This is the fourth course in the specialization and is aimed at those with basic knowledge of statistics, probability and linear algebra. It will prove to be especially interesting for those with datasets that are being used to make decisions: either business, medical, or technology based.

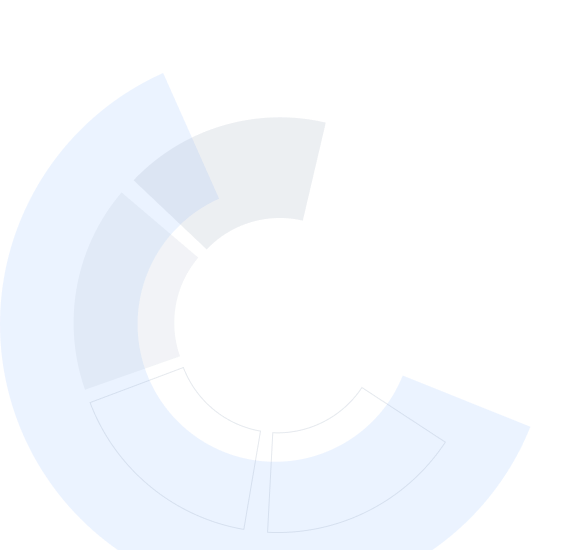

Data Science Decisions in Time: Using Causal Information
Dieser Kurs ist Teil von Spezialisierung Data Science Decisions in Time

Dozent: Thomas Woolf
Empfohlene Erfahrung
Kompetenzen, die Sie erwerben
- Kategorie: causal models
- Kategorie: directed acyclic graphs
- Kategorie: causal forests
