Nouvellement mis à jour pour 2024 ! Mathematics for Apprentissage automatique and Data Science est un programme en ligne fondamental créé par DeepLearning.IA et enseigné par Luis Serrano. Dans l'apprentissage automatique, vous appliquez des concepts mathématiques à travers la programmation. Et donc, dans cette spécialisation, vous appliquerez les concepts mathématiques que vous apprenez en utilisant la programmation Python dans des exercices pratiques en laboratoire. En tant qu'apprenant dans ce programme, vous aurez besoin de compétences de base à intermédiaires en programmation Python pour réussir. Après avoir terminé ce cours, vous serez en mesure de : - Représenter des données sous forme de vecteurs et de matrices et identifier leurs propriétés en cours d'utilisation des concepts de singularité, de rang et d'indépendance linéaire, etc.

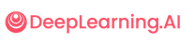
Algèbre linéaire pour l'apprentissage automatique et la science des données
Ce cours fait partie de Spécialisation Mathématiques pour l'apprentissage automatique et la science des données
Enseigné en Anglais
Certains éléments de contenu peuvent ne pas être traduits

Instructeur : Luis Serrano
118 695 déjà inscrits
Cours
(1,544 avis)
94%
Expérience recommandée
Ce que vous apprendrez
Représenter les données sous forme de vecteurs et de matrices et identifier leurs propriétés à l'aide des concepts de singularité, de rang et d'indépendance linéaire
Appliquer les opérations courantes de l'algèbre vectorielle et matricielle telles que le produit point, l'inverse et les déterminants
Exprimer certains types d'opérations matricielles sous forme de transformations linéaires et appliquer les concepts de valeurs propres et de vecteurs propres à des problèmes d'apprentissage automatique
Compétences que vous acquerrez
- Catégorie : Valeurs propres et vecteurs propres
- Catégorie : Équation linéaire
- Catégorie : Déterminants
- Catégorie : Apprentissage automatique
- Catégorie : Algèbre linéaire
Détails à connaître

Ajouter à votre profil LinkedIn
9 quizzes
Cours
(1,544 avis)
94%
Expérience recommandée
Découvrez comment les employés des entreprises prestigieuses maîtrisent des compétences recherchées
