This course introduces you to one of the main types of modelling families of supervised Machine Learning: Regression. You will learn how to train regression models to predict continuous outcomes and how to use error metrics to compare across different models. This course also walks you through best practices, including train and test splits, and regularization techniques.

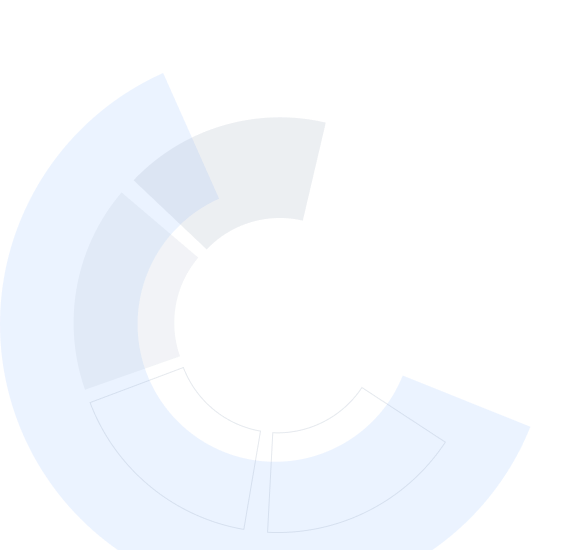
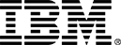
Supervised Machine Learning: Regression
This course is part of multiple programs.



Instructors: Mark J Grover
Sponsored by Barbados NTI
56,497 already enrolled
(651 reviews)
Skills you'll gain
Details to know

Add to your LinkedIn profile
13 assignments
See how employees at top companies are mastering in-demand skills
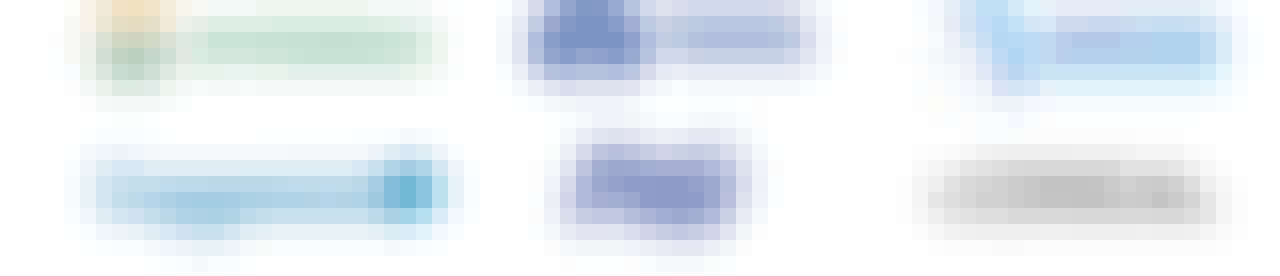
Build your subject-matter expertise
- Learn new concepts from industry experts
- Gain a foundational understanding of a subject or tool
- Develop job-relevant skills with hands-on projects
- Earn a shareable career certificate