- Kategorie: A/B Testing
- Kategorie: structural equations
Wichtige Details

Zu Ihrem LinkedIn-Profil hinzufügen
August 2024
16 Aufgaben
Erfahren Sie, wie Mitarbeiter führender Unternehmen gefragte Kompetenzen erwerben.
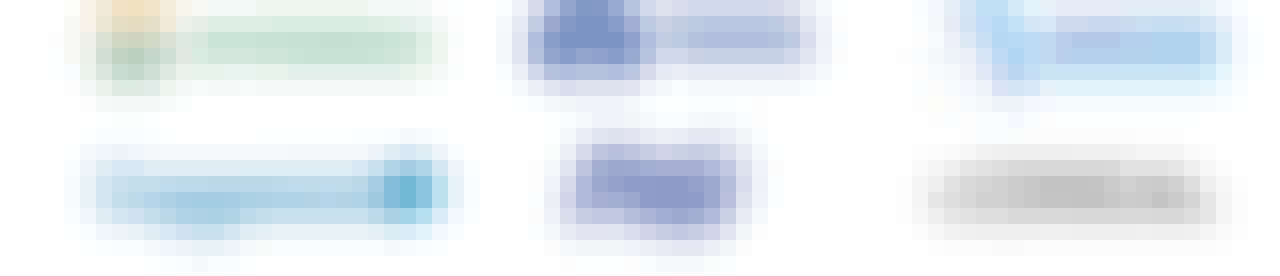
Erweitern Sie Ihre Fachkenntnisse
- Lernen Sie neue Konzepte von Branchenexperten
- Gewinnen Sie ein Grundverständnis bestimmter Themen oder Tools
- Erwerben Sie berufsrelevante Kompetenzen durch praktische Projekte
- Erwerben Sie ein Berufszertifikat zur Vorlage
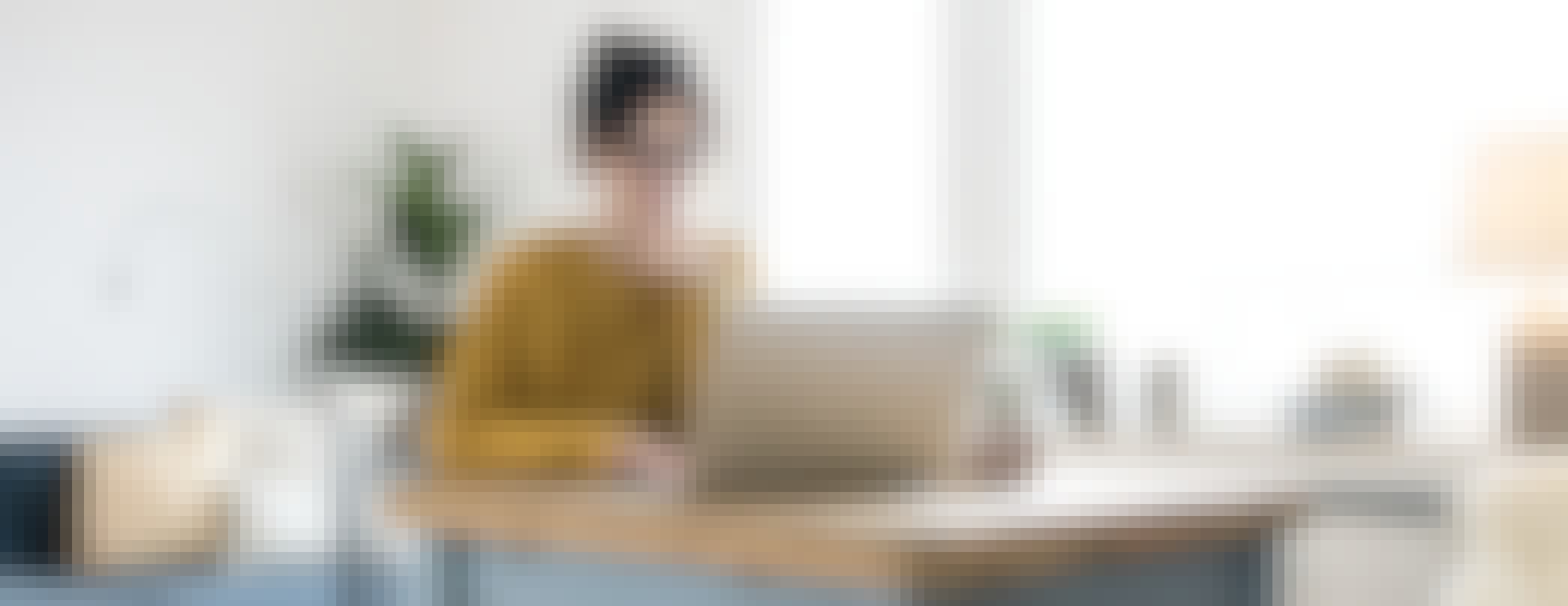
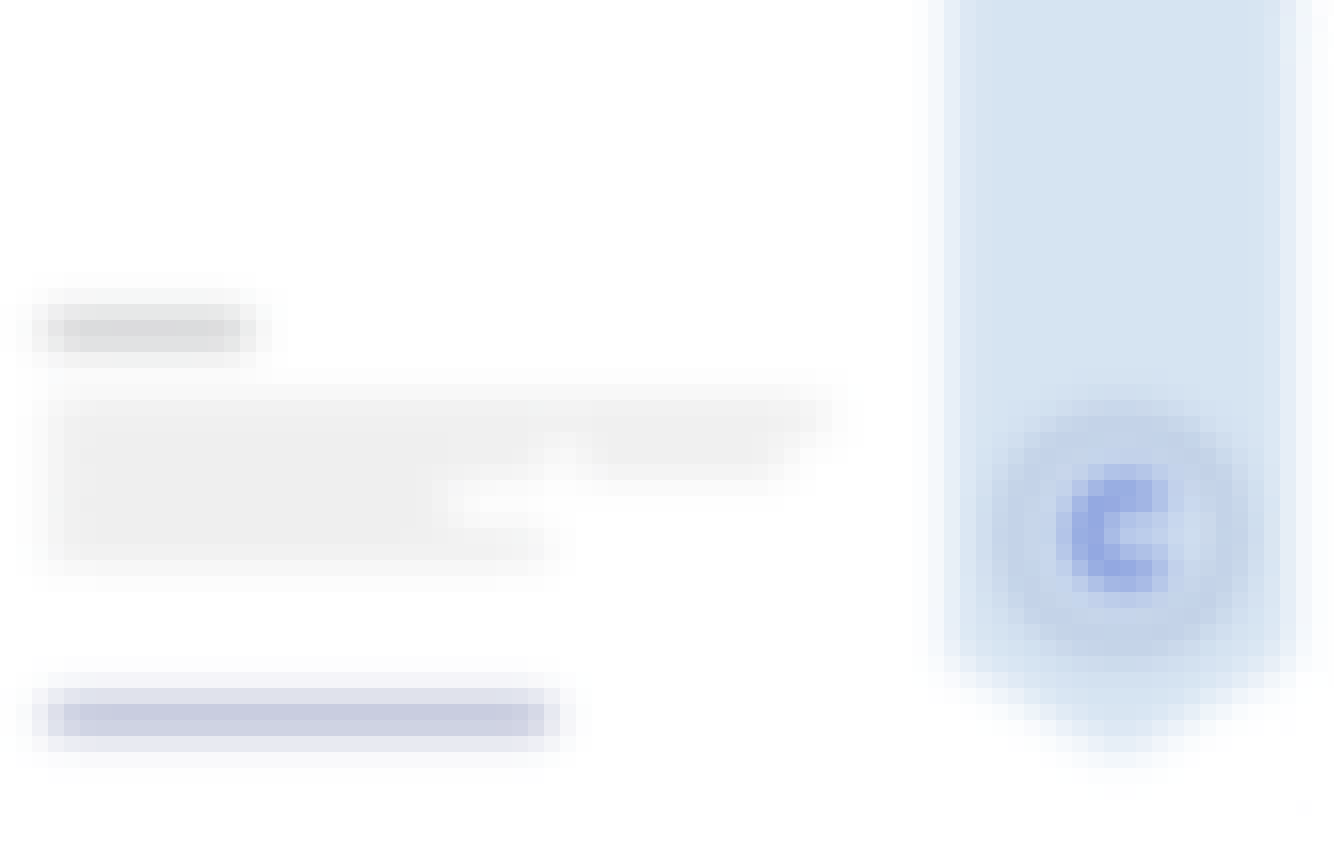
Erwerben Sie ein Karrierezertifikat.