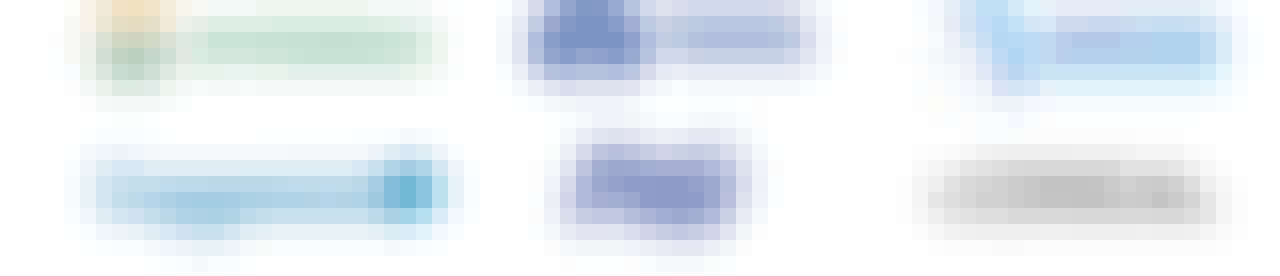
Élaborez votre expertise du sujet
- Apprenez de nouveaux concepts auprès d'experts du secteur
- Acquérez une compréhension de base d'un sujet ou d'un outil
- Développez des compétences professionnelles avec des projets pratiques
- Obtenez un certificat professionnel partageable
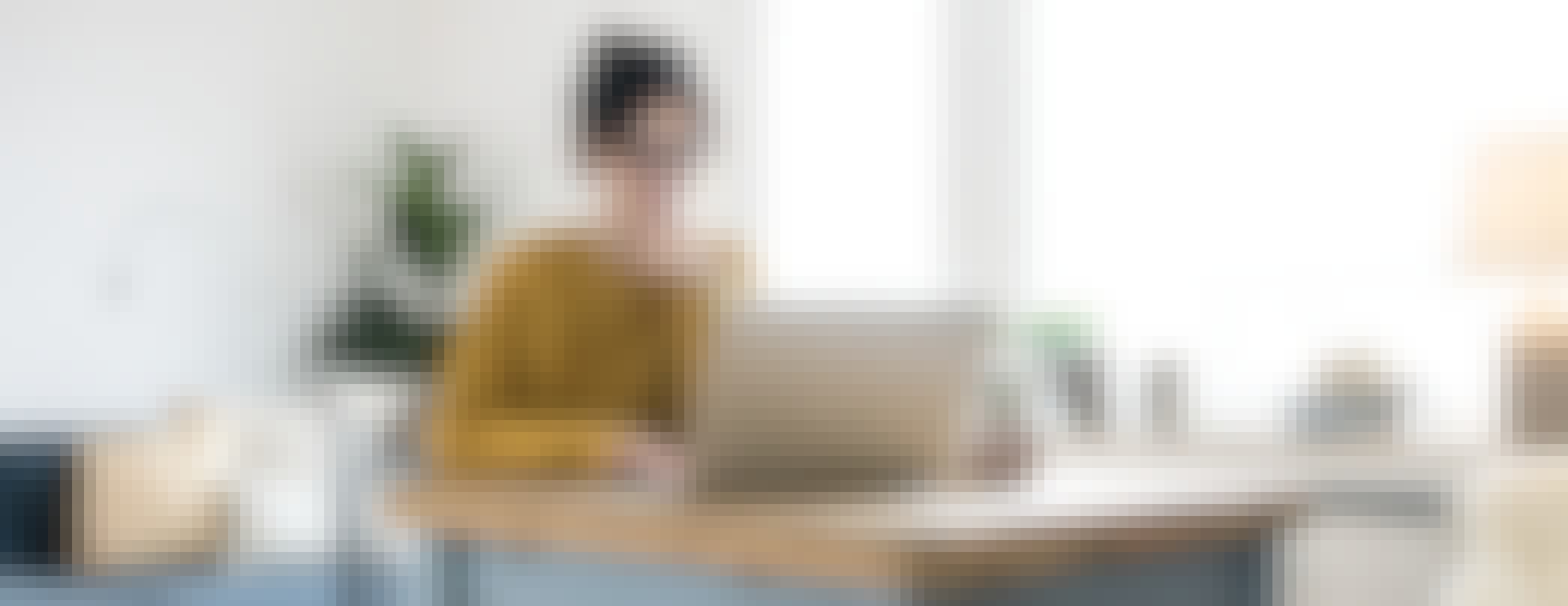
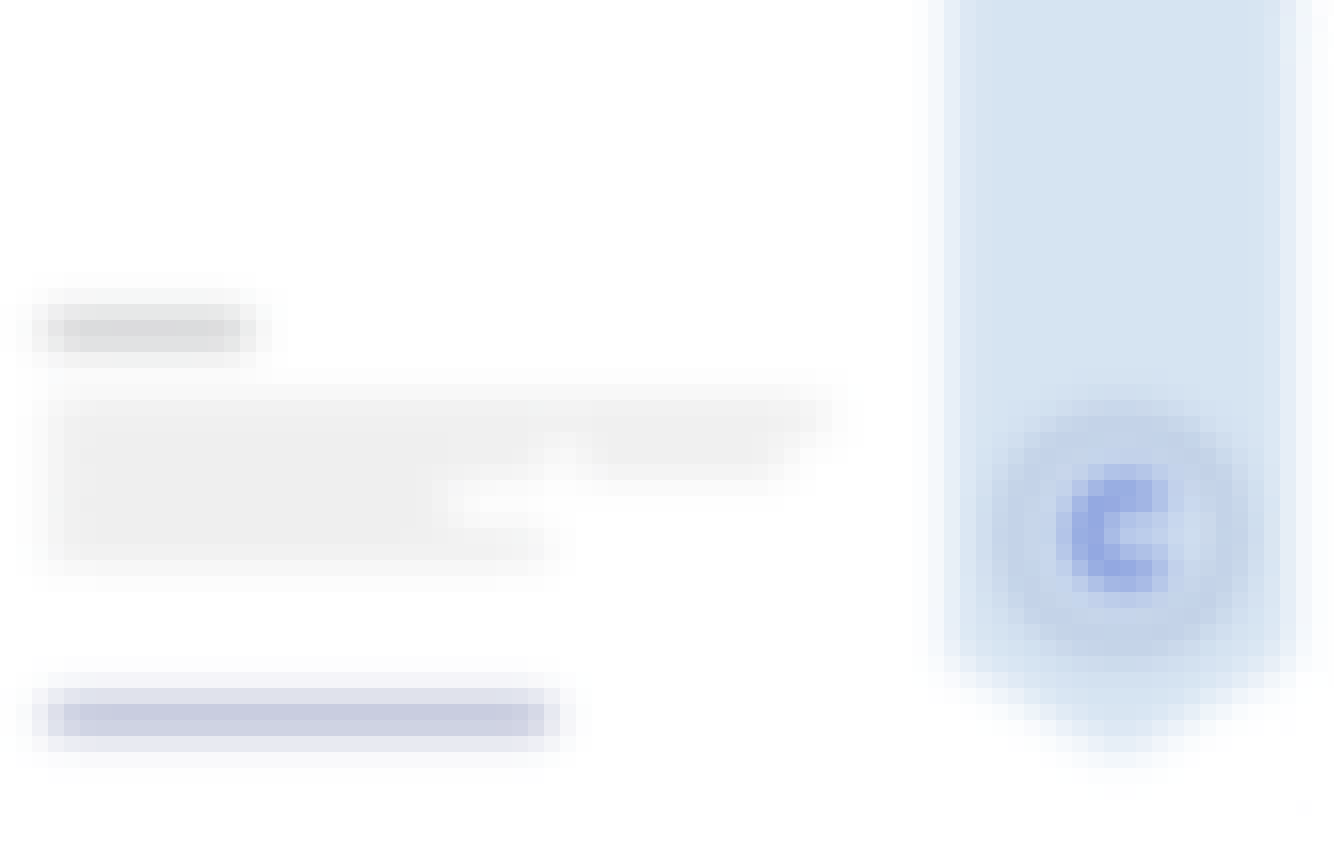
Obtenez un certificat professionnel
Ajoutez cette qualification à votre profil LinkedIn ou à votre CV
Partagez-le sur les réseaux sociaux et dans votre évaluation de performance
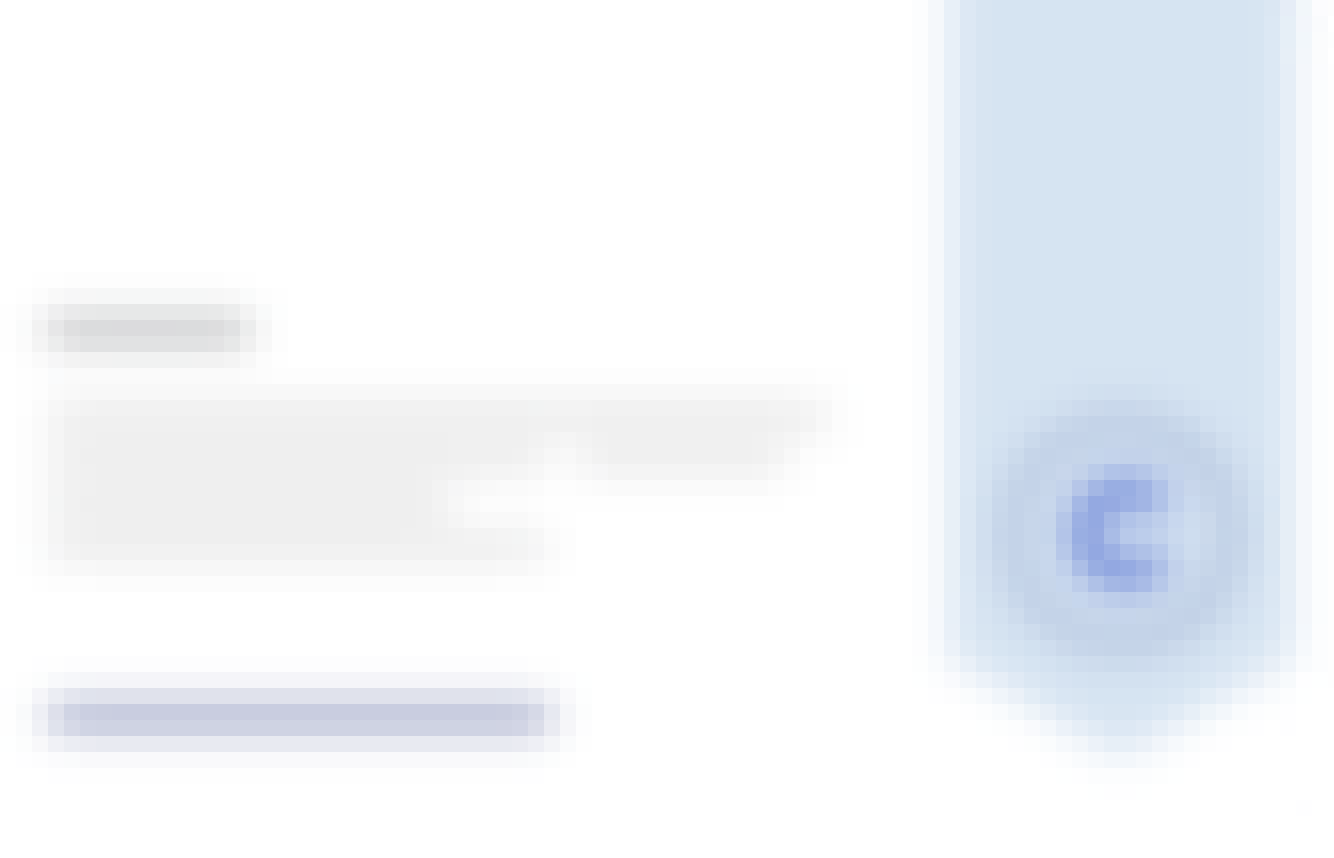
Il y a 4 modules dans ce cours
Les matrices sont couramment utilisées dans l'apprentissage automatique et la science des données pour représenter les données et leurs transformations. Au cours de cette semaine, vous apprendrez comment les matrices découlent naturellement des systèmes d'équations et comment certaines propriétés des matrices peuvent être considérées en termes d'opérations sur les systèmes d'équations.