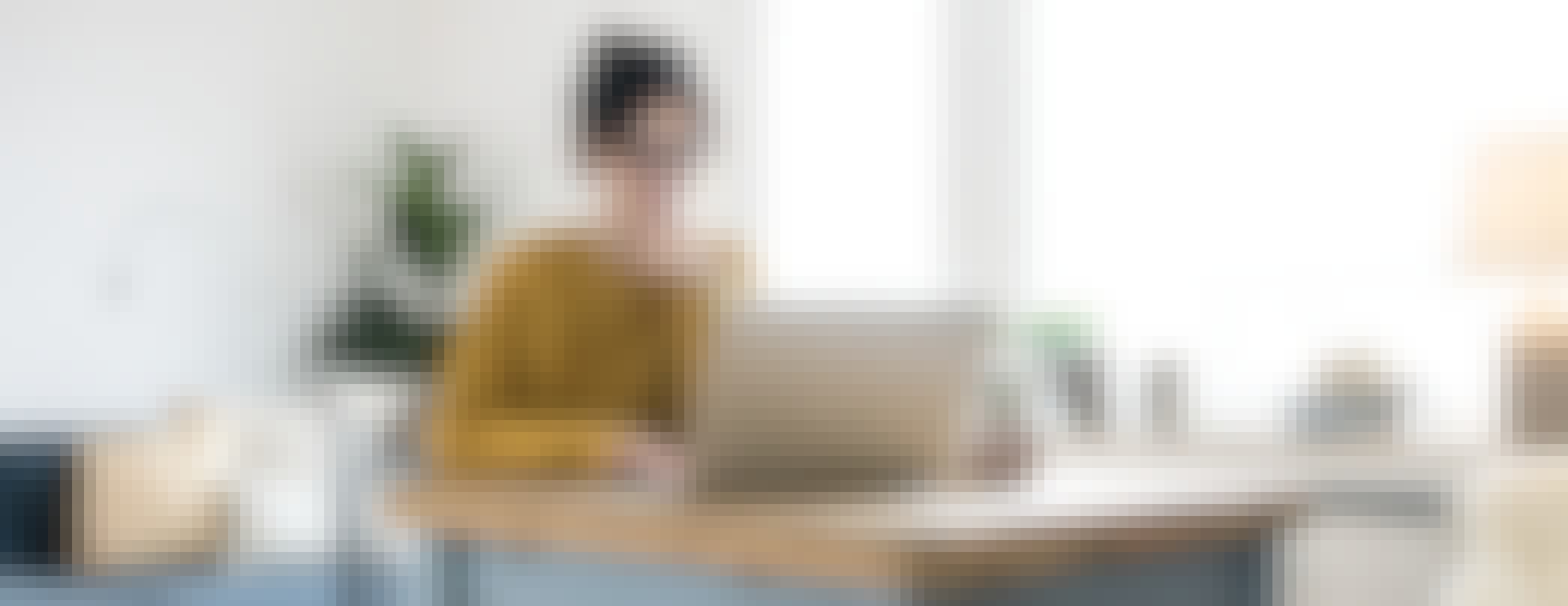
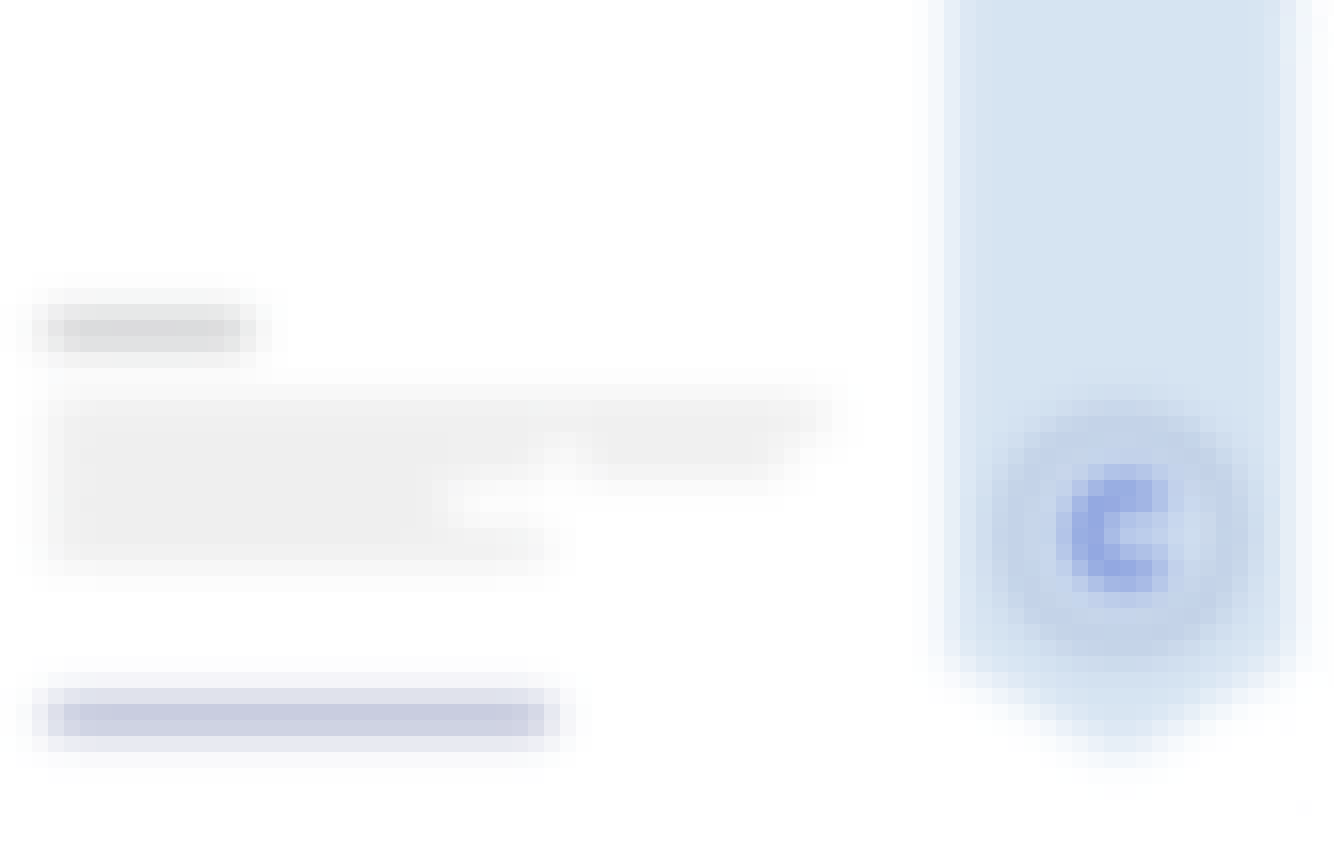
Earn a career certificate
Add this credential to your LinkedIn profile, resume, or CV
Share it on social media and in your performance review
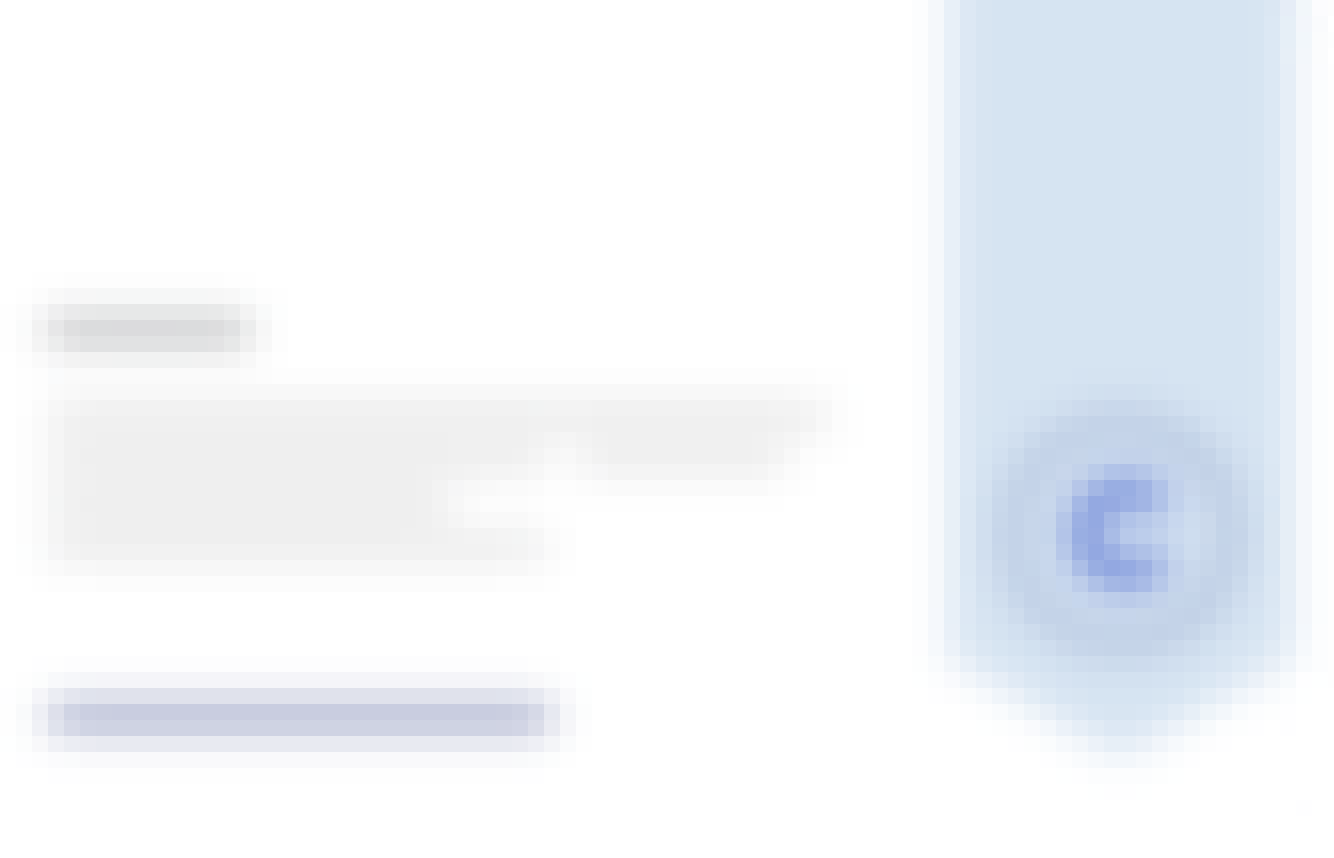
There are 6 modules in this course
This module introduces a brief overview of supervised machine learning and its main applications: classification and regression. After introducing the concept of regression, you will learn its best practices, as well as how to measure error and select the regression model that best suits your data.
What's included
11 videos2 readings3 assignments2 app items
There are a few best practices to avoid overfitting of your regression models. One of these best practices is splitting your data into training and test sets. Another alternative is to use cross validation. And a third alternative is to introduce polynomial features. This module walks you through the theoretical framework and a few hands-on examples of these best practices.
What's included
