This course will introduce the concepts of interpretability and explainability in machine learning applications. The learner will understand the difference between global, local, model-agnostic and model-specific explanations. State-of-the-art explainability methods such as Permutation Feature Importance (PFI), Local Interpretable Model-agnostic Explanations (LIME) and SHapley Additive exPlanation (SHAP) are explained and applied in time-series classification. Subsequently, model-specific explanations such as Class-Activation Mapping (CAM) and Gradient-Weighted CAM are explained and implemented. The learners will understand axiomatic attributions and why they are important. Finally, attention mechanisms are going to be incorporated after Recurrent Layers and the attention weights will be visualised to produce local explanations of the model.

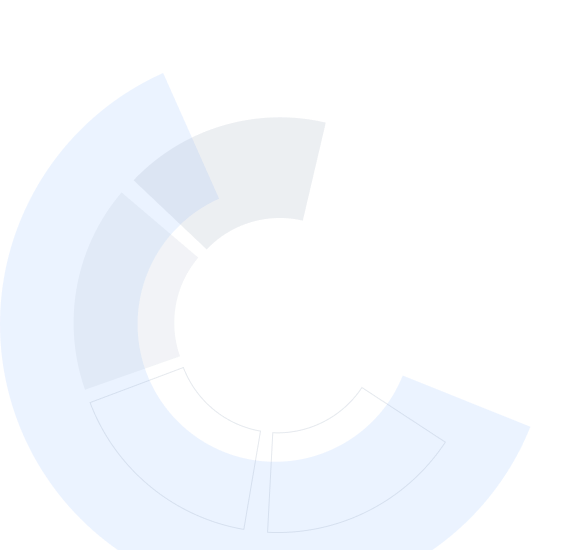
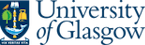
Explainable deep learning models for healthcare - CDSS 3
This course is part of Informed Clinical Decision Making using Deep Learning Specialization

Instructor: Fani Deligianni
Sponsored by PTT Global Chemical
1,673 already enrolled
(15 reviews)
Recommended experience
What you'll learn