Inclus
14 vidéos8 lectures3 quizzes1 élément d'application2 laboratoires non notés
Cette semaine, vous apprendrez à résoudre un système d'équations linéaires à l'aide de la méthode d'élimination et de la forme d'échelon de rang. Vous découvrirez également une propriété importante d'une matrice : le rang. Le concept de rang d'une matrice est utile en vision artificielle pour la compression d'images.
Inclus
12 vidéos5 lectures2 quizzes1 devoir de programmation1 laboratoire non noté
Une instance individuelle (observation) de données est généralement représentée sous la forme d'un vecteur dans l'apprentissage automatique. Au cours de cette semaine, vous découvrirez les propriétés et les opérations sur les vecteurs. Vous découvrirez également les transformations linéaires, l'inverse d'une matrice et l'une des opérations les plus importantes sur les matrices : la multiplication matricielle. Vous verrez comment la multiplication matricielle découle naturellement de la composition de transformations linéaires. Enfin, vous apprendrez à appliquer aux réseaux neuronaux certaines des propriétés des matrices et des vecteurs que vous avez apprises jusqu'à présent.
Inclus
14 vidéos3 lectures2 quizzes1 devoir de programmation3 laboratoires non notés
Au cours de cette dernière semaine, vous examinerez plus en détail les déterminants. Vous apprendrez comment les déterminants peuvent être interprétés géométriquement comme une surface et comment calculer le déterminant du produit et de l'inverse des matrices. Nous terminerons ce cours avec les valeurs propres et les vecteurs propres. Les vecteurs propres sont utilisés pour la réduction de la dimensionnalité dans l'apprentissage automatique. Vous verrez comment les vecteurs propres découlent naturellement du concept de bases propres.
Inclus
20 vidéos7 lectures2 quizzes1 devoir de programmation1 laboratoire non noté
Instructeur

Offert par
Recommandé si vous êtes intéressé(e) par Algorithmes
Imperial College London
Pour quelles raisons les étudiants sur Coursera nous choisissent-ils pour leur carrière ?




Avis des étudiants
Affichage de 3 sur 1544
1 544 avis
- 5 stars
70,95 %
- 4 stars
19,34 %
- 3 stars
4,72 %
- 2 stars
2,39 %
- 1 star
2,58 %

Ouvrez de nouvelles portes avec Coursera Plus
Accès illimité à plus de 7 000 cours de renommée internationale, à des projets pratiques et à des programmes de certificats reconnus sur le marché du travail, tous inclus dans votre abonnement
Faites progresser votre carrière avec un diplôme en ligne
Obtenez un diplôme auprès d’universités de renommée mondiale - 100 % en ligne
Rejoignez plus de 3 400 entreprises mondiales qui ont choisi Coursera pour les affaires
Améliorez les compétences de vos employés pour exceller dans l’économie numérique
Foire Aux Questions
Il s'agit d'un cours adapté aux débutants, visant à enseigner les concepts couverts avec un minimum de connaissances de base nécessaires. Si vous êtes familier avec les concepts de l'algèbre linéaire, vous trouverez que ce cours est une bonne révision pour le prochain cours de la spécialisation, Calculus for Machine Learning and Data Science.
Oui ! Nous voulons faire tomber les barrières qui empêchent les gens de progresser en mathématiques. Dans ce cours, nous inversons la pédagogie traditionnelle de l'enseignement des mathématiques, en commençant par les cas d'utilisation dans le monde réel et en revenant à la théorie.
La plupart des gens qui sont bons en maths ont simplement plus de pratique des maths et, par conséquent, plus d'aisance avec l'état d'esprit nécessaire pour réussir. Ce cours est l'endroit idéal pour commencer ou perfectionner ces compétences fondamentales, et construire l'état d'esprit nécessaire pour être bon en maths.
Algèbre linéaire (matrices, vecteurs et leurs applications)