Fügen Sie diese Qualifikation zur Ihrem LinkedIn-Profil oder Ihrem Lebenslauf hinzu.
Teilen Sie es in den sozialen Medien und in Ihrer Leistungsbeurteilung.
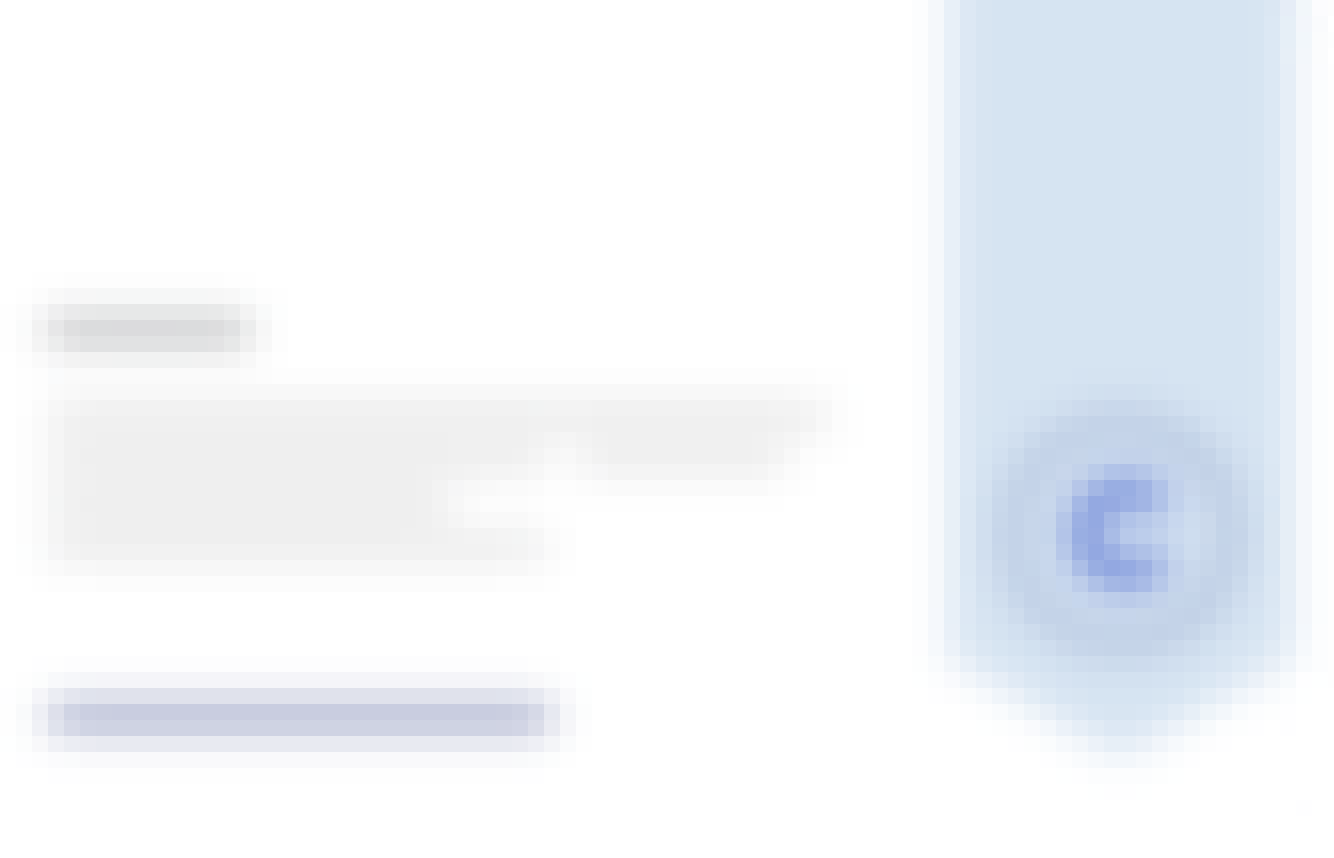
In diesem Kurs gibt es 6 Module
How do we best use information for setting polices, for setting prices, and for deciding what actions to take? We've been exploring this throughout our specialization. In this fourth course, we add in an intriguing route for optimizing decisions: causality. We start with considerations of a causal graphical structure, when it can be cleanly defined, where we have the ability to make decisions, to take actions, and to evaluate overall policies with a clear eye towards how the individual decisions are causally linked to the outcome. This is not always possible, but the ability to think about setting up this type of decision making structure is the end goal of our specialization. It is only possible with sufficient data, and that data does need to have a particular structure, ideally even having been collected with a causal analysis as the end point. In this first week we evaluate how to best update prices for a supermarket, as an example of the type of decision making that may be possible with these methods.
