiants
5 647 avis
- 5 stars
77,08 %
- 4 stars
18,80 %
- 3 stars
3,11 %
- 2 stars
0,63 %
- 1 star
0,35 %
Affichage de 3 sur 5647
Révisé le 20 juil. 2021
Multivariate Calculus course has been by far one of the best moocs I have taken in relation to learn maths for ml, its foundations helped a lot to understand how everything relates from bottom up.
Révisé le 3 août 2019
Very Well Explained. Good content and great explanation of content. Complex topics are also covered in very easy way. Very Helpful for learning much more complex topics for Machine Learning in future.
Révisé le 25 mars 2022
This calculus course covering realy great topic. expecially with great example but also easy to understand this is the easely this is the best explanation calculus i've ever learn so far

Ouvrez de nouvelles portes avec Coursera Plus
Accès illimité à 10,000+ cours de niveau international, projets pratiques et programmes de certification prêts à l'emploi - tous inclus dans votre abonnement.
Faites progresser votre carrière avec un diplôme en ligne
Obtenez un diplôme auprès d’universités de renommée mondiale - 100 % en ligne
Rejoignez plus de 3 400 entreprises mondiales qui ont choisi Coursera pour les affaires
Améliorez les compétences de vos employés pour exceller dans l’économie numérique
Foire Aux Questions
L'accès aux cours et aux devoirs dépend de votre type d'inscription. Si vous suivez un cours en mode audit, vous pourrez consulter gratuitement la plupart des supports de cours. Pour accéder aux devoirs notés et obtenir un certificat, vous devrez acheter l'expérience de certificat, pendant ou après votre audit. Si vous ne voyez pas l'option d'audit :
Il se peut que le cours ne propose pas d'option d'audit. Vous pouvez essayer un essai gratuit ou demander une aide financière.
Le cours peut proposer l'option "Cours complet, pas de certificat" à la place. Cette option vous permet de consulter tous les supports de cours, de soumettre les évaluations requises et d'obtenir une note finale. Cela signifie également que vous ne pourrez pas acheter un certificat d'expérience.
Lorsque vous vous inscrivez au cours, vous avez accès à tous les cours de la Specializations, et vous obtenez un certificat lorsque vous terminez le travail. Votre certificat électronique sera ajouté à votre page de réalisations - de là, vous pouvez imprimer votre certificat ou l'ajouter à votre profil LinkedIn. Si vous souhaitez uniquement lire et visualiser le contenu du cours, vous pouvez auditer le cours gratuitement.
Si vous vous êtes abonné, vous bénéficiez d'une période d'essai gratuite de 7 jours pendant laquelle vous pouvez annuler votre abonnement sans pénalité. Après cette période, nous ne remboursons pas, mais vous pouvez résilier votre abonnement à tout moment. Consultez notre politique de remboursement complète.