7 videos1 reading3 assignments2 app items
There is a trade-off between the size of your training set and your testing set. If you use most of your data for training, you will have fewer samples to validate your model. Conversely, if you use more samples for testing, you will have fewer samples to train your model. Cross Validation will allow you to reuse your data to use more samples for training and testing.
What's included
6 videos1 reading2 assignments2 app items1 plugin
This module walks you through the theory and a few hands-on examples of regularization regressions including ridge, LASSO, and elastic net. You will realize the main pros and cons of these techniques, as well as their differences and similarities.
What's included
10 videos1 reading3 assignments1 app item
In this section, you will understand the relationship between the loss function and the different regularization types.
What's included
5 videos1 reading2 assignments2 app items
In this section you will test everything you learned
What's included
1 reading1 peer review1 app item
Instructors



Offered by
Why people choose Coursera for their career




Learner reviews
651 reviews
- 5 stars
76.45%
- 4 stars
17.58%
- 3 stars
3.82%
- 2 stars
0.76%
- 1 star
1.37%
Showing 3 of 651
Reviewed on Aug 10, 2021
Well structured course. Concepts are explained clearly with hands on exercises.
Reviewed on Feb 6, 2022
really good course, content is rich with good machine learning concepts
Reviewed on Apr 9, 2021
Very well presented. This is without doubt the best series for Machine Learning on Coursera.
Recommended if you're interested in Data Science
Duke University
University of California San Diego
New York University

Open new doors with Coursera Plus
Unlimited access to 10,000+ world-class courses, hands-on projects, and job-ready certificate programs - all included in your subscription
Advance your career with an online degree
Earn a degree from world-class universities - 100% online
Join over 3,400 global companies that choose Coursera for Business
Upskill your employees to excel in the digital economy