Program global explainability methods in time-series classification
Program local explainability methods for deep learning such as CAM and GRAD-CAM
Understand axiomatic attributions for deep learning networks
Incorporate attention in Recurrent Neural Networks and visualise the attention weights
Details to know

Add to your LinkedIn profile
5 assignments
See how employees at top companies are mastering in-demand skills
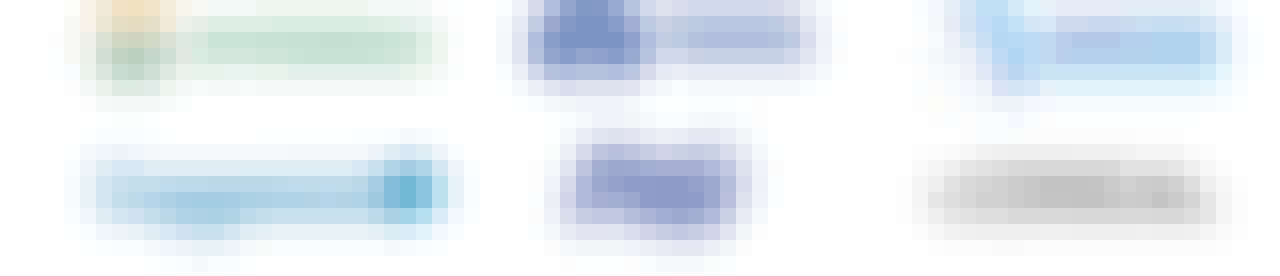
Build your subject-matter expertise
- Learn new concepts from industry experts
- Gain a foundational understanding of a subject or tool
- Develop job-relevant skills with hands-on projects
- Earn a shareable career certificate
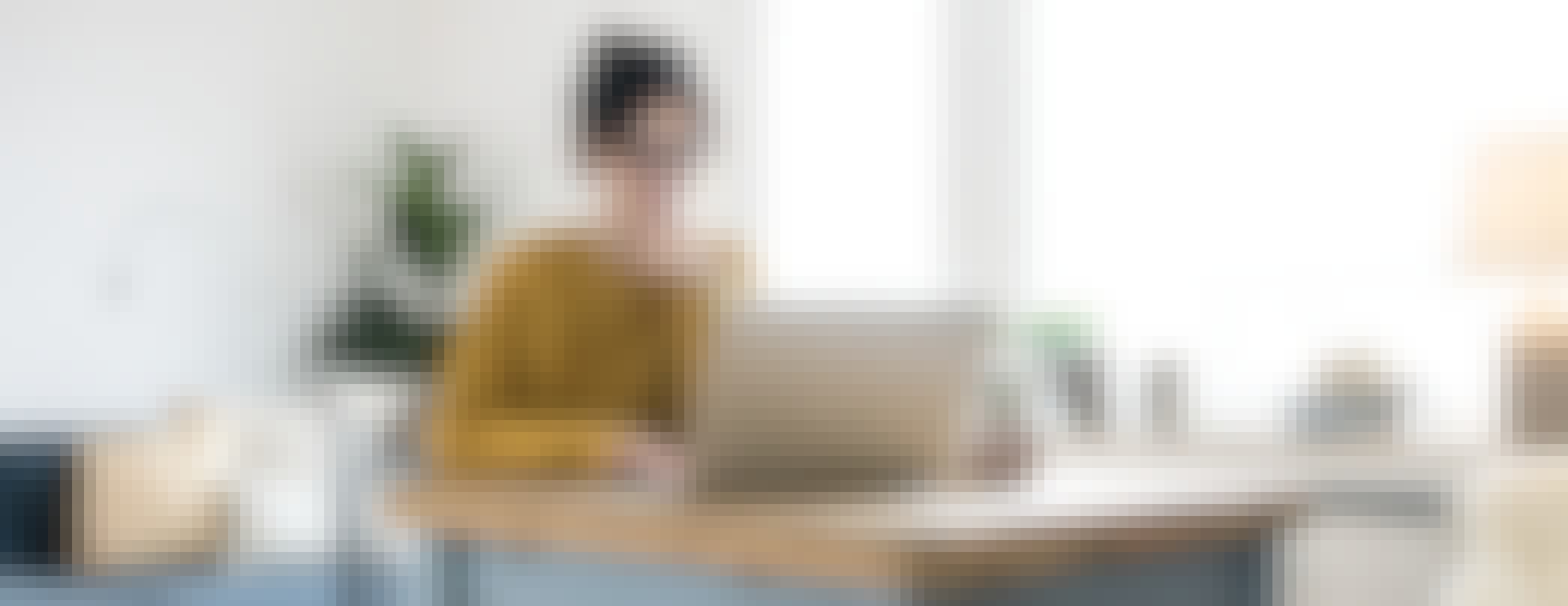
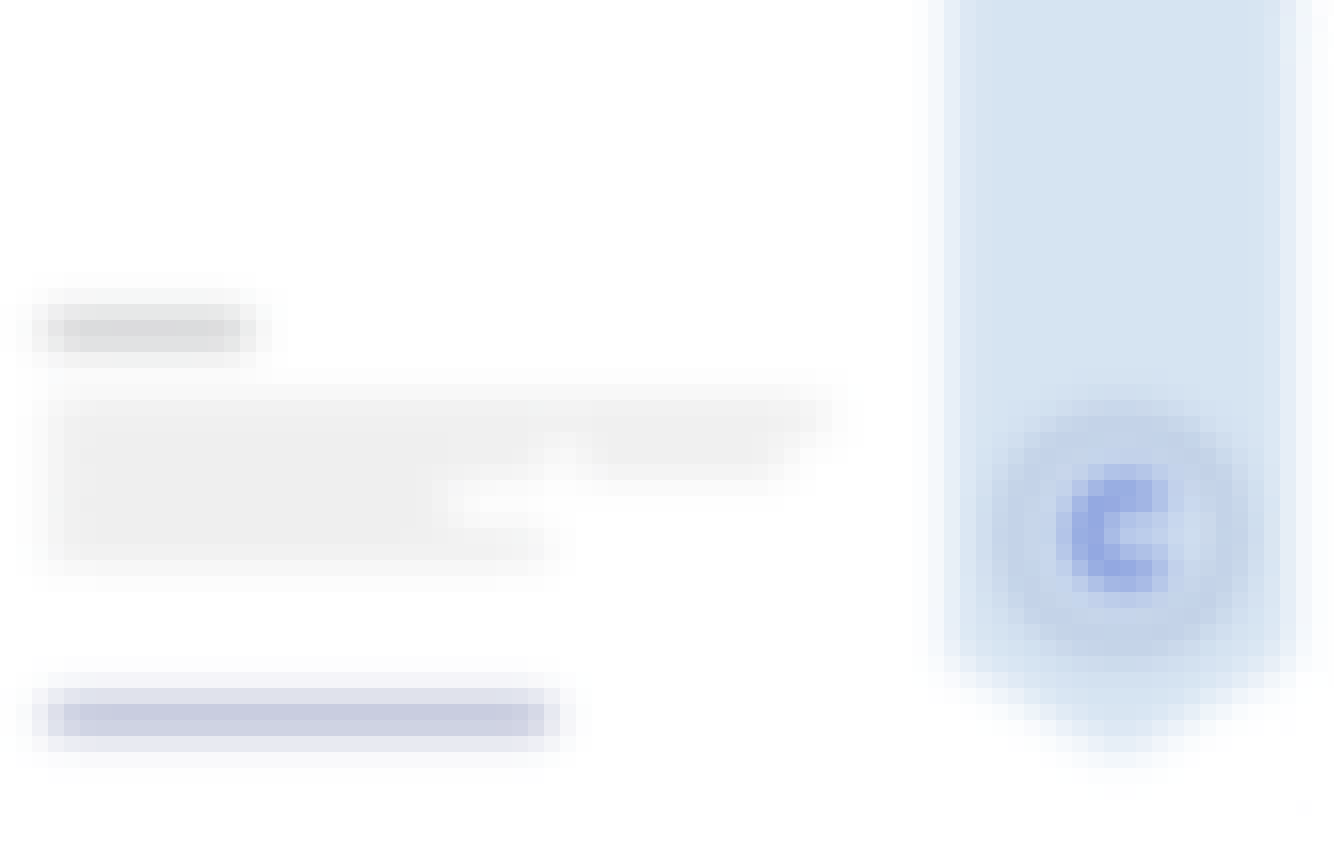
Earn a career certificate
Add this credential to your LinkedIn profile, resume, or CV
Share it on social media and in your performance review
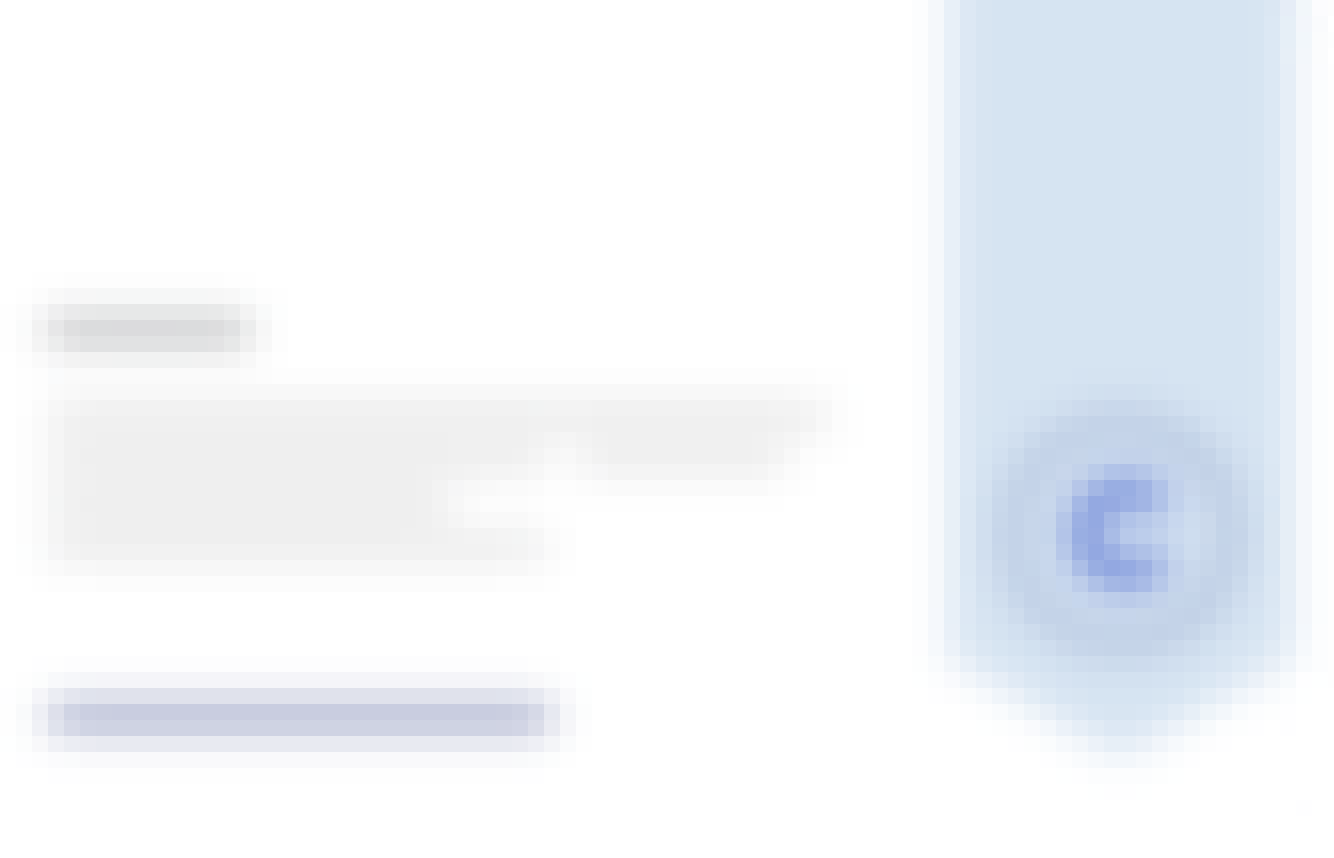
There are 4 modules in this course
Deep learning models are complex and it is difficult to understand their decisions. Explainability methods aim to shed light to the deep learning decisions and enhance trust, avoid mistakes and ensure ethical use of