Das ist alles enthalten
3 Videos1 Lektüre3 Aufgaben
Classification and Regression are the lodestars of statistics and they come up again within the context of causal decisions. In this case the regression is really using the data to attempt to determine causal effects. In an analog to classification, when we define the optimal causal decision we are optimizing in a different way on the data than we do within the causal effect. This helps us to decide what we most want to determine from the data and what are the most important goals from the analysis. In this second week we continue our story for optimizing causal decisions by focusing on where best to locate a restaurant based on location data (anonymized) from cell phone user data.
Das ist alles enthalten
3 Videos1 Lektüre3 Aufgaben
Online advertising is both a challenge and an opportunity for businesses. From the marketing perspective, defining how much to spend and on who and when is the question they need answered. From a user perspective, the fewer the ads the better, but if there must be ads, can they be relevant and helpful. This is an example of a marketplace, based on online advertising. A simple example, one that we considered earlier, is A:B testing. In this week we return to this question of how to optimize the presentation of information, but now from a causal perspective. We will go over the causal forest route for how to determine an optimal causal decision. We will also go over randomized clinical trials and how the control of the treatment effect can make the analysis significantly cleaner.
Das ist alles enthalten
3 Videos1 Lektüre3 Aufgaben
David Blei's group contributed an intriguing route for defining causal decisions where confounding is still present, but can be treated in a way that still determines a causal understanding of the decision process. We will explore that paper, and its implications, along with other similar routes for working with data where the confounding issues may otherwise make the analysis a challenge. We open with how best to define the spend on a new movie project: hire that star actor or spend more on stunts? This is another example of a causally linked decision that does not have a final 'right' or 'wrong' but that does have major implications for a business.
Das ist alles enthalten
3 Videos1 Lektüre3 Aufgaben
How best to determine a decision for a large group versus an individual is our final motif for the specialization. In many ways this is the defining question for healthcare: how best to determine an optimal treatment for each individual? While there is a lot of work ahead to describe how this may be done in a detailed setting, there has been progress towards how to use information collected for many individuals that can then be a help in defining the best treatment for an individual. It is this current progress that has many people excited for the future of personalized medicine. At the same time it is a appropriate to realize the limits of what can be done, right now, with the question of an optimal individual treatment.
Das ist alles enthalten
3 Videos1 Lektüre3 Aufgaben
Das ist alles enthalten
1 Aufgabe1 Programmieraufgabe
Dozent

Empfohlen, wenn Sie sich für Data Analysis interessieren
Johns Hopkins University
Coursera Project Network
Warum entscheiden sich Menschen für Coursera für ihre Karriere?





Neue Karrieremöglichkeiten mit Coursera Plus
Unbegrenzter Zugang zu über 7.000 erstklassigen Kursen, praktischen Projekten und Zertifikatsprogrammen, die Sie auf den Beruf vorbereiten – alles in Ihrem Abonnement enthalten
Bringen Sie Ihre Karriere mit einem Online-Abschluss voran.
Erwerben Sie einen Abschluss von erstklassigen Universitäten – 100 % online
Schließen Sie sich mehr als 3.400 Unternehmen in aller Welt an, die sich für Coursera for Business entschieden haben.
Schulen Sie Ihre Mitarbeiter*innen, um sich in der digitalen Wirtschaft zu behaupten.
Häufig gestellte Fragen
Access to lectures and assignments depends on your type of enrollment. If you take a course in audit mode, you will be able to see most course materials for free. To access graded assignments and to earn a Certificate, you will need to purchase the Certificate experience, during or after your audit. If you don't see the audit option:
The course may not offer an audit option. You can try a Free Trial instead, or apply for Financial Aid.
The course may offer 'Full Course, No Certificate' instead. This option lets you see all course materials, submit required assessments, and get a final grade. This also means that you will not be able to purchase a Certificate experience.
When you enroll in the course, you get access to all of the courses in the Specialization, and you earn a certificate when you complete the work. Your electronic Certificate will be added to your Accomplishments page - from there, you can print your Certificate or add it to your LinkedIn profile. If you only want to read and view the course content, you can audit the course for free.
If you subscribed, you get a 7-day free trial during which you can cancel at no penalty. After that, we don’t give refunds, but you can cancel your subscription at any time. See our full refund policy.