AI. Explanations can be categorised as global, local, model-agnostic and model-specific. Permutation feature importance is a global, model agnostic explainabillity method that provide information with relation to which input variables are more related to the output.
What's included
6 videos8 readings1 assignment1 discussion prompt5 ungraded labs
Local explainability methods provide explanations on how the model reach a specific decision. LIME approximates the model locally with a simpler, interpretable model. SHAP expands on this and it is also designed to address multi-collinearity of the input features. Both LIME and SHAP are local, model-agnostic explanations. On the other hand, CAM is a class-discriminative visualisation techniques, specifically designed to provide local explanations in deep neural networks.
What's included
5 videos7 readings1 assignment1 discussion prompt7 ungraded labs
GRAD-CAM is an extension of CAM, which aims to a broader application of the architecture in deep neural networks. Although, it is one of the most popular methods in explaining deep neural network decisions, it violates key axiomatic properties, such as sensitivity and completeness. Integrated gradients is an axiomatic attribution method that aims to cover this gap.
What's included
4 videos6 readings1 assignment1 discussion prompt7 ungraded labs
Attention in deep neural networks mimics human attention that allocates computational resources to a small range of sensory input in order to process specific information with limited processing power. In this week, we discuss how to incorporate attention in Recurrent Neural Networks and autoencoders. Furthermore, we visualise attention weights in order to provide a form of inherent explanation for the decision making process.
What's included
3 videos3 readings2 assignments1 discussion prompt4 ungraded labs
Instructor

Offered by
Why people choose Coursera for their career




Recommended if you're interested in Data Science
Stanford University
University of California, Davis

Open new doors with Coursera Plus
Unlimited access to 10,000+ world-class courses, hands-on projects, and job-ready certificate programs - all included in your subscription
Advance your career with an online degree
Earn a degree from world-class universities - 100% online
Join over 3,400 global companies that choose Coursera for Business
Upskill your employees to excel in